Cognitive factors in predicting continued use of information systems with technology adoption models
Chi-Cheng Huang
Introduction.The ultimate viability of an information system is dependent on individuals’ continued use of the information system. In this study, we use the technology acceptance model and the theory of interpersonal behaviour to predict continued use of information systems.
Method.We established a Web questionnaire on the mysurvey Website and recruited participants via social networks. Data were collected from a sample of 1154 Webmail users in Taiwan.
Analysis. Our models are analysed using the partial least squares structural equation modelling method.
Results. The results of this study indicate that (1) the theory of interpersonal behaviour has better ability to predict continued use than the technology acceptance model; (2) the technology acceptance model has a better ability to predict intention to continue use than the theory of interpersonal behaviour; (3) the combination between the technology acceptance model and the theory of interpersonal behaviour is properly able to explain continued use of information systems; (4) multiple group analysis indicates that two relations in the combined model differ significantly between light experience users and heavy experience users.
Conclusions. The technology acceptance model and the theory of interpersonal behaviour should not be viewed as alternative but as supplementary models. Moreover, the integration of such two models can properly predict continued use of information systems.
Introduction
Information system researchers have long sought to determine why different individuals choose to use specific information systems (e.g.,Davis, 1989; Delone and McLean, 2003; Venkatesh, Morris, Davis, and Davis, 2003). Although understanding the initial adoption of an information system is important for information system success, the ultimate viability of an information system is dependent on individuals’ continued use of the information system (Karahanna, Straub and Chervany 1999). The development of models for explaining and predicting information system continued use has become an important issue of information system research. Although the use of well-developed models such as the technology acceptance model, the theory of planned behaviour, the expectation–confirmation model, or task-technology fit may give explanations about the determinants of continued information system use, researchers need to consider the question of which model should be used. We empirically compare the following two technology adoption models: the technology acceptance model and the theory of interpersonal behaviour (Triandis, 1980). The two models were selected because they relate to an issue that has been extensively discussed in information system research in a controversial manner: is the enactment of continued information system use mainly under conscious control (the technology acceptance model) or is it activated in a more automatic, habitualised fashion (the theory of interpersonal behaviour)? The theory of interpersonal behaviour was proposed by Triandis (1980) and has been applied to explain behaviour in social psychology, and posits that social factors, affect and perceived consequences influence intention, which in turn influences behaviour. It also posits that habit and facilitating conditions affect behaviour. Although previous information system studies (Chang and Cheung, 2001; Gagnon et al., 2003; Thompson, Higgins and Howell 1991, 1994) have used the theory to explain computer or Internet use, until now, it has rarely been applied in predicting continued information system use.
Numerous empirical studies have found that the technology acceptance model consistently explains a substantial proportion of the variance (typically about 40%) in usage intention and behaviour (Venkatesh and Davis, 2000). Such findings imply that 60% of the variance in intention and behaviour remains unexplained. According to the model, technology use is determined by conscious control. However, with repetition of the same use, it may be determined not by conscious control but by automaticity. The popular meaning of automaticity is something that happens, no matter what, as long as certain conditions are met (Bargh and Chartrand, 1999). Social behaviour is often triggered automatically on the mere presence of relevant situational features; this behaviour is unmediated by conscious perceptual or judgmental processes (Bargh, Chen and Burrows 1996). If an individual consistently behaves the same way in response to a situation, that response should become automatically associated with those situational features. Contemporary information system research suggests that the technology acceptance model may fall short of providing an accurate account of automatic use because such use is driven mainly by habit rather than by conscious judgment (Aarts and Dijksterhuis, 2000; Verplanken, Aarts, van Knippenberg and Moonen, 1998). Triandis (1980) indicated that habit is a repeated behavioural pattern that occurs automatically, outside conscious awareness. Preconscious activation of mental representations (i.e., habit) develops from their frequent and consistent activation in the presence of a given stimulus event in the environment (Bargh, 1989; Bargh and Chartrand, 1999). Kim and Malhotra (2005) indicated that system usage is driven by conscious intention when the linkage between stimuli and action is not fully developed. Once information system use becomes routine (performed frequently in a stable environment), habit is likely to be a reliable predictor of actual use. From these perspectives, we see important reasons for model integration: increasing empirical evidence suggesting that the technology acceptance model has the need to integrate with other theories for improvement of its prediction abilities, and the fact that the theory of interpersonal behaviour demonstrates a new perspective in exploring information system use.
According to our previous discussion, selecting a suitable model to predict continued use of information systems should be a significant problem for information system researchers. Although previous studies have added independent variables such as trust, information technology self-efficacy, habit, satisfaction, perceived enjoyment, facilitating conditions, information quality, system quality, and social influence into the technology acceptance model for predicting continued use (Alwahaishi and Snásel, 2013; Bhattacherjee, Perols and Sanford, 2008; Gefen, 2003; Hu, Clark and Ma, 2003; Hong, Thong and Tam, 2006; Mäntymäki and Salo, 2011; Roca, Chiub and Martinez 2006; Saeed and Abdinnour-Helm, 2008; Sun and Jeyaraj, 2013; Venkatesh, Thong, Chan, Hu and Brown 2011), Benbasat and Barki (2007) indicated that there is no commonly accepted adoption model in the information systems field. A suitable model should reflect the characteristics of continued use of information systems. According to the information systems literature, there are three approaches to reflect such characteristics: (1) continued use of information systems is a rational decision process involving cognitive evaluations and conscious intentions (e.g., de Guinea and Markus, 2009; Karahanna, Straub and Chervany 1999; Kim and Malhotra, 2005; Venkatesh, Speier and Morris, 2002); (2) affective responses are inputs that elicit continued use of information systems (e.g.,Bhattacherjee, 2001; Bhattacherjee et al., 2008; de Guinea and Markus, 2009; Kim, Chan and Chan, 2007; Thong, Hong and Tam, 2006) and (3) continued use of information systems is an automatic or habitual behaviour pattern (Cheung and Limayem, 2005; de Guinea and Markus, 2009; Limayem and Cheung, 2008, 2011; Limayem, Hirt and Cheung, 2007).
These approaches are consistent with the suggestions of de Guinea and Markus (2009), indicating that intention, emotion and habit are three determinants to shape continued use. The technology acceptance model describes information system use as fundamentally intentional behaviour and the theory of interpersonal behaviour regards affect and habit as inputs to the decision process of information system use. Thus, combining the technology acceptance model with the theory of interpersonal behaviour to formulate a predictive model to consider intention, affect and habit simultaneously may provide better understanding of the continued use of information systems. This study may provide a bridge to link the three approaches of rational decision process, affect and habitual behaviour for predicting continued use of information systems. Thus, the main purpose of this study is to integrate the technology acceptance model and the theory of interpersonal behaviour and see whether such integration can explain continued use fully. Based on our integrated model, three research objectives arise:
- Compare predictive power between the technology acceptance model and the theory of interpersonal behaviour;
- Examine the appropriateness of model integration between the technology acceptance model and the theory of interpersonal behaviour;
- Analyse causal relationships among variables in the integrated model and thereby explore the importance of these relationships in predicting continued use of information systems.
Information science and information system adoption: a review
Information science is a discipline that investigates the properties and behaviour of information, the forces governing the flow of information and the means of processing information (Borko, 1968). Hawkins (2001) also indicated that information science draws on important concepts from a number of related disciplines such as behavioural science, communications, management and computing technology to become a whole focusing on information. Within such related disciplines, behavioural science involves psychology and human-computer interaction. From the perspective of behavioural science, researchers within the information science field may examine the use of information in organizations, along with the interaction between people, organizations, and any existing information systems (Hawkins, 2001). Thus, behavioural studies of users with respect to information systems may help information science researchers to understand what factors determine the use of information systems. As a result, organizations may develop strategies to encourage employees to use information systems and, thereby, information within organizations may be transmitted and shared effectively through information systems use.
To improve organizational productivity, a number of organizations have begun to invest in information systems. Some estimates reported that since the 1980s, about 50% of all new capital investment in organizations relates to information systems (Venkatesh et al., 2003). After introducing information systems into organizations, these systems need to be adopted and used continuously by employees. Information system researchers made substantial efforts to understand what factors affect users’ beliefs toward information system adoption or continued use. A number of theoretical perspectives or models have been used to provide an understanding of the determinants of information system adoption. In the past two decades of information system use research, the main focus has been on cognitive behavioural models (Limayem and Hirt, 2003), which include the theory of reasoned action (Fishbein and Ajzen, 1975), the theory of planned behaviour (Ajzen, 1991), the technology acceptance model (Davis, 1989; Davis et al., 1989), social cognitive theory (Compeau and Higgins, 1995), the theory of interpersonal behaviour (Triandis, 1980), and the unified theory of acceptance and use of technology (Venkatesh et al., 2003). However, these models have some limitations (Sun and Zhang, 2006). The first limitation concerns their explanatory power, in that most of the models report explaining less than 60% of variance. This implies that additional factors may need to be taken into account. The second limitation is the inconsistent relationships among factors in these models, which implies that the generalisability of these models may vary across different contexts. To refine these behavioural models, a number of researchers have incorporated additional factors from different contexts into these models (Benbasat and Barki, 2007) or combined different behavioural models (e.g., Dishaw and Strong, 1999; Lu, Zhou and Wang 2009; Taylor and Todd, 1995) to form an integrated model. These efforts aim to further explain the relationships among users’ beliefs about information system use and to improve the explained variance of information system use.
Among the behavioural models, the technology acceptance model is a popular information system model: this is an adapted version of the theory of reasoned action (Fishbein and Ajzen, 1975), specifically developed to explain user adoption of a technology (Davis et al., 1989). A theory of the development and refinement of technology acceptance by Davis (1989) was a major step in information system use research as he examined perceived ease of use and perceived usefulness to understand information system use. The technology acceptance model and its variants (Taylor and Todd, 1995; Venkatesh and Davis, 2000; Venkatesh et al., 2003; Venkatesh, Thong and Xu, 2012) have been used in explaining information system use. The technology acceptance model is generally regarded as the most influential and commonly employed theory for describing the adoption of information systems (Lee, Kozar and Larsen, 2003). The first two technology acceptance model articles written by Davis (1989) and Davis, Bagozzi and Warshaw (1989) have received 27,469 and 14,658 journal citations, respectively, according to Google Scholar, as of 2016. The technology acceptance model has been applied in information systems (e.g., word processors, spreadsheets, groupware, e-mail, decision support systems, enterprise resource planning, World Wide Web, online trading systems), technologies (e.g., the Internet, personal computers, tablet computers, global positioning systems, cellular phones, telemedicine technology, interactive TV), or Internet services (e.g., cloud computing, Internet banking, Webmail, online shopping, mobile data services, multimedia message services, e-government services).
Although the literature has widely examined information system adoption using the technology acceptance model, continued information system use has become the subject of theoretical developments and empirical examinations. Previous literature (e.g., Lee et al., 2003; Venkatesh and Davis, 2000) indicated that low percentages of variance explained were considered as a major problem of technology acceptance model studies. In addition, de Guinea and Markus (2009) suggested that conscious intentions, emotion and habit are three important pillars of continued information system use. From these perspectives, the technology acceptance model must be adjusted to fit in the context of continued information system use. Because Triandis’s (1980) theory of interpersonal behaviour accounts for habit, intention and affect, we integrate this theory into the technology acceptance model as a model adjustment for predicting continued information system use.
Research method
The motivation for choosing Webmail
In this study, we chose Webmail as a research target. Webmail is a Web-based e-mail service that allows people to process e-mail by using a Web browser instead of an e-mail client such as Microsoft Outlook. From this perspective, Webmail may be considered as a kind of cloud-based service. Cloud computing is a current technology trend for developing the next generation application architecture (Hutchinson, Ward and Castilon, 2009). Cloud computing refers to both the applications delivered as services over the Internet and the hardware and software systems within the data centres which provide those services (Armbrust et al., 2010). Behrend, Wiebe, London and Johnson (2011) mention that cloud applications exist online and are available to users through the Internet, rather than being installed on particular users’ local computers. Users can receive and write e-mail from anyone else’s computer with a Web browser connected to the Internet. Although Webmail is a cloud-based service, it is built and supported by hardware and software systems within the e-mail centres, allowing users to deliver and communicate information more effectively. Thus, cloud services have been considered as a research area in the context of information system. Information system researchers (e.g., Behrend et al., 2011; Opitz, Langkau, Schmidt and Kolbe, 2012; Park and Kim, 2014; Stantchev, Colomo-Palacios, Soto-Acosta and Misra, 2014) have used the technology acceptance model to examine cloud service adoption.
We believe that individuals are likely to check Webmail habitually. According to three annual investigations of the Taiwan Network Information Centre between 2010 and 2012, individuals most frequently used the Internet to search for information, check Webmail or visit social networking sites (TWNIC, 2013). Thus, checking Webmail may have become a routinised action when individuals surf the Internet. With repeated performances, a situational cue automatically activates the behaviour without any conscious effort (Kim, 2009). An individual will, without involving awareness or intentions, react immediately to the context of surfing the Internet (a situational cue) by checking Webmail (behaviour) because he or she may rely on Webmail to transmit files and share information in everyday life. Thus, checking Webmail may be regarded as an automatic behavioural pattern or a habit.
Although Webmail is not a new technological innovation and it has already reached a high level of spread, we think that it is still worth it to understand continued use of Webmail for a number of reasons. First, unlike technology such as Voice Over Internet Protocol or Internet television, Webmail is easy to learn and is popular among various groups including teenagers or elders. Such popular technology may help us to uncover the continued use of various groups. Second, retaining current users is important for a relatively mature technology, such as Webmail, because 5% of current customers will create between 25% and 85% of an organization’s profit (Reichheld and Sasser, 1990). Third, information systems literature (e.g., Kim, 2009; Kim and Malhotra, 2005) indicated that habit (e.g., the behaviour-behaviour relationship) and feedback (e.g., the behaviour-evaluation relationship) play important roles in affecting continued information system use. Checking Webmail is one of the three most popular activities on the Internet in Taiwan, suggesting that in everyday life a large number of individuals routinely visit Webmail sites. Thus, Webmail is a suitable technology to analyse the mechanisms of habit and feedback.
The arguments for using the technology acceptance model and the theory of interpersonal behaviour
In the past two decades of information system use research, the main focus has been on cognitive behavioural models (Limayem and Hirt, 2003). Among these models, the technology acceptance model is an information system-specific model. The technology acceptance model regards information system use as a rational, deliberate and cognitive decision-making behaviour. According to the technology acceptance model, a technology use is determined by conscious intention. However, this assumption may not be applicable to continued use of information systems because it ignores that frequently performed behaviour tends to become habitual, and thus automatic, over time (Ouelette and Wood, 1998). With repetition of the same technology use, the technology use may be determined not by conscious intention but by habit. To understand continued use of information systems, researchers should consider habit as a determinant in their information system models. Limayem and Hirt (2003) suggested that a model used to predict continued information system use should include: (1) the intentional and automatic factors of behaviour; (2) factors in properties of the social context and (3) factors reflecting limited control over behaviour. In addition, the suggestions of de Guinea and Markus (2009) indicated that conscious intention, affect and habit are three determinants that shape continued use. Thus, the theory of interpersonal behaviour may be a suitable theory to be integrated into the technology acceptance model for predicting continued Webmail use because it considers the determinants (e.g., affect, habit, social factors, facilitating conditions) as suggested by Limayem and Hirt (2003) and de Guinea and Markus (2009).
Research framework and hypotheses
We collected responses from actual Webmail users. In addition, we also considered sex, age and user experience as three control variables. We selected these particular variables because of their potential impact on information system use as suggested by the existing literature. Ajzen (2002) suggested that measures of attitude should include affective and cognitive components. To understand the impact of different components of attitude on continued Webmail use, we separated attitude into affective and cognitive components. Moreover, we used perceived usefulness as a surrogate for 'perceived consequences' to measure a person’s perceived value of using Webmail. Triandis (1980) used an objective measure to evaluate facilitating conditions. Following the suggestions of Limayem and Hirt (2003), we considered a person’s associated perceptions towards facilitating conditions. Substituting perceived facilitating conditions for objective facilitating conditions is in line with the theory of planned behaviour. We tested the model by administering a Web survey.
In addition to the original causal links in the technology acceptance model and the theory of interpersonal behaviour, we explored three valuable viewpoints in the integrated model. First, because Ajzen’s (1991) theory supported the relation between facilitating conditions and intention and Venkatesh and Davis’s (2000) theory supported the relation between social factors and perceived usefulness, we added two hypothesized paths (facilitating conditions—intention to continue use and social factors—perceived usefulness) into the integrated model. Second, the role of habit in predicting behaviour has been widely examined in information system use and social-psychological behaviour. Intuitively, the more behaviour is performed out of habit, the less cognitive planning (intention) it may involve (de Guinea and Markus, 2009). From this perspective, therefore, we considered whether the impact of intention to continue use on actual use may be weakened when an individual’s habit is developed. Third, as users get into the habit of using a system, learning effects will kick in and may increase the perceived usefulness and the perceived ease of use. That is, users generally perceive a system easier to use and more useful as they acquire more knowledge and confidence through gained experience in using a targeted system. These effects will also heighten affect and cognitive attitude toward system use. From this perspective, we therefore consider whether the impacts of perceived usefulness and the perceived ease of use on attitudes (affect and cognitive attitude) may be heightened when an individual’s habit is developed. Thus, we added the moderating effects of habit (habit × intention to continue use, habit × perceived ease of use, and habit × perceived usefulness) into the integrated model. Figure 1 describes our research model.
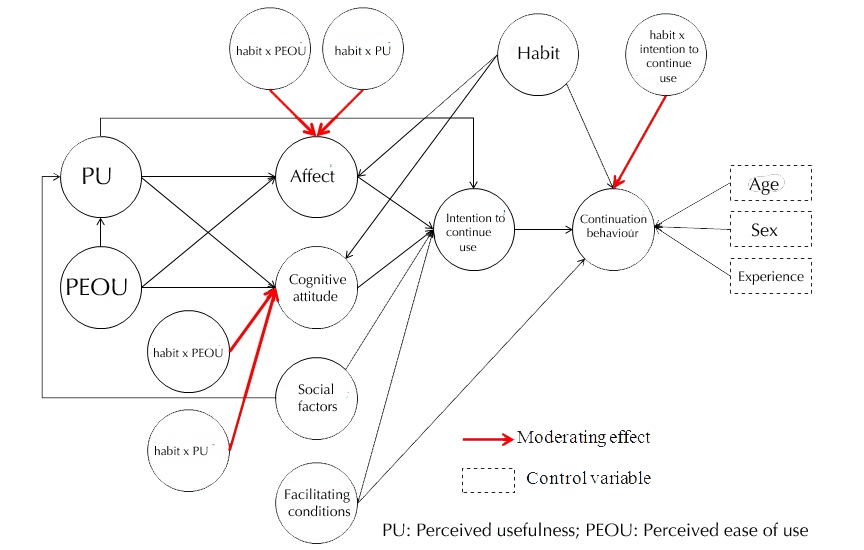
Figure 1: Grade levels of the participants [Click for larger image]
According to Figure 1 and our discussion in the literature review, we also develop several research hypotheses as follows:
H1: Habit, facilitating conditions and intention to continue use are positively associated with actual behaviour.
H2: Affective attitude, cognitive attitude, social factors, facilitating conditions and perceived usefulness are positively associated with intention to continue use.
H3: Habit, perceived ease of use and perceived usefulness are positively associated with affective attitude.
H4: Habit, perceived ease of use and perceived usefulness are positively associated with cognitive attitude.
H5: Social factors and perceived ease of use are positively associated with perceived usefulness.
H6: Habit negatively moderates the relationship between intention to continue use and actual behaviour.
H7a: Habit positively moderates the relationship between perceived ease of use and affective attitude.
H7b: Habit positively moderates the relationship between perceived usefulness and affective attitude.
H7c: Habit positively moderates the relationship between perceived ease of use and cognitive attitude.
H7d: Habit positively moderates the relationship between perceived usefulness and cognitive attitude.
Measurement development
The survey measures for this study were derived from previously published studies: the technology acceptance model constructs (perceived ease of use, perceived usefulness) questions were adapted from Davis et al. (1989), intention to continue use and continued-use questions were adapted from Limayem et al. (2007), affective and cognitive attitude questions were adapted from Davis (1993) and Taylor and Todd (1995), social factors questions were adapted from Pahnila and Warsta (2010) and facilitating conditions questions and habit questions were adapted from Limayem and Hirt (2003). Except for facilitating conditions, the eight other constructs were measured with reflective indicators. Facilitating conditions were measured with formative indicators because the indicators were conceptually distinct. All of the constructs were measured using seven-point Likert scales ranging from strongly disagree (1) to strongly agree (7).
Survey administration
We established a Web questionnaire on the mySurvey Website. MySurvey provides a mechanism to identify the individual Internet protocol of Internet users. Thus, we can check the Internet protocol and avoid redundancy in completing the Web questionnaire. It was very difficult to access all of the Webmail users in Taiwan because no comprehensive list of Webmail users was available. As a result, it may be impossible to establish a sampling frame because we did not know about the characteristics of Webmail users. The nature of the Internet may prevent random sampling for electronic surveys (Kehoe and Pitkow, 1997). Thus, we decided to adopt a non-probabilistic method to recruit participators. We recruited participators through social networks. That is, we employed an individual’s social network to access others. The perspective of an individual’s social network sampling is similar to the perspective of Breadth-First-Search sampling (Gjoka et al., 2009; Leskovec and Faloutsos, 2006). Breadth-First-Search sampling is widely used for sampling large networks. Breadth-First-Search sampling starts from a seed and explores all neighbours’ nodes. This process continues iteratively. Similarly, we invited our friends to complete the questionnaire and asked them to forward this invitation to their friends. This sampling process continues iteratively. We described our sampling process in detail as follows. We initially examined our contact list in our Gmail accounts to identify how many friends have Webmail accounts. A total of 291 friends were identified. We created a Facebook group, and the group’s page contained descriptions regarding contact information, importance about our study and something about our credentials. Also, we posted a hyperlink to the Web questionnaire. Subsequently, we contacted the 291 friends by e-mail and invited them to join the Facebook group. Also, we asked these friends to complete the Web questionnaire. The invitation also asked them to forward or cross post the e-mail to their friends with Webmail accounts, who, in turn, forwarded it to their friends. However, such cross-posting may be perceived as rude behaviour or spam and individuals who received the e-mail invitation could have rejected our survey. To avoid such perceptions, we included a brief description of the purpose of this study in the e-mail invitation. The description may have helped to increase the credibility of our survey.
We used Facebook to announce our survey message. Social network sites such as Facebook, Twitter, MySpace, etc., have flourished in recent years (Ku et al., 2013; Praveena and Thomas, 2014). Social network sites offer a platform for individuals to interact with one another and manage their friendships (Kim, 2011; Ku, Chen and Zhang, 2013). According to an investigation of use of Facebook in Taiwan, Facebook (88%) has become the most used social network site (TNS, 2014). Also, this investigation indicated that one in three individuals had used Facebook to share information or recommendations from others. Although Facebook was initially used as a communication tool to connect users, Praveena and Thomas (2014) pointed out that Facebook has now grown into a platform for varied discussions even concerning social issues. Thus, it seemed feasible to use Facebook to announce our survey message .
Because we used the viewpoint of social networks to select the sample, our survey may be likely to encounter self-selection bias. By way of cross-posting, our sample may include only our friends or friends’ friends. Thus, we did not reach individuals who were not included in our or friends’ social networks, thereby leading to self-selection bias. Such bias may be a threat to the generalization of our findings and we should interpret our findings with caution. In addition, we may have included only individuals who were willing to join our Facebook group and who completed the Web questionnaire following the e-mail invitation. Individuals who rejected the e-mail invitation did not join the Facebook group to complete the Web questionnaire. Wright (2005) indicated that there is a tendency of some individuals to respond to an invitation to participate in an online survey, while others ignore it, leading to non-response bias. To increase response rate, we offered all participators a gift certificate that was awarded in a random drawing as a survey participation incentive. The information regarding the gift certificate was included in our invitation e-mail and posted on the Facebook group.
The final sample consisted of 1154 participants. In terms of sex, 52% of the participants were male. In terms of current residence, 43.5% are in southern Taiwan, 37.5% are in northern Taiwan, 16.3% are in central Taiwan, and 1.6% are in eastern Taiwan. In terms of educational background, 57.6% are in university, 24.8% are in graduate school, 11.7% are in high school, and 5.9% are in college. In terms of use experience, the distribution is as follows: between five and ten years (42.4%), more than ten years (30.8%), between one and five years (22.5%), and less than one year (4.2%). In terms of e-mail account, the distribution is as follows: Yahoo mail (41.8%), Hotmail (29%), Gmail (26.6%), and others (2.6%).
According to a survey on Lifewire (Tschabitscher, 2017), Zoho Mail was ranked as the most used Webmail in the U.S.A., Yandex Mail ranked second, Hotmail (renamed Outlook) ranked third, Gmail ranked fourth and Yahoo Mail ranked seventh. The survey by ArabCrunch (Saqer, 2009) reported that Yahoo Mail is the most popular Webmail in Jordan, followed by Hotmail. The survey by ComScore (2012) revealed that Hotmail ranked as the top Webmail in Europe, followed by Gmail, Yahoo Mail, Mail.Ru Mail and Yandex Mail. Also, ComScore (2009) reported that Yahoo Mail is the most popular Webmail in Japan, followed by Hotmail, Gmail, Goo Mail and Nifty Mail. These surveys reported Webmail use in different geopolitical regions; however, they did not emphasise which factors influence Webmail use. Although previous studies (e.g., Burton-Jones and Hubona, 2005, 2006; Davis, 1993; Gefen and Straub, 1997) have examined factors influencing e-mail use, these studies focused on the context of organizations. Thus, the present study may broaden our understanding of the factors affecting Webmail use from the viewpoint of geopolitical region.
Partial least squares structural equation modelling
Partial least squares structural equation modelling uses an ordinary least squares regression-based method with the objective of explaining latent constructs’ variance by minimizing the error terms and maximizing the R2 value (Hair et al., 2011, 2014). Henseler, Ringle and Sinkovics (2009) indicated that a partial least squares structural equation model contains two types of linear equations: the measurement (also labelled outer model) and the structural (also labelled inner model). The structural model describes the relationships between latent variables, and the measurement model describes the relationships between a latent variable and its manifest variables. We used Smart partial least squares software (Ringle, Wende and Will, 2005) to assess the theoretical model and hypothesised relationships in this study.
Related research
The technology acceptance model
The original technology acceptance model assesses the impact of four internal factors on the actual use of information system (Davis et al., 1989). The internal factors are perceived ease of use, perceived usefulness, attitude toward use and behavioural intention to use. Perceived usefulness is defined as the extent to which a person believes that using a particular system will enhance his or her job performance. Perceived ease of use is defined as the extent to which a person believes that using a particular system will be free of effort (Davis, 1989). Attitude toward use is defined as an individual’s evaluation about performing behaviour (Taylor and Todd, 1995). Behavioural intention to use indicates to what extent people want to perform behaviour and how much effort they are prepared to exert to perform it (Ajzen, 1991). The original technology acceptance model theorizes that an individual’s behavioural intention is the most important predictor for using an information system, which in turn is determined by attitude toward use and perceived usefulness. In addition, attitude toward use is determined by perceived ease of use and perceived usefulness. Perceived usefulness is influenced by perceived ease of use. The technology acceptance model has come to be one of the most widely used models in information system research, in part because of its understandability and simplicity (King and He, 2006). A number of information system studies have begun to show that continued information system use is explained by the technology acceptance model (Hong et al., 2006; Ifinedo, 2006; Kim and Malhotra, 2005; Liao, Palvia and Chen, 2009; Roca, Chiub and Martinez, 2006; Venkatesh et al., 2011). Although the technology acceptance model has received considerable empirical validation in predicting continued information system use, many information system researchers have incorporated additional variables into the model for the sake of theory broadening. Table 1 summarizes the relationships among variables in the literature in the context of continued information system use.
Studies | Context | Findings |
---|---|---|
Karahanna et al. (1999) | Windows technology | The authors found that perceived usefulness and image exert positive effects on attitude. Attitude is an antecedent of continued intention; however, subjective norms do not influence continued intention. Top management, supervisor, peers and computer specialists are determinants of subjective norms. |
Hu et al. (2003) | Microsoft PowerPoint | The authors indicated that perceived usefulness and computer self-efficacy show positive effects on continued intention. Perceived ease of use and job relevance have positive effects on perceived usefulness; however, subjective norms and compatibility have negative effects on perceived usefulness. In addition, compatibility and computer self-efficacy have positive influences on perceived ease of use. |
Gefen (2003) | Online shopping | The authors supported that perceived ease of use, perceived usefulness and habit exert positive effects on continued intention. Habit is a major predictor of perceived ease of use and perceived usefulness among experienced shoppers. |
Lippert and Forman (2005) | Collaborative visibility network | The authors reported that prior experience with a similar technology, the opportunity to experiment, prior technological knowledge and training effectiveness exert positive effects on perceived ease of use and perceived usefulness, which in turn, influence technology performance. Perceived ease of use and the opportunity to experiment are two antecedents of perceived usefulness. In addition, technology performance influences continued use. |
Hong et al. (2006) | Mobile Internet | The authors pointed out that perceived ease of use, perceived usefulness and satisfaction show positive effects on continued use. Perceived ease of use and confirmation have positive effects on perceived usefulness. In addition, confirmation has a positive effect on perceived ease of use, perceived usefulness and satisfaction. |
Ifinedo (2006) | Web-based learning technology | The authors revealed that perceived ease of use, technology characteristics and user characteristics have positive effects on perceived usefulness. Technology characteristics and user characteristics exert positive effects on perceived ease of use. Moreover, perceived ease of use and perceived usefulness exert positive influences on continued intention. |
Roca et al. (2006) | e-learning system | The authors supported that continued intention is determined by satisfaction, which in turn is jointly determined by perceived ease of use, perceived usefulness, cognitive absorption, confirmation, information quality, system quality and service quality. |
Premkumar and Bhattacherjee (2008) | Computer-based tutorials | The authors indicated that perceived ease of use, perceived usefulness and satisfaction exert positive effects on continued intention. Perceived ease of use is a determinant of perceived usefulness. In addition, disconfirmation and performance have positive effects on satisfaction. |
Wu and Kuo (2008) | Google search engine | The authors found that the predicting power of perceived ease of use and perceived usefulness on continued intention is weakened by the addition of either habitual use or past use. In addition, the relationships between the evaluations of perceived ease of use/perceived usefulness and continued intention are weakened when stronger habit is considered. |
Liao et al. (2009)) | e-learning system | The authors reported that perceived ease of use and perceived usefulness exert positive effects on attitude. Attitude and perceived usefulness are two important determinants of continued intention. Perceived ease of use is an antecedent of perceived usefulness. In addition, the authors also found that satisfaction exerts a positive effect on attitude and continued intention. Confirmation and perceived usefulness are two determinants of satisfaction. |
Mäntymäki and Salo (2011) | Social virtual worlds | The authors found that perceived enjoyment, perceived ease of use and perceived usefulness have positive effects on attitude. Attitude, perceived enjoyment and perceived usefulness show positive effects on continued intention. In addition, perceived ease of use exerts a positive effect on perceived enjoyment and perceived usefulness. |
Venkatesh et al. (2011) | SmartID and GovWeb | The authors revealed that perceived ease of use, perceived usefulness, facilitating conditions and trust have positive effects on attitude toward SmartID and continued intention to use SmartID. Attitude toward SmartID is an antecedent of continued intention to use SmartID. In addition, perceived ease of use, perceived usefulness, social influence and trust have positive effects on attitude toward GovWeb and perceived ease of use and perceived usefulness have positive effects on continued intention to use GovWeb. Attitude toward GovWeb is an important determinant of continued intention to use GovWeb. |
Alwahaishi and Snásel (2013) | Information and communications technology | The authors indicated that perceived usefulness, social influence, facilitating conditions and perceived playfulness show positive effects on continued intention. |
Sun and Jeyaraj (2013) | Course management system | The authors found that perceived usefulness, social influence and perceived compatibility show positive effects on continued intention; however, perceived ease of use and facilitating conditions did not affect continued intention. |
Bhattacherjee and Lin (2015) | Insurance work system | The authors supported that habit, continued intention and satisfaction exert positive effects on continued use. Habit negatively moderates the relationship between continued intention and continued use. Subject norms, perceived usefulness and satisfaction influence continued intention. |
The theory of interpersonal behaviour
The role of affect on intention
Attitude has been regarded as a powerful predictor for particular behaviour. A person with a favourable attitude is expected to perform a favourable behaviour. The social psychology literature (Ajzen, 2002; Conner and Sparks, 2005; Hagger and Chatzisarantis, 2005) has shown that attitude contains two specific components: cognitive and affective. In Triandis’s (1980) theory of interpersonal behaviour, the affective component of attitude (affect) is particularly expected to predict behaviour. Affect refers to the feelings of joy, elation or pleasure or the feelings of depression, disgust, displeasure or hate that an individual associates with a particular act (Triandis, 1980). Positive feelings will increase the intention to use an information system, while negative feelings will decrease the intention to use an information system. Previous research (Chang and Cheung, 2001; Limayem and Hirt, 2003; Pahnila and Warsta, 2010; Thompson, Higgins and Howell, 1991, 1994) in the theory of interpersonal behaviour has supported the relationship between affect and intention in information use.
The role of social factors on intention
Social factors that correspond to subjective norms in the theory of planned behaviour (Bergeron et al., 1995) refer to an individual’s internalization of the reference groups’ subjective culture and specific interpersonal agreements that the individual has made with others in specific social situations (Triandis, 1980). Social factors as direct determinants of behavioural intention are represented as subjective norms in the theory of planned behaviour (Ajzen, 1991) and the second version of the technology acceptance model (Venkatesh and Davis, 2000), and they are represented as a social influence in the unified theory of acceptance and use of technology (Venkatesh et al., 2003). While they have different labels, each of these constructs contains the explicit or implicit notion that an individual’s behaviour is influenced by the way in which he or she believes that others will view him or her as a result of having used the technology (Venkatesh et al., 2003). Because technology use occurs within a social environment, it has been suggested that social factors be included in theoretical models of technology acceptance (Sirte and Karahanna, 2006). The more positive (favourable) the social factors regarding intention to use an information system are, the more likely it is that individuals will intend to use the information system. Empirical support for the relationship between social factors and intention can be found in the theory of interpersonal behaviour literature (Chang and Cheung, 2001; Karahanna et al., 1999; Limayem and Hirt, 2003).
The effect of habit on behaviour
Triandis (1980) defined habit as situation-behaviour sequences that are or have become automatic, so that they occur without self-instruction. So when behaviour is habit-driven, a person does not think about it and it diminishes the conscious attention with which acts are performed. Habit has always been conceptualized as frequency of past behaviour. However, it can be argued that repetition of behaviour is a necessary, but not a sufficient condition to define behaviour as habit (Limayem et al., 2007). In addition to repetition, habits are defined by the automatic, non-reflective nature of acting. Previous work in the theory of interpersonal behaviour research (Cheung and Limayem, 2005; Limayem and Hirt, 2003; Limayem, Khalifa and Chin, 2004; Pahnila and Warsta, 2010) has confirmed that habit can influence individuals’ information system use.
The effect of facilitating conditions on behaviour
Except for totally volitional behaviour, people require the necessary resources and support to perform behaviour (Cheung, Chang and Lai, 2000). To predict non-volitional behaviour, Triandis (1980) considered facilitating conditions in the theory of interpersonal behaviour. Facilitating conditions refer to objective factors that are out there in the environment, which several judges or observers can agree make an action easy to perform (Triandis, 1980). Limayem and Hirt (2003) indicated that even if a person has the intention to perform a particular behaviour or habitually performs that behaviour, the behaviour may not occur when the facilitating conditions do not permit it. This finding is similar to the concept of perceived control in Ajzen’s theory of planned behaviour. Perceived control is a construct that reflects situational enablers or constraints to behaviour (Ajzen, 1985). Ajzen’s conceptualisation of perceived control refers to internal and external constraining factors. Facilitating conditions are described as factors in the environment that encourage or discourage behaviour and that can be regarded as an external control (Venkatesh, 2000). Previous work in the theory of interpersonal behaviour research (Bergeron, Raymond, Rivard and Gara, 1995; Cheung et al., 2000; Limayem, Khalifa and Chin, 2004; Thompson et al., 1991, 1994) has supported the relationship between facilitating conditions and information system use.
The role of perceived consequences on intention
In addition to affect and social factors, Triandis (1980) proposed a third factor, perceived consequences, that influences intention. Triandis argued that each act is perceived as having potential consequences that have value, together with a probability that the consequence will occur (Bergeron et al., 1995; Thompson et al., 1991). The theory of interpersonal behaviour hypothesizes that the higher the expected value of an act is, the more likely it is that a person will intend to perform it. From the perspective of information system use, Triandis found that perceived consequences are consistent with perceived usefulness. Perceived usefulness is defined as a person’s expectation that using a particular system will enhance his or her job performance (Davis et al., 1989). In other words, perceived usefulness is the expected value (e.g., better job performance) of using a particular information system. Thus, we could regard perceived usefulness as being synonymous with perceived consequences in information system use. The previous theory of interpersonal behaviour research (Chang and Cheung, 2001; Limayem and Hirt, 2003; Limayem et al., 2004) has supported the relationship between perceived consequences and intention in information system use. More specifically, perceived usefulness has been found to exert a positive effect on intention to use a particular information system in the technology acceptance model literature (Davis et al., 1989; Taylor and Todd, 1995; Venkatesh, 2000; Venkatesh and Davis, 2000; Venkatesh et al., 2003).
Results
Measurement model
We first conducted a partial least squares structural equation modelling analysis to examine the item reliability in the technology acceptance model and the theory of interpersonal behaviour. Table 2 and Table 3 show the weights and loadings of the measures to their respective constructs. As Chin (1998) notes, loadings should be interpreted for reflective measures and weights for formative ones. Construct item loadings should exceed 0.7, and item weights should be significant, suggesting item reliability (Hair et al., 2011). As shown in Table 2 and Table 3, all the item loadings are higher than 0.7. Although one item weight for facilitating conditions is insignificant, we decided to retain it, following the suggestion by Henseler et al. (2009) and Jarvis et al. (2003) that researchers should keep both significant and insignificant formative indicators in the measurement model because dropping a formative indicator may omit a unique part of the composite latent construct and change the meaning of the construct. We can confirm that the overall measurement items in the technology acceptance model and the theory of interpersonal behaviour have good item reliability.
Construct | Item | Loading | t-value |
---|---|---|---|
Perceived ease of use | item 1 item 2 item 3 item 4 |
0.906** 0.931** 0.910** 0.914** |
105.011 140.105 121.769 112.892 |
Perceived usefulness | item 1 item 2 item 3 item 4 item 5 |
0.830** 0.907** 0.911** 0.849** 0.878** |
60.252 107.767 127.604 76.281 80.758 |
Affect | item 1 item 2 item 3 |
0.838** 0.890** 0.895** |
61.527 93.008 156.172 |
Cognitive attitude | item 1 item 2 item 3 |
0.916** 0.926** 0.901** |
146.214 135.156 88.579 |
Intention to continue use | item 1 item 2 |
0.962** 0.960** |
307.310 258.622 |
Continued use | item 1 item 2 |
0.921** 0.815** |
45.838 213.540 |
Note: **p<0.01 |
Construct | Item | Loading | Weight | t-value |
---|---|---|---|---|
Perceived usefulness | item 1 item 2 item 3 item 4 item 5 |
0.829** 0.907** 0.911** 0.849** 0.879** | 58.569 106.918 129.119 72.828 81.760 | |
Affect | item 1 item 2 item 3 |
0.842** 0.894** 0.889** |
63.158 96.697 133.501 | |
Intention to continue use | item 1 item 2 |
0.962** 0.960** |
314.275 256.216 | |
Continued use | item 1 item 2 | 0.823** 0.915** | 54.217 233.417 | |
Habit | item 1 item 2 item 3 item 4 item 5 |
0.925** 0.862** 0.884** 0.815** 0.904** | 185.961 92.867 87.448 47.017 111.622 | |
Social factors | item 1 item 2 item 3 |
0.933** 0.963** 0.957** | 123.381 219.903 260.432 | |
Facilitating conditions | item 1 item 2 item 3 item 4 item 5 | 0.468** 0.096* 0.030 0.472** -0.394** |
7.069 1.808 0.055 6.796 10.600 | |
Note: *p<0.05,**p<0.01 |
In addition, we assessed the convergent validity by examining the composite reliability and average variance extracted from the measures in Table 4 and Table 5. The average variance extracted should be at least 0.5, and the composite reliability should be higher than 0.7 (Hair et al., 2014). Table 4 and Table 5 indicate that all of the average variance extracted and composite reliability exceed the cut-off values listed above. Thus, we can confirm that the measurement models in the technology acceptance model and the theory of interpersonal behaviour have adequate convergent validity. Moreover, we assessed discriminant validity by looking at the square root of the average variance that was extracted for each construct (Bock et al., 2005). Table 4 and Table 5 indicate that the square root of the average variance that was extracted for each construct is greater than the levels of correlations that involve the construct, thereby confirming the discriminant validity in the technology acceptance model and the theory of interpersonal behaviour.
Perceived ease of use | Perceived usefulness | Affect | Cognitive attitude | Intention to continue use | Continued use | |
---|---|---|---|---|---|---|
Perceived ease of use | 0.92 | |||||
Perceived usefulness | 0.73** | 0.88 | ||||
Affect | 0.57** | 0.74** | 0.88 | |||
Cognitive attitude | 0.69** | 0.81** | 0.79** | 0.92 | ||
Intention to continue use | 0.65** | 0.76** | 0.77** | 0.80** | 0.96 | |
Continued use | 0.49** | 0.57** | 0.61** | 0.57** | 0.64** | 0.87 |
Average variance extracted | 0.84 | 0.77 | 0.77 | 0.84 | 0.92 | 0.76 |
Composite reliability | 0.95 | 0.94 | 0.91 | 0.94 | 0.96 | 0.86 |
Mean | 5.80 | 5.48 | 5.09 | 5.54 | 5.50 | 5.64 |
Standard deviation | 1.09 | 1.07 | 1.10 | 1.04 | 1.20 | 1.33 |
Note: The red numbers on the diagonal are square roots of average variance extracted. ** Correlation is significant at the 0.01 level. |
Perceived ease of use | Perceived usefulness | Affect | Cognitive attitude | Intention to continue use | Continued use | |
---|---|---|---|---|---|---|
Perceived ease of use | 0.88 | |||||
Perceived usefulness | 0.74** | 0.88 | ||||
Affect | 0.76** | 0.77** | 0.96 | |||
Cognitive attitude | 0.57** | 0.61** | 0.64** | 0.87 | ||
Intention to continue use | 0.70** | 0.76** | 0.76** | 0.75** | 0.88 | |
Continued use | 0.48** | 0.58** | 0.47** | 0.35** | 0.51** | 0.95 |
Average variance extracted | ">0.77 | 0.77 | 0.92 | 0.76 | 0.77 | 0.90 |
Composite reliability | 0.94 | 0.91 | 0.96 | 0.86 | 0.94 | 0.97 |
Mean | 5.48 | 5.09 | 5.50 | 5.64 | 5.26 | 4.69 |
Standard deviation | 1.07 | 1.10 | 1.20 | 1.33 | 1.22 | 1.17 |
Structural path model
The second step was to analyse and compare the two structural models (the technology acceptance model and the theory of interpersonal behaviour model). Following the suggestions of Hair, Ringle and Sarstedt (2014), we performed a bootstrapping procedure (with 5,000 sub-samples) to test the statistical significance of each path coefficient in the two models. Figure 2 describes the structural model coefficients in the technology acceptance model. All relations postulated by the technology acceptance model were confirmed empirically. Affect, cognitive attitude and perceived usefulness explain 70.9% variance of intention to continue use. Intention to continue use explains 50.5% variance of continuance behaviour. Figure 3 describes the structural model coefficients in the theory of interpersonal behaviour. All relations postulated by the theory of interpersonal behaviour were confirmed empirically with one exception: social factors do not have direct influences on intention to continue use. Affect, social factors and perceived usefulness explain 68% variance of intention to continue use. Habit, intention to continue use and facilitating conditions explain 65.3% variance of continuance behaviour. According to Figure 2 and Figure 3, we may see important reasons for model integration. The technology acceptance model explains more variance of intention to continue use than the theory of interpersonal behaviour; however, the theory of interpersonal behaviour explains more variance of continuance behaviour than the technology acceptance model. By combining the two models, we may obtain an integrated model with higher predictive power for continued use of information systems.
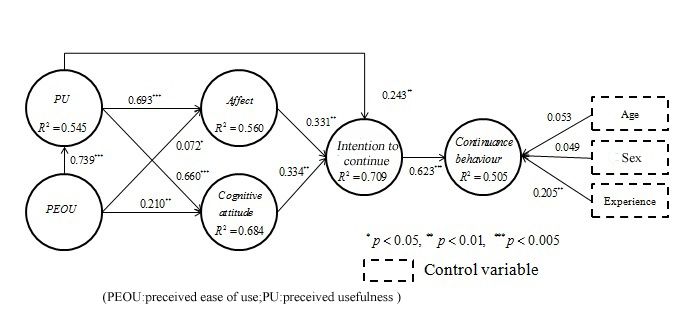
Figure 2: Technology acceptance mode
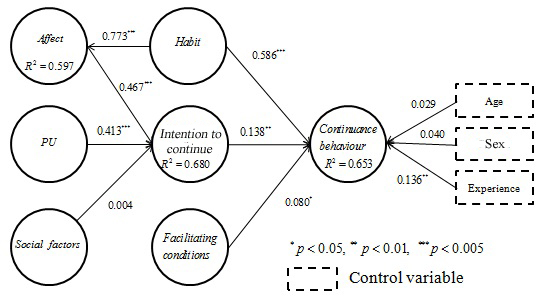
Figure 3: The theory of interpersonal behaviour model.
Test of an integrated model
The main purpose of this study is to examine whether the two theoretical models can be integrated to explain continued information system use properly. In addition to the bootstrapping procedure, we applied the product-indicator approach (Chin, Marcolin and Newsted, 2003) to detect the moderating effects of habit. Figure 4 presents the structural model coefficients in the integrated model.
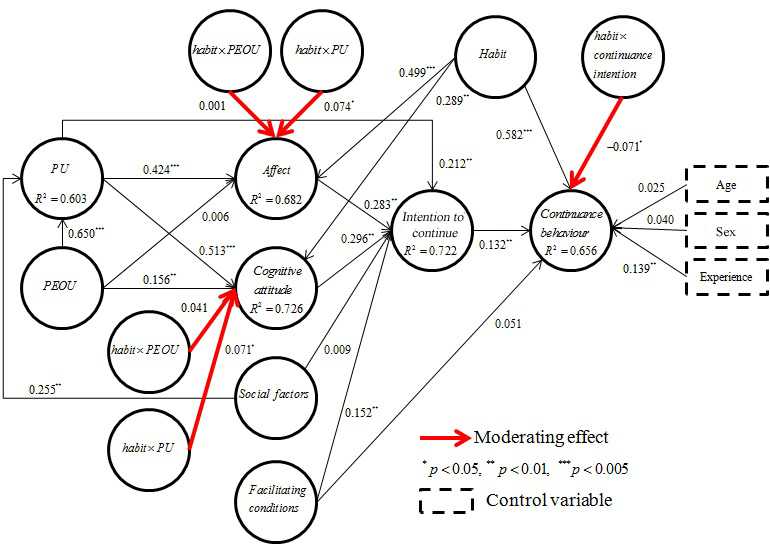
Figure 4: The integrated research model. [Click for larger image]
The R2 value of 0.603 indicates that the model explains a good amount of variance in perceived usefulness. The R2value of 0.682 indicates that the model explains a good amount of variance in affect. The R2value of 0.726 indicates that the model explains a good amount of variance in cognitive attitude. The R2 value of 0.722 indicates that the model explains a good amount of variance in intention to continue use. The R2 value of 0.656 indicates that the model explains a good amount of variance in continuance behaviour. Habit (-0.071) negatively moderated the relationship between intention to continue use and actual continued use. Moreover, habit positively moderates the perceived usefulness-affect (0.074) and perceived usefulness-cognitive attitude (0.071) relations. According to our findings, H4 and H5 were supported; however, H1, H2, H3 were supported partially. We also confirmed H6, H7b and H7d. With regard to the three control variables included in the integrated model, all but user experience were not significantly related with continuance behaviour. Thus, the exact role of user experience in continuance behaviour may not be ignored. Past studies usually regarded user experience as a moderating role in evaluating individuals’ behaviour. To assess the moderating role of user experience, we used multiple group analysis as proposed by Keil et al. (2000) and Henseler et al. (2009) to see whether different parameter estimates occur for each group. Because 73.2% of the participants have user experience of more than five years, we divided our sample into two groups with respect to user experience (i.e.,≤5 years >5 years ). Table 6 summarizes the results for all the relationships in the integrated model. As can be seen, only two relationships (path coefficients) differ significantly across the two groups. The impact of intention to continue use on continued use is significantly stronger for participants with light user experience (≤5 years ). In addition, the moderating effect of habit on the relationship between intention to continue use and actual continued use is significantly stronger for participants with heavy user experience (>5 years ).
Group 1: ≤5 years | Group 2: >5 years | Group 1 vs. Group 2 | |||||
---|---|---|---|---|---|---|---|
p(1) | se(p(1)) | p(2) | se(p(2)) | |p(1)-p(2)| | tKeil et al.value | PHens elervalue | |
perceived ease of use → perceived usefulness | 0.651** | 0.045 | 0.633** | 0.024 | 0.018 | 0.353 | 0.372 |
perceived ease of use →affect | 0.005 | 0.038 | 0.011 | 0.037 | 0.006 | 0.604 | 0.486 |
perceived usefulness→affect | 0.370** | 0.079 | 0.436** | 0.041 | 0.066 | 0.743 | 0.738 |
perceived ease of use → cognitive attitude | 0.209** | 0.058 | 0.139* | 0.035 | 0.07 | 1.035 | 0.155 |
perceived usefulness→ cognitive attitude | 0.434** | 0.062 | 0.546** | 0.042 | 0.112 | 1.498 | 0.941 |
affect→intention to continue use | 0.284** | 0.064 | 0.289** | 0.044 | 0.005 | 0.064 | 0.517 |
cognitive attitude→ intention to continue use | 0.313** | 0.070 | 0.284** | 0.046 | 0.029 | 0.347 | 0.371 |
social factors→ intention to continue use | 0.054 | 0.033 | 0.028 | 0.023 | 0.026 | 0.647 | 0.228 |
perceived usefulness→ intention to continue use | 0.210** | 0.067 | 0.219** | 0.041 | 0.009 | 0.114 | 0.536 |
facilitation conditions→ intention to continue use | 0.198* | 0.059 | 0.132* | 0.028 | 0.066 | 1.012 | 0.149 |
intention to continue use→ continuance behaviour | 0.277** | 0.070 | 0.097* | 0.040 | 0.18 | 2.294* | 0.019* |
habit→continuance behaviour | 0.556** | 0.065 | 0.611** | 0.041 | 0.055 | 0.702 | 0.759 |
facilitating conditions→ continuance behaviour | 0.012 | 0.032 | 0.068 | 0.035 | 0.056 | 1.182 | 0.866 |
habit→affect | 0.536** | 0.062 | 0.477** | 0.030 | 0.059 | 0.858 | 0.248 |
habit→cognitive attitude | 0.328** | 0.057 | 0.262** | 0.033 | 0.066 | 1.023 | 0.154 |
social factors→ perceived usefulness | 0.276** | 0.049 | 0.253** | 0.025 | 0.023 | 0.419 | 0.350 |
habit×perceived ease of use →affect | 0.060 | 0.065 | 0.022 | 0.065 | 0.038 | 0.414 | 0.275 |
habit×perceived usefulness→ affect | 0.008 | 0.082 | 0.042 | 0.048 | 0.034 | 0.364 | 0.652 |
habit×perceived ease of→ cognitive attitude | 0.052 | 0.051 | 0.086 | 0.047 | 0.034 | 0.491 | 0.713 |
habit×perceived usefulness→ cognitive attitude | 0.005 | 0.045 | 0.098* | 0.047 | 0.093 | 1.431 | 0.942 |
habit×intention to continue use→ continuance behaviour | -0.001 | 0.021 | -0.094* | 0.028 | 0.093 | 2.66** | 0.009** |
N | 309 | 845 | |||||
Note: p(1) and p(2) are path coefficients of Group 1 and Group 2, respectively; se(p(1)) and se(p(2)) are the standard error of p(1) and p(2) , respectively. *p<0.05,**p<0.01 |
Discussion
The appropriateness of model integration between the technology acceptance model and the theory of interpersonal behaviour
The main purpose of this study is to examine whether the technology acceptance model and the theory of interpersonal behaviour can be combined to properly explain information system use. To this end, we need to understand the difference between the two theoretical models. According to our results, all the hypotheses derived from the technology acceptance model were confirmed. The theory of interpersonal behaviour supported all the hypotheses concerning the relationships between constructs, with one exception. Concerning the ability to predict continuance behaviour, our findings indicate that the power of the technology acceptance model is lower than that of the theory of interpersonal behaviour. However, concerning the ability to predict intention to continue use, our findings indicate that the power of the theory of interpersonal behaviour is lower than that of the technology acceptance model. From a theoretical point of view, our results suggest that the technology acceptance model and the theory of interpersonal behaviour have their own advantages in predicting different behavioural aspects of continued use of information systems, and thus show the possibility of model integration. In other words, we think that the results of this study support the perspective that the two models should not be viewed as alternative but as supplementary models. The technology acceptance model and the theory of interpersonal behaviour were developed in different research contexts. Our integration between the technology acceptance model and the theory of interpersonal behaviour may provide a sound theoretical framework that includes factors identified as important determinants of continued use, thereby broadening the technology acceptance model.
In addition, our results show that the theory of interpersonal behaviour has sufficient predictive power in terms of intention to continue use (R2=0.680) and continuance behaviour (R2=0.653). From a theoretical perspective, although the theory of interpersonal behaviour has been examined empirically in the context of social-psychological behaviour, our results extend the application of the theory of interpersonal behaviour to an information system context and we regard this theory as an information systems predictive model.
The importance of habit in predicting continued use of information systems
Our results indicated that H1 was supported partially. That is, we confirmed that habit and intention to continue use have effects on continuance behaviour; however, facilitating conditions did not exert an effect on continuance behaviour. In particular, we found that habit has a larger effect on continued use than intention to continue use. This finding implied that habit should be a determinant in predicting continued Webmail use. Our multiple group analysis reported that the impact of intention to continue use on continuance behaviour is significantly stronger for Webmail users with light experience than for Webmail users with heavy experience. Our results are similar to the findings of Kim and Molhotra (2005) and Venkatesh et al (2012), indicating that the influence of use intention on Web-based information technology use is stronger for light users than for heavy users. The theory of planned behaviour and the theory of reasoned analysis emphasize that the most proximal cause of behaviour is intention. When Webmail users have light experience, they may need to consider deliberately the continued Webmail use because the online environment usually involves some uncertainty and risk related to privacy, security or fraud. Thus, continuous willingness to use Webmail may exert stronger influence on continued Webmail use for Webmail users with light experience. In addition, Figure 4 shows that the path coefficient from habit×intention to continue use to continuance behavior is -0.071. That is, H6 was confirmed and habit negatively moderates the relationship between intention to continue use and continuance behaviour. Our multiple group analysis also showed that the relationship from habit×intention to continue use to continuance behavioris significantly different ( p<0.01) between heavy experience users and light experience users. In other words, we found that the moderating effect of habit on the relationship between intention to continue use and continuance behaviour is stronger for heavy experience users than for light experience users. Although several studies (Limayem and Hirt, 2003; Limayem et al., 2007) have revealed that in the continued use of information systems stage habit negatively moderates the relationship between intention to continue use and continuance behaviour, these studies did not explicitly clarify whether the moderating effect of habit on the intention to continue use–continuance behaviour relation is associated with user experience. On the contrary, our study explicitly accounts for user experience and the moderating role of habit simultaneously, and exhibits that the moderating impact of habit on the intention to continue use–continuance behaviour relation significantly differs across two groups (light experience users vs. heavy experience users). We believe that these findings provide researchers with an in-depth understanding of the moderating role of habit on continued use of information systems from the perspective of different user experience levels on which further research can be built. From a managerial perspective, managers and decision makers should understand that while it might be reasonable to try to shape people’s intentions during the early system adoption stages, later on, the same strategies will not have the same effect because it is not intention to continue uses but habits that govern a person’s continued use.
According to our results, H3 was supported partially and H4 was supported. That is, habit and perceived usefulness exert effects on affective attitude. Habit, perceived ease of use and perceived usefulness exert effects on cognitive attitude. According to these findings, habit is positively related to the two-component attitude structure. In addition, H7b and H7d were supported. That implies that habit positively moderates the relationships between perceived usefulness and affect and between perceived usefulness and cognitive attitude. The effect of the perceived usefulness on the two components of attitude changes linearly with respect to the habit, because the stronger the habit that is established on continued use of a system, the more experiences the user gains, which helps them adjust their perception of the system’s usefulness. As a result, perceived usefulness increases a user’s liking (affect) and positive evaluation (cognitive attitude) toward continued use of a system as habit establishes. Taken together, our findings explicitly clarify that habit is helpful for improving the affective and cognitive evaluation of a technology. From a theoretical perspective, our findings support the notion of a feedback mechanism where a habitual behaviour plays an important role in affecting system evaluation.
The impact of the two components of attitude on continued use of information systems
Attitude is traditionally regarded as a unidimensional construct, and it is regarded as a powerful factor in predicting behaviour. However, the social psychology literature (Ajzen, 2002; Conner and Sparks, 2005; Hagger and Chatzisarantis, 2005) has indicated that overall attitude should be separated into two specific components: cognitive (e.g., worthless and valuable) and affective (e.g. unpleasant and pleasant). The technology acceptance model and its variants use unidimensional attitudes to predict information system use (Davis, 1993; Davis et al., 1989; Taylor and Todd, 1995). Unlike previous technology acceptance models, we simultaneously used affective and cognitive attitudes to predict continued use of information systems. According to Figure 4, H2 was supported partially. That implies that affective attitude, cognitive attitude, facilitating conditions and perceived usefulness show effects on intention to continue use. In particular, cognitive attitude exerts a stronger impact on intention to continue use than affective attitude, perceived usefulness, social factors and facilitating conditions. This result also indicates that affective and cognitive attitudes have larger impacts on intention to continue use than perceived usefulness and facilitating conditions. Our results are consistent with Venkatesh et al.’s (2011) extended unified theory of acceptance and use of technology, which suggests attitude is a stronger predictor than perceived ease of use, perceived usefulness, social influence or facilitating conditions for changes in intention to continue use. Specifically, our results indicated the importance of cognitive attitude. Although previous studies employing the technology acceptance model claimed that perceived usefulness is the strongest predictor of behavioural intention, our results revealed that attitude, especially cognitive attitude, rather than perceived usefulness, has more influence on intention to continue use. In addition, the attitude construct is regarded as a core construct in the theory of planned behaviour and the theory of reasoned analysis. Ajzen (1991) claimed that the theory of reasoned analysis and theory of planned behaviour will be rejected if attitude does not predict intention. To further confirm the importance of the two-component attitude structure, we dropped it from our integrated model and performed a partial least squares structural equation modelling analysis. We found that the effect size (f2 ) of the two-component attitude structure on intention to continue use is 0.252. The f2 values of 0.02, 0.15 and 0.35 indicate an exogenous construct’s small, medium or large effect, respectively, on an endogenous construct (Hair et al., 2014). We may conclude that the two-component attitude structure can contribute to R2intention to continue use due to a medium f2 effect size.
In contrast to the perspective of attitude ignorance, our results indicated that the two-component attitude structure has an important impact on the perceived usefulness–intention to continue use relation. Both affective and cognitive attitudes partially mediate the perceived usefulness–intention to continue use relation. That is to say, the effect of perceived usefulness in directly explaining intention to continue use diminishes significantly, which is contrary to the findings of many technology adoption studies. In addition, for light experience users and heavy experience users, our multiple group analysis also reported that perceived ease of use and perceived usefulness influence cognitive attitude but perceived usefulness influences affective attitude. Our findings explicitly distinguish the effects of perceived ease of use and perceived usefulness on affective or cognitive attitude from the perspective of user experience.
From a theoretical perspective, our findings regarding the effect of attitude on intention to continue use, the f2 effect size and multiple group analysis are very powerful results because they suggest that researchers should consider two-component attitude for better explaining intention to continue use of a technology. These findings suggest that researchers should be careful when removing attitude from their models of the technology adoption of individuals. It is important to consider these findings in the context of the technology adoption literature, which often ignores attitude or treats attitude as a unidimensional construct. Although this two-component attitude structure is supported across multiple attitude measurement methods and conceptualizations in research on the theory of planned behaviour, it is found relatively rarely in technology adoption research. For example, Davis et al. (1989) reported that attitude adds little value in explaining information system use. The attitude construct has often been ignored in research on technology acceptance, such as Venkatesh and Davis (2000), Venkatesh (2000) and Venkatesh et al. (2003). This lack of value in explaining information system use may have resulted because Davis et al. (1989) treated attitude as affect; however, the indicators for the attitude construct that they used included both cognitive and affective aspects. Thus, Davis et al.’s (1989) conclusions are plausible and need to be deliberately explained. We suggest that future continued use of information systems research deliberately examine the role of attitude by including affective and cognitive attitudes. From a managerial perspective, we suggest that managers and decision makers use policies or methods to reinforce users’ positive affect and cognitive attitude that will keep a targeted technology adopted.
Facilitating conditions as determinants of intention to continue use and not continuance behaviour
According to H1 and H2, the effect of facilitating conditions on intention to continue use was supported; however, facilitating conditions did not exert significant effects on continuance behaviour. This finding is in line with the result of Martins et al. (2014) that users are not concerned about the surrounding environment (facilitating conditions) so as to influence the use of Internet banking. We performed an additional partial least squares structural equation analysis, in which the path coefficient of facilitating conditions increased from 0.051 to 0.181; moreover, the analysis showed a significant effect on continuance behaviour by removing habit from our research model. One possible explanation for this finding might be that when the impact of habit is considered, Webmail users are more concerned about habit than environmental constraints to determine the continuance behaviour of Webmail. Ajzen (1991) argued that for behaviour without problems of volitional control, perceived control will contribute nothing to the prediction of behaviour. In our study, facilitating conditions include Internet accessibility and support for Webmail systems. Because information technology adoption is prevalent and Internet penetration is very high in Taiwan, we speculate that individuals may have sufficient Internet skills once they develop habit to use Webmail in everyday life. As a result, individuals may not need any assistance to use Webmail, indicating that facilitating conditions are not barriers for predicting the continuance behaviour of Webmail.
Social factors as indirect determinants of intention to continue use through perceived usefulness
According to H2, our results indicate that social factors have no direct effects on intention to continue use in the context of the information system. Even though the theory of planned behaviour suggests the effect of social norms on intention, such effect is not still clear in the context of social psychology or information systems (Armitage and Conner, 2001; Chau and Hu, 2001;Mathieson, 1991; Shepperd et al., 1988; Van den Putte, 1991). We believe that future research needs to further explore such effect. In addition, H5 shows that social factors and perceived ease of use have effects on perceived usefulness. In other words, our results indicate that social factors can influence intention to continue use indirectly through perceived usefulness. This finding is consistent with Venkatesh and Davis’ (2000) second version of the technology acceptance model and the subsequent research. Similarly, Pontiggia and Virili (2010) reported that network effects (e.g., social effects) may push users toward acceptance by enhancing the perceived usefulness of a targeted system. According to Deutsch and Gerard (1955), individuals’ perceptions of norms consist of two influences: informational and normative. They defined normative social influence as influence to conform to the positive expectations of another. Informational social influence may be defined as influence to accept information that is obtained from another as evidence about reality. When people accept such information, they may adjust their own beliefs about reality. Hogarth and Einhorn’s (1992) belief updating theory can explain this inference. Belief updating theory suggests that prior knowledge is adjusted by the impact of succeeding pieces of evidence. From the perspective of belief updating, in the Webmail use context, if a friend who is important to a person suggests that a particular Webmail system might be useful (evidence), a person may come to adjust his or her prior belief to believe that the Webmail system actually is useful (belief updating) and, in turn, form a intention to continue use to use it. That is, social factors have indirect effects on intention to continue use through perceived usefulness, as opposed to a direct compliance effect on intention to continue use. Although the relationship between social factors and perceived usefulness does not differ significantly across two groups (light experience users vs. heavy experience users), our multiple group analysis reported that the path coefficient from social factors to perceived usefulness is smaller for heavy experience users than for light experience users. From a theoretical perspective, our multiple group results may contribute to the information system literature by indicating that the direct effects of social factors on perceived usefulness will decrease with heavy user experience. This is because heavy user experience may help to better understand a technology’s strengths and weaknesses, supplanting reliance on social cues as a reference for usefulness perceptions. From a managerial perspective, managers and decision makers may provide online word-of-mouth systems that allow people to share their opinions regarding Webmail use. Perhaps by eliciting current Webmail users to post positive information toward Webmail’s usefulness on the system, potential users may be persuaded to recognize Webmail as a useful tool and thus plan to use Webmail in the future.
Conclusions and limitations
Our results indicate that the technology acceptance model and the theory of interpersonal behaviour should not be viewed as alternative but as supplementary models. In summary, we applied the technology acceptance model and the theory of interpersonal behaviour as two theories to predict continued use of information systems. We believe our findings provide researchers with a better understanding of continued use by bridging the fields of information systems and social psychology. In addition to the fact that most of the hypothesized relationships were supported in the two theories, we also found that crossover effects exist between them. For example, our results reported that habit positively moderates the effect of perceived usefulness on cognitive attitude and affect. Social factors have effects on perceived usefulness and, in turn, influence intention to continue use. Our findings with respect to crossover effects may contribute to the information systems literature by confirming that the two theories are interdependent and supplementary in predicting continued use.
As with all other studies, this study is not without its limitations. The first limitation concerns survey method. Because we adopted the viewpoint of social networks to select the sample, our results may be threatened by self-selection bias or lack of sample representativeness. Our sample may only comprise our friends or friends’ friends. Thus, we did not access individuals who were not included in our or friends’ social networks. For example, we found that 81% of our participants came from Northern and Southern Taiwan. In addition, 57.6 % of our participants had a bachelor’s degree. From these perspectives, our results should be limited to interpreting the perceptions of participants who had a bachelor’s degree and lived in two areas (Northern and Southern Taiwan). In other words, our sample may be biased toward individuals who had higher educational status and lived in these two areas in Taiwan. Although Web survey methods cost less and lead to faster transmission, their use raises concerns in terms of sample representativeness. Since we investigated Webmail users in Taiwan, our survey populations were geographically and demographically diverse. We suggest that future research use Facebook’s advertising tool to create advertisements across Facebook users who live in five big areas (Northern, Central, Southern, Eastern and Offshore Taiwan). By doing so, a wider sample may be selected and self-selection bias can be reduced. Because we adopted the viewpoint of social networks, our e-mail invitation was sent to our friends or friends’ friends. Such invitation may be perceived as rude behaviour or spam. To avoid such perceptions, we have included a brief description of the purpose of this study in the e-mail invitation. However, someone may still delete the unwanted e-mail because they may have no interest in our investigation. To increase participants’ interest and reduce non-response rate, following Wright (2005), we suggest that future researchers create a study report, highlighting the most interesting results to the participants, post it on their personal Webpages or social network sites (e.g., Facebook) and provide all participants in advance with a link to their personal Webpages or social network sites. By doing so, study results can be presented and participants can understand them clearly. As a result, participants’ motivation to join the survey may be increased.
The second limitation concerns the generalization of this study. Although we deliberately designed our study with the selection of an often used information system such as Webmail, it is unclear to what extent our results may be extended to other areas of information system use. Webmail is a relatively mature Internet-based information system. Hence, we may not claim that our results will hold equally well in the context of other Internet-based information systems and we suggest that further examination in different contexts such as mature information systems (e.g., Facebook) or new information systems (e.g., LinkedIn) may be needed. Moreover, we employed cross-sectional research design in this study. We suggest that future research use a longitudinal methodology to examine our research model and thereby deepen our understanding of causal relationships between continuance behaviour and cognitive factors. These examinations will allow us to test how generalizable our research model is.
The third limitation concerns the explanatory ability of our research model. Even after combining the technology acceptance model and the theory of interpersonal behaviour, approximately 34% of the variance in continuance behaviour remains unexplained. We believe that further refinement of our model is warranted. Future research should incorporate additional antecedent factors beyond intention to continue use, facilitating conditions and habit. Ajzen’s (1991) theory of planned behaviour maintained that perceived behavioural control has a direct effect on intention and actual behaviour. Perceived behavioural control refers to the appraisals of ease or difficulty for performing behaviour. To strengthen the predictive ability of the theory of planned behaviour, several studies suggested that researchers may distinguish the differentiated components in the theory of planned behaviour. Perceived behavioural control was suggested to divide into self-efficacy and perceived controllability (or labelled facilitating conditions) (e.g., Ajzen, 2002; Courneya et al., 2006; Hagger et al., 2005). Self-efficacy refers to an individual’s self-confidence for engaging in behaviour. Compeau and Higgins (1995) indicated that when an individual believes that he or she has confidence in using a computer, he or she is likely to use the computer. Similarly, Hsu and Chiu (2004) mentioned that Web-specific self-efficacy can exert a direct effect on using e-services. Thus, we suggest to add self-efficacy to our research model to predict continued Webmail use. We focused on Davis et al.’s (1989) technology acceptance model in this study. After Davis (1989) proposed usefulness and ease of use as two determinants to explain technology acceptance behaviour, Davis and his colleagues subsequently produced extended versions of their original model (Davis et al., 1989), such as the version without behavioural intention (Davis 1993), Venkatesh and Davis’ (2000) second version and Venkatesh et al.’s (2003) unified theory of acceptance and use of technology. We suggest that in future research the different versions of the technology acceptance model be integrated with the theory of interpersonal behaviour to predict continued Webmail use.
The fourth limitation concerns model broadening. Although this study combined the technology acceptance model and the theory of interpersonal behaviour to improve the predictive power of continued use of information systems, considering cognitive and habitual aspects may not be sufficient. Future research may incorporate other aspects such as emotion aspects (e.g., positive or negative emotions) into survey design. Contemporary customer behaviour literature supports that an individual’s intention to repurchase products or services is primarily determined by his or her satisfaction with the use of such products or services. An individual will display satisfaction as a positive emotion after he or she consumes specific products or services. Drawing on the concept of satisfaction, Bhattacherjee (2001) proposed the expectation-confirmation model to explain continued information system use. The expectation-confirmation model maintains that users’ intention to continue use is determined by their level of satisfaction and perceived usefulness of the information system. Additionally, the extent of users’ confirmation of expectations positively influences the perceived usefulness of the information system and satisfaction. Perceived usefulness of the information system influences satisfaction. Future research may combine the expectation-confirmation model with our research model to synthesize the aspects of cognition, emotion and habit in predicting Webmail continuance. Given the importance of continued use, we hope that our findings will be valuable to others who engage in developing the theory and practice of the continued information system use.
Acknowledgements
This research was conducted with the support of the Ministry of Science and Technology, Taiwan, R.O.C. (MOST 101-2410-H-156-022) and we also thank Mr. Kun-Chu Chang for his assistance in this study.
About the author
Chi-Cheng Huang is an Associate Professor in the Department of Information Management at Aletheia University, New Taipei City 25103, Taiwan. He received his Ph.D. in the Institute of Public Affairs Management from National Sun Yat-sen University. He may be contacted at chuang@mail.au.edu.tw.
References
- Aarts, H. & Dijksterhuis, A. (2000). Habits as knowledge structures: automaticity in goal-directed behavior. Journal of Personality and Social Psychology, 78(1), 53-63.
- Ajzen, I. (1985). From intentions to actions: a theory of planned behaviour. In J. Kuhl & J. Beckmann (Eds.), Action-control: from cognition to behaviour (pp. 11-39). Heidelberg, Germany: Springer.
- Ajzen, I. (1991). The theory of planned behaviour. Organizational Behaviour and Human Decision Processes, 50, 179-211.
- Ajzen, I. (2002). Constructing a TPB questionnaire: conceptual and methodological considerations. Retrieved from http://www-unix.oit.umass.edu/~aizen/pdf/tpb.measurement.pdf. (Archived by WebCite® at http://www.webcitation.org/6bEQwM0eH)
- Alwahaishi, S. & Snásel, V. (2013). Acceptance and use of information and communications technology: a UTAUT and flow based theoretical model. Journal of technology management & innovation, 8(2), 61-73.
- Armbrust, M., Fox, A., Griffith, R., Joseph, A. D., Katz, R., Konwinski, A., Lee, G., Patterson, D., Rabkin, A., Stoica, I. & Zaharia, M. (2010). A view of cloud computing. Communications of the ACM, 53(4), 50-58.
- Armitage, C. J. & Conner, M. (2001). Efficacy of the theory of planned behaviour: a meta-analytic review. British Journal of Social Psychology, 40(4), 471-499.
- Armbrust, M., Fox, A., Griffith, R., Joseph, A. D., Katz, R., Konwinski, A., ... & Zaharia, M. (2010). A view of cloud computing. Communications of the ACM, 53(4), 50-58.
- Bargh, J.A. (1989). Conditional automaticity: varieties of automatic influence in social perception and cognition. In J.S. Uleman & J. A. Bargh (Eds.), Unintented thought (pp. 3-51). New York, NY: Guilford press.
- Bargh, J.A. & Chartrand, T. L. (1999). The unbearable automaticity of being. American psychologist, 54(7), 462-479.
- Bargh, J.A., Chen, M. & Burrows, L. (1996). Automaticity of social behavior: direct effects of trait construct and stereotype activation on action. Journal of personality and social psychology, 71(2), 230.
- Bergeron, F., Raymond, L., Rivard, S. & Gara, M-F. (1995). Determinants of EIS use: testing a behavioural model. Decision Support Systems, 14(2), 131-146.
- Behrend, T.S., Wiebe, E.N., London, J.E. & Johnson, E.C. (2011). Cloud computing adoption and usage in community colleges. Behaviour & Information Technology, 30(2), 231-240.
- Benbasat, I. & Barki, H. (2007). Quo vadis TAM? Journal of the Association for Information Systems, 8(4), 211-218.
- Bhattacherjee, A. (2001). Understanding information systems continuance: an expectation-confirmation model. MIS Quarterly, 25(3), 351-370.
- Bhattacherjee, A. & Lin, C.P. (2015). A unified model of IT continuance: three complementary perspectives and crossover effects. Journal of Information Systems, 24(4), 364-373.
- Bhattacherjee, A., Perols, J. & Sanford, C. (2008). Information technology continuance: a theoretic extension and empirical test. Journal of Computer Information Systems, 49(1), 17-26.
- Bock, G.W., Zmud, R.W., Kim, Y.G. & Lee, J.N. (2005). Behavioural intention formation in knowledge sharing: examining the roles of extrinsic motivators, social-psychological forces, and organizational climate. MIS Quarterly, 29(1), 87-111.
- Borko, H. (1968). Information science: what is it? American Documentation, 19(1), 3-5.
- Burton-Jones, A. & Hubona, G.S. (2005). Individual differences and usage behavior: revisiting a technology acceptance model assumption. ACM Sigmis Database, 36(2), 58-77.
- Burton-Jones, A. & Hubona, G.S. (2006). The mediation of external variables in the technology acceptance model. Information & Management, 43(6), 706-717.
- Chang, M.K. & Cheung, W. (2001). Determinants of the intention to use Internet/WWW at work: a confirmatory study. Information & Management, 39(1), 1-14.
- Chau, P.Y.K. & Hu, P.J.H. (2001). Information technology acceptance by individual professionals: a model comparison approach. Decision Sciences, 32(4), 699-719.
- Cheung, C.M.K. & Limayem, M. (2005). The role of habit in IS continuance: examining the evolving relationship between intention and usage. Paper presented at the twenty-sixth international conference on information systems, Las Vegas, NV, December 11-14. Retrieved from http://citeseerx.ist.psu.edu/viewdoc/download?doi=10.1.1.231.7431&rep=rep1&type=pdf. (Archived by WebCite® at http://www.webcitation.org/6bER7B9Sm)
- Cheung, W., Chang, M.K. & Lai, V.S. (2000). Prediction of internet and world wide web usage at work: a test of an extended Triandis model. Decision Support Systems, 30(1), 83-100.
- Chin, W.W. (1998). Issues and opinion on structural equation modeling. MIS Quarterly, 22(1), 7-16.
- Chin, W.W., Marcolin, B.L. & Newsted, P.R. (2003). A partial least squares latent variable modeling approach for measuring interaction effects: results from a Monte Carlo simulation study and an electronic-mail emotion/adoption study.Information Systems Research, 14(2), 189-217.
- Compeau, D. & Higgins, C. (1995). Computer self-efficacy: development of a measure and initial test. MIS Quarterly, 19(2), 189–211.
- ComScore. (2012). Web-based e-mail usage in Europe jumps 14 percent over past year. Retrieved from http://www.comscore.com/Insights/Press-Releases/2012/8/Web-Based-e-mail-Usage-in-Europe-Jumps-14-Percent-Over-Past-Year. (Archived by WebCite® at http://www.webcitation.org/6g5oxtI0T)
- ComScore. (2009). E-mail usage in Japan. Retrieved from http://www.comscore.com/Insights/Press-Releases/2009/1/e-mail-Usage-Japan. (Archived by WebCite® at http://www.webcitation.org/6g5p4xZKP)
- Conner, M. & Sparks, P. (2005). The theory of planned behaviour and health behaviour. In M. Conner & P. Norman (Eds.), Predicting health behaviour: research and practice with social cognition models (pp. 170-222). Maidenhead, UK: Open University Press.
- Courneya, K.S., Conner, M. & Rhodes, R.E. (2006). Effects of different measurement scales on the variability and predictive validity of the “two-component” model of the theory of planned behavior in the exercise domain. Psychology & Health, 21(5), 557-570.
- Davis, F.D. (1989). Perceived usefulness, perceived ease of use, and user acceptance of information technology. MIS Quarterly, 13(3), 319-340.
- Davis, F.D. (1993). User acceptance of information technology: system characteristics, user perceptions, and behavioural impacts. International Journal of Man-Machine Studies, 38(3), 475-487.
- Davis, F.D., Bagozzi, R.P. & Warshaw, P.R. (1989). User acceptance of computer technology: a comparison of two theoretical models. Management Science, 35(8), 982-1003.
- de Guinea, A.O. & Markus, M.L. (2009). Why break the habit of a lifetime? Rethinking the roles of intention, habit, and emotion in continuing information technology use. MIS Quarterly, 33(3), 433-444.
- Delone, W. H. & McLean, E. R. (2003). The DeLone and McLean model of information systems success: a ten-year update. Journal of management information systems, 19(4), 9-30.
- Deutsch, M. & Gerard, H. (1955). A study of normative and informational social influences upon individual judgment. Journal of Abnormal and Social Psychology, 51(3), 629-636.
- Dishaw, M.T. & Strong, D.M. (1999). Extending the technology acceptance model with task–technology fit constructs. Information & management, 36(1), 9-21.
- Fishbein, M. & Ajzen, I. (1975). Belief, attitude, intention, and behaviour: an introduction to theory and research. Reading, MA: Addison-Wesley Publishing.
- Gagnon, M.P., Godin, G., Gagné, C., Fortin, J.P., Lise, L., Daniel, R. & Alain, C. (2003). An adaptation of the theory of interpersonal behaviour to the study of telemedicine adoption by physicians. International Journal of Medical Informatics, 71(2-3), 103-115.
- Gefen, D. (2003). TAM or just plain habit: a look at experienced online shoppers. Journal of Organizational and End User Computing, 15(3), 1-13.
- Gefen, D. & Straub, D.W. (1997). Gender differences in the perception and use of e-mail: an extension to the technology acceptance model. MIS Quarterly, 21(4), 389-400.
- Gjoka, M., Kurant, M., Butts, C.T. & Markopoulou, A. (2009). A walk in Facebook: uniform sampling of users in online social networks. Retrieved from https://pdfs.semanticscholar.org/3a2c/e539f95ff889d196759b947eb993d9d17bdd.pdf?_ga=1.150345590.1754761156.1488164793. (Archived by WebCite® at http://www.webcitation.org/6obRZzbNa)
- Hagger, M.S. & Chatzisarantis, N.L.D. (2005). First‐and higher‐order models of attitudes, normative influence, and perceived behavioural control in the theory of planned behaviour. British Journal of Social Psychology, 44(4), 513-535.
- Hair, J.F., Hult, G.M., Ringle, C.M. & Sarstedt, M. (2014). A primer on partial least squares structural equation modeling (PLS-SEM). Thousand Oaks, CA.: Sage Publications, Inc.
- Hair, J.F., Ringle, C.M. & Sarstedt, M. (2011). PLS-SEM: indeed a silver bullet. The Journal of Marketing Theory and Practice, 19(2), 139-152.
- Hawkins, D.T. (2001). Information science abstracts: tracking the literature of information science. Part 1: definition and map. Journal of the American Society for Information Science and Technology, 52(1), 44-53.
- Henseler, J., Ringle, C.M. & Sinkovics, R.R. (2009). The use of partial least squares path modeling in international marketing. Advances in International Marketing, 20, 277-320.
- Hogarth, R. M. & Einhorn, H. J. (1992). Order effects in belief updating: the belief adjustment model. Cognitive Psychology, 24(1), 1-55.
- Hong, S.-J., Thong, J. Y. L. & Tam, K. Y. (2006). The effects of post-adoption beliefs on the expectation-confirmation model for information technology continuance. International Journal of Human – Computer Studies, 64(9), 799-810.
- Hsu, M.H. & Chiu, C.M. (2004). Predicting electronic service continuance with a decomposed theory of planned behaviour. Behaviour & Information Technology, 23(5), 359-373.
- Hu, P.J.H., Clark, T.H.K. & Ma, W.W. (2003). Examining technology acceptance by school teachers: a longitudinal study. Information & management, 41(2), 227-241.
- Hutchinson, C., Ward, J. & Castilon, K. (2009). Navigating the next-generation application architecture. IT Professional Magazine, 11(2), 18-22.
- Ifinedo, P. (2006). Acceptance and continuance intention of web-based learning technologies (WLT) use among university students in a Baltic country. The Electronic Journal on Information Systems in Developing Countries, 23(6), 1-20.
- Jarvis, CB, MacKenzie, S B. & Podsakoff, P.M. (2003). A critical review of construct indicators and measurement model misspecification in marketing and consumer research. Journal of Consumer Research, 30(2), 199-218.
- Kantar TNS. (2014). Facebook investigation of consumer online behaviour in Taiwan. Taipei: Kantar TNS. Retrieved from http://www.slideshare.net/yuanping/facebook-36279879 (Archived by WebCite® at http://www.webcitation.org/6bESANF3c)
- Karahanna, E., Straub, D. W. & Chervany, N. L. (1999). Information technology adoption across time: a cross-sectional comparison of pre-adoption and post-adoption beliefs. MIS Quarterly, 23(2), 183-213.
- Keil, M., Tan, B. C. Y., Wei, K. K., Saarinen, T., Tuunainen, V. & Wassenaar, A. (2000). A cross-cultural study on escalation of commitment behaviour in software projects. MIS Quarterly, 24(2), 299-325.
- Kehoe, C. M. & Pitkow, J. E. (1997). Surveying the territory: GVU’s five WWW user surveys. World Wide Web Journal, 1(3), 77-84. Retrieved from https://smartech.gatech.edu/bitstream/handle/1853/3534/97-14.pdf (Archived by WebCite® at http://www.webcitation.org/6bERUdsMq)
- Kim, B. (2010). An empirical investigation of mobile data service continuance: incorporating the theory of planned behaviour into the expectation–confirmation model. Expert Systems with Applications, 37(10), 7033-7039.
- Kim, B. (2011). Understanding antecedents of continuance intention in social-networking services. Cyberpsychology, Behaviour, and Social Networking, 14(4), 199-205.
- Kim, S.S. (2009). The integrative framework of technology use: an extension and test. MIS Quarterly, 33(3), 513-538.
- Kim, S.S. & Malhotra, N.K. (2005). A longitudinal model of continued IS use: an integrative view of four mechanisms underlying postadoption phenomena. Management Science, 51(5), 741-755.
- Kim, Y.J., Chun, J.U. & Song, J. (2009). Investigating the role of attitude in technology acceptance from an attitude strength perspective. International Journal of Information Management, 29(1), 67-77.
- Kim, H. W., Chan, H. C. & Chan, Y. P. (2007). A balanced thinking–feelings model of information systems continuance. International Journal of Human-Computer Studies, 65(6), 511-525.
- King, W.R. & He, J. (2006). A meta-analysis of the technology acceptance model. Information & Management, 43(6), 740-755.
- Ku, Y.C., Chen, R. & Zhang, H. (2013). Why do users continue using social networking sites: an exploratory study of members in the United States and Taiwan. Information & Management, 50(7), 571–581.
- Lee, Y., Kozar, K.A. & Larsen, K.R.T. (2003). The technology acceptance model: past, present, and future. Communications of the Association for Information Systems, 12, 752-780.
- Leskovec, J. & Faloutsos, C. (2006). Sampling from large graphs. In Proceedings of the 12th ACM SIGKDD International Cconference on Knowledge Discovery and Data Mining (pp. 631-636). New York, NY: ACM. Retrieved from http://www.stat.cmu.edu/~fienberg/Stat36-835/Leskovec-sampling-kdd06.pdf. (Archived by WebCite® at http://www.webcitation.org/6g5pGDI4t)
- Liao, C., Palvia, P. & Chen, J. L. (2009). Information technology adoption behaviour life cycle: toward a technology continuance theory (TCT). International Journal of Information Management, 29(4), 309-320.
- Limayem, M., & Cheung, C. M. (2008). Understanding information systems continuance: the case of Internet-based learning technologies. Information & management, 45(4), 227-232.
- Limayem, M., & Cheung, C. M. (2011). Predicting the continued use of Internet-based learning technologies: the role of habit. Behaviour & Information Technology, 30(1), 91-99.
- Limayem, M. & Hirt, S.G. (2003). Force of habit and information systems usage: theory and initial validation. Journal of the Association for Information Systems, 4(1), 65-95.
- Limayem, M., Hirt, S.G. & Cheung, C.M.K. (2007). How habit limits the predictive power of intention: the case of information systems continuance. MIS Quarterly, 31(4), 705-737.
- Limayem, M., Khalifa, M. & Chin, W. (2004). Factors motivating software piracy: a longitudinal study. IEEE Transactions on Engineering Management, 51(4), 414-425.
- Lippert, S.K. & Forman, H. (2005). Utilization of information technology: examining cognitive and experiential factors of post-adoption behavior. IEEE Transactions on Engineering Management, 52(3), 363-381.
- Lu, Y., Zhou, T. & Wang, B. (2009). Exploring Chinese users' acceptance of instant messaging using the theory of planned behavior, the technology acceptance model, and the flow theory. Computers in human behavior, 25(1), 29-39.
- Mäntymäki, M. & Salo, J. (2011). Teenagers in social virtual worlds: continuous use and purchasing behavior in Habbo Hotel. Computers in Human Behavior, 27(6), 2088-2097.
- Martins, C., Oliveira, T. & Popovič, A. (2014). Understanding the Internet banking adoption: a unified theory of acceptance and use of technology and perceived risk application. International Journal of Information Management, 34(1), 1-13.
- Mathieson, K. (1991). Predicting user intentions: comparing the technology acceptance model with the theory of planned behaviour. Information systems research, 2(3), 173-191.
- McWilliams, G. (2000). Building strong brands through online communities. MIT Sloan Management Review, 41(3), 43-54.
- Opitz, N., Langkau, T.F., Schmidt, N.H. & Kolbe, L.M. (2012). Technology acceptance of cloud computing: empirical evidence from German IT departments. In Proceedings of 45th Hawaii International Conference on System Science, Maui, HI, (pp. 1593-1602). Washington, DC: IEEE.
- Ouellette, J. A. & Wood, W. (1998). Habit and intention in everyday life: the multiple processes by which past behavior predicts future behavior. Psychological bulletin, 124(1), 54.
- Pahnila, S. & Warsta, J. (2010). Online shopping viewed from a habit and value perspective. Behaviour & Information Technology, 29(6), 621-632.
- Park, E. & Kim, K.J. (2014). An integrated adoption model of mobile cloud services: exploration of key determinants and extension of technology acceptance model. Telematics and Informatics, 31(3), 376-385.
- Pontiggia, A. & Virili, F. (2010). Network effects in technology acceptance: laboratory experimental evidence. International Journal of Information Management, 30(1), 68-77.
- Praveena, K. & Thomas, S. (2014). Continuance intention to use Facebook: a study of perceived enjoyment and TAM. Bonfring International Journal of Industrial Engineering and Management Science, 4(1), 24-29.
- Premkumar, G. & Bhattacherjee, H. (2008). Explaining information technology usage: a test of competing models. Omega, 36(1), 64-75.
- Reichheld, F. & Sasser, Jr. W.E. (1990). Zero defections: quality comes to services. Harvard Business Review, 68, 105-111. Retrieved from https://hbr.org/1990/09/zero-defections-quality-comes-to-services [Unable to archive.]
- Ringle, C.M., Wende, S. & Will, A. (2005). Smart PLS 2.0 M3. Bönningstedt, Germany: SmartPLS Gmbh. Retrieved from http://www.smartpls.com/ (Archived by WebCite® at http://www.webcitation.org/6bESNWAJx)
- Roca, J.C., Chiub, C.M. & Martinez, F.J. (2006). Understanding e-learning continuance intention: an extension of the TAM. Human-Computer Studies, 64(8), 683-696.
- Saqer, G. (2009, August 11). Survey: Yahoo! Mail is the most popular webmail in Jordan. [Web log post]. Retrieved from http://arabcrunch.com/2009/08/survey-yahoo-mail-is-the-most-popular-webmail-in-jordan.html. (Archived by WebCite® at http://www.webcitation.org/6g5ooaZv9)
- Saeed, K. A. & Abdinnour-Helm, S. (2008). Examining the effects of information system characteristics and perceived usefulness on post adoption usage of information systems. Information & Management, 45(6), 376-386.
- Shepperd, B. H., Hartwick, J. & Warshaw, P. R. (1988). The theory of reasoned action: a meta-analysis of past research with recommendations for modifications and future research. Journal of Consumer Research, 15(3), 325-343.
- Sirte, M. & Karahanna, E. (2006). The role of espoused national cultural values in technology acceptance. MIS Quarterly, 30(3), 679-704.
- Stantchev, V., Colomo-Palacios, R., Soto-Acosta, P. & Misra, S. (2014). Learning management systems and cloud file hosting services: a study on students' acceptance. Computers in Human Behavior, 31, 612-619.
- Sun, H. & Zhang, P. (2006). The role of moderating factors in user technology acceptance. International Journal of Human-Computer Studies, 64(2), 53-78.
- Sun, Y. & Jeyaraj, A. (2013). Information technology adoption and continuance: a longitudinal study of individuals' behavioral intentions. Information & Management, 50(7), 457-465.
- Taiwan Network Information Center. (2013). Internet usage survey in Taiwan. Taipei: Taiwan Network Information Center. Retrieved from http://www.twnic.net.tw/index2.php (Archived by WebCite® at http://www.webcitation.org/6oZxbPgfC)
- Taylor, S. & Todd, P.A. (1995). Understanding information technology usage: a test of competing models. Information Systems Research, 6(2), 144-188.
- Thompson, R. L., Higgins, C. A. & Howell J.M. (1991). Personal computing: toward a conceptual model of utilization. MIS Quarterly, 15(1), 125-143.
- Thompson, R.L., Higgins, C.A. & Howell, J.M. (1994). Influence of experience on personal computer utilization: testing a conceptual model. Journal of Management Information Systems, 11(1), 167-187.
- Thong, J. Y. L., Hong, S.-J. & Tam, K. Y. (2006). The effects of post-adoption beliefs on the expectation-confirmation model for information technology continuance. International Journal of Human – Computer Studies, 64(9), 799-810.
- Triandis, H.C. (1980). Values, attitudes, and interpersonal behaviour. Nebraska Symposium on Motivation, 27, 195-259.
- Tschabitscher, H. (2016, June 1). Top ten free e-mail services 2017. Lifewire. Retrieved from https://www.lifewire.com/top-free-email-services-1171481. (Archived by WebCite® at http://www.webcitation.org/6obQt6LxT )
- Van den Putte, B. (1991). 20 years of the theory of reasoned action of Fishbein and Ajzen: a meta-analysis. Unpublished manuscript, University of Amsterdam, Netherlands.
- Venkatesh, V. (2000). Determinants of perceived ease of use: integrating control, intrinsic motivation, and emotion into the technology acceptance model. Information System Research, 11(4), 342-365.
- Venkatesh, V. & Davis, F.D. (2000). A theoretical extension of the technology acceptance model: four longitudinal field studies. Management Science, 46(2), 186-204.
- Venkatesh, V., Morris, M., Davis, G. & Davis, F.D. (2003). User acceptance of information technology: toward a unified view. MIS Quarterly, 27(3), 425-478.
- Venkatesh, V., Thong, J.Y.L., Chan, F.K.Y., Hu, P.J.H. & Brown, S.A. (2011). Extending the two-stage information systems continuance model: incorporating UTAUT predictors and the role of context. Information Systems Journal, 21(6), 527-555.
- Venkatesh,V., Thong, J.Y.L. & Xu, X. (2012). Consumer acceptance and use of information technology: extending the unified theory of acceptance and use of technology. MIS Quarterly, 36(1), 157-178.
- Venkatesh, V., Speier, C. & Morris, M. G. (2002). User acceptance enablers in individual decision making about technology: toward an integrated model. Decision Sciences, 33(2), 297-316.
- Verplanken, B., Aarts, H., van Knippenberg, A. & Moonen, A. (1998). Habit versus planned behaviour: a field experiment. British Journal of Social Psychology, 37(1), 111-128.
- Wright, K.B. (2005). Researching internet-based populations: advantages and disadvantages of online survey research, online questionnaire authoring software packages, and web survey services. Journal of Computer-Mediated Communication, 10(3). Retrieved from http://onlinelibrary.wiley.com/doi/10.1111/j.1083-6101.2005.tb00259.x/full (Archived by WebCite® at http://www.webcitation.org/6bERzcaeM)
- Wu, M.C. & Kuo, F.Y. (2008). An empirical investigation of habitual usage and past usage on technology acceptance evaluations and continuance intention. ACM Sigmis Database, 39(4), 48-73.