Exploring reward mechanisms on social question and answer Websites: quantifying the interdependences among user activities.
Juan Chen, Yong Liu and Hongxiu Li.
Introduction. Previous studies suggest that the users of social question and answer Websites contribute their knowledge to a community in exchange for intrinsic rewards from other members, such as reciprocity and reputation. Thus, rewarding knowledge contributors is of great importance for maintaining the users of such Websites. However, there is a lack of knowledge on the reward mechanisms used by the sites. In addition, while previous studies have highlighted the importance of reciprocity in motivating knowledge sharing, little is known about how reciprocity takes place in a knowledge community, hence, this study addresses that research gap.
Method. This study seeks to explore reward mechanisms and quantify the conversion ratio based on studying the profiles of 33,974 observations from a Chinese social question and answer Website.
Analysis. The structural equation modelling technique was adopted to estimate the research model via the partial least squares approach.
Results. The results show that users who have more followers and publish more questions and articles, tend to answer more questions on social question and answer Websites. The interaction effect between the users’ question-asking activities and the appreciation they receive has a significant influence on user popularity.
Conclusions. Writing articles and providing answers generate appreciation from other community members. In addition, users are likely to follow individual users who not only asked interesting questions but who also provided high-quality answers, implying a like-minded effect.
Introduction
People nowadays increasingly seek and share knowledge in online knowledge communities, especially on social question and answer Websites like Quora and Zhihu. Social question and answer sites have become popular because they provide a convenient setting for people to contribute and access knowledge. For instance, Quora claimed 100 million monthly unique visitors at the end of March 2016 (D'Angelo, 2016). Despite the increasing popularity of social question and answer sites, a key challenge is how to engage users in knowledge creation and dissemination on such sites because most users seemingly never ask or answer a question.
The famous 90-9-1 principle states that in an online community, 90% of users only access the Websites and never contribute knowledge, 9% of them occasionally participate, while only 1% of the key users create the vast majority of the community content (Nielsen, 2006). In other words, most social question and answer site users are simply knowledge consumers and do not share any knowledge within the community, thus most knowledge created in a community comes from a very small percentage of users. Therefore, maintaining and enlarging the proportion of contributors is key to the survival and success of such sites – no one would visit a site that provides no answers. Previous studies have reported a number of initially active online communities which failed to retain their users and thereby turned into cyber ghost towns (Jin, Lee, and Cheung, 2010; Preece, 2001).
A key reason for demotivated knowledge sharing is related to the nature of knowledge as a public good in an online knowledge community (Cheung, Lee, and Lee, 2013; Wasko and Teigland, 2004). A user's consumption of knowledge on social question and answer sites is free and does not lead to that content being withdrawn or the users being excluded from accessing that knowledge, which meets the definition of public good (Samuelson, 1954). Wasko and Teigland (2004, p. 25) noted that ‘public good of knowledge that is available to anyone in the network, making it easy for individuals to free-ride on the efforts of others'. As a result, the public good nature of knowledge makes most members maximise their gains by consuming the available knowledge, rather than by making an effort to contribute knowledge. However, using social exchange theory offers a strategic instrument for analysing reciprocal knowledge sharing (c.f., Bock and Kim, 2001; Liao, 2008) and suggesting ways to involve more than the one percent.
The aim of this study is to examine the reward mechanism in a social question and answer context using the data collected from a popular Chinese social question and answer site. According to the social exchange theory, users are assumed to be self-interested in knowledge sharing – they assess the cost and rewards before they share knowledge in online communities (Cheung et al., 2013; Wasko and Faraj, 2005). The intangible rewards for knowledge contributors are of great importance in encouraging them to engage in the community (Wasko and Faraj, 2005). Studies have identified a list of personal benefits that motivate knowledge sharing, such as reputation, reciprocity, enjoyment in helping others and social interaction (Cheung et al., 2013; Liang, Liu and Wu, 2008). Arguably, a knowledge community should facilitate an effective conversion of its users' knowledge input into tangible benefits in order to elicit their future contributions. Even though a reward mechanism would seem to be important to any knowledge community, there is a lack of study and knowledge on this issue.
Furthermore, the users of an online knowledge sharing site can choose to perform different activities in their community, such as asking questions, following others and publishing answers and articles. However, there is a lack of understanding on the interdependence between the different activities, and their driving mechanisms. Social exchange and social capital theories indicate an effect whereby successful knowledge exchange leads to a more interpersonal relationship between two parties, and that the interpersonal relationships formed between those parties then results in them being more active in their community (c.f., Chiu, Hsu and Wang, 2006; Cropanzano and Mitchell, 2005; Liao, 2008). Nevertheless, the question of how to provide support to users so that they can establish more interpersonal relationships and increase user activity remains largely a mystery.
To address the above-mentioned research gap, we conducted an empirical study on the users of Zhihu, which is a popular Chinese social question and answer Website offering a rich collection of diverse knowledge. Similar to Quora, questions posted in Zhihu often demand deep reflection and intensive knowledge. It is common that question-askers in Zhihu receive lengthy but very sophisticated answers from other users. As of May 2016, Zhihu claimed over fifty million registered users, had twelve million daily active users and five billion page views per month on average (Li, 2016); the average time that users spent on Zhihu on a daily basis was thirty-three minutes. The site has accumulated ten million questions and thirty-four million answers and received thirty-five million up-votes (Li, 2016). It is worth noting that there are more answers than questions posted on the site. Due to the success of Zhihu, it would appear worthwhile determining the reward systems and the action mechanisms that the users find when they use the site.
In the study, a research framework was developed by integrating the actions of both knowledge contributors and receivers into the framework. Specifically, based on a valid sample of 33,974 users, the study quantifies the effect of the users' knowledge input, such as publishing questions, answers and articles, and in triggering the knowledge receivers' reward mechanisms, such as giving likes and following the contributors. The research contributes to the literature by investigating how social exchange would occur in a social question and answer site from a reward mechanism perspective and offers new insights into understanding social interaction in knowledge communities.
The remainder of the paper is structured as follows. First, we provide a review of the literature, then discuss the research framework. Thereafter, we present the research methodology and discuss the results. Lastly, we consider the implications of our findings and conclude the paper.
Theoretical background: social exchange theory
Social exchange theory is one of the most widely used theories in studying knowledge sharing (Liang et al., 2008; Liu, 2008). Derived from economic exchange theory, social exchange theory postulates that people participate in exchange activities if they perceive the reward exceeds its costs (Bock and Kim, 2001; Liao, 2008). A basic tenet of the theory is that, by following certain unspecified rules of exchange (Bock and Kim, 2001), relationships between parties ‘evolve over time into trusting, loyal, and mutual commitments' (Cropanzano and Mitchell, 2005, p. 875). Reciprocity rules and negotiated rules are two of the major rules that have been investigated in earlier studies.
Specifically, reciprocity rules assume reciprocity to be an interdependent exchange process, implying that a beneficial action from one person to others will lead to a favourable response from the others (Cropanzano and Mitchell, 2005). In addition, reciprocity represents a folk belief that people get what they deserve. Albeit reciprocity can be viewed as a social norm, people endorse reciprocity differently.
Negotiated rules imply that the parties involved in an exchange may negotiate the method of exchange to aim at a beneficial arrangement (Cook, Emerson and Gillmore, 1983; Cropanzano and Mitchell, 2005); for instance, people negotiate tasks and responsibilities in team work. Furthermore, based on the social exchange theory, the resources that are exchangeable can be symbolic, e.g., status and information; or they can be material in nature, e.g., money and goods, leading to economic and extrinsic or socio-emotional and intrinsic outcomes of exchange, e.g., the feeling of being valued or respected (Cropanzano and Mitchell, 2005). The socio-emotional outcomes of exchange often trigger feelings of being valued, trusted and personal obligation, thus leading to the formation of enduring social relationships (c.f., Cropanzano and Mitchell, 2005; Oh, Zhang and Park, 2016; Ye, Chen and Jin, 2006). In this regard, social exchange theory resonates strongly with theories of social capital such that reciprocal behaviour engenders trust and social capital (Herreros, 2004; Wang and Chiang, 2009).
Social exchange theory emphasises the continuance of exchange actions as a result of a party's willingness to return a favour (Wang and Chiang, 2009; Wayne, Shore and Liden, 1997). In this vein, asking for a favour from another party appears to be a good trigger for starting a circle of reciprocity of social exchange by indicating to another party the feeling that they are valued and appreciated (Rubin, 2010; Simmons, 2013). For instance, in a knowledge community like Zhihu, it is common that two users follow each other and answer each other's questions.
Social exchange theory postulates that exchange activities within an organisation or a community affect individual perceptions about the justice of the exchanges, which in turn affects the future performance of the individuals (Cropanzano, Prehar and Chen, 2002). Perceived justice derived from social exchange is said to positively affect the level of interpersonal trust between parties involved in an exchange (Aryee, Budhwar and Chen, 2002) while distributive justice reflects the perceived fairness of the exchange outcomes received. For instance, when one exchanges work for pay, the perceived fairness of the pay against the workload would affect the future work performance of the individual (c.f., Cropanzano, Prehar, and Chen, 2002). If the cost of an exchange exceeds the benefit or if there are no benefits, individuals may perceive the outcome to be unfair and are unlikely to repeat the action (Cropanzano, Prehar and Chen, 2002; Liao, 2008).
In a summary of twenty-nine studies, Liang et al. (2008) suggested that social exchange theory offers an important theoretical lens through which to understand knowledge sharing . Apparently, ‘sharing knowledge is not human nature, especially knowledge that people deem valuable' (Liao, 2008, p. 1884). Knowledge sharing can be regarded as an act driven by a self-interested analysis of the cost and benefit of the action (Liang et al., 2008). If knowledge contributors perceive that the extrinsic and intrinsic rewards of knowledge sharing do not justify the cost, they will stop knowledge sharing (Liao, 2008). In other words, in the knowledge sharing process, people seek a fair outcome of exchange through a process of maximizing the gains of their behaviour and by minimising the costs, such as time and effort.
Wasko and Faraj (2005, p. 39) noted that, ‘in order to contribute knowledge, individuals must think that their contribution to others will be worth the effort and that some new value will be created, with expectations of receiving some of that value for themselves'. The expectation of personal benefits motivates individuals to share knowledge with unacquainted others in an online environment (Constant, Sproull, and Kiesler, 1996; Wasko and Faraj, 2005). Previous studies have identified a list of these personal benefits: reputation (Chang and Chuang, 2011; Hung, Durcikova, Lai, and Lin, 2011; Wasko and Faraj, 2005), reciprocity (Chai, Das, and Rao, 2011; Chang and Chuang, 2011), interpersonal trust (Chang and Chuang, 2011; Chen and Hung, 2010) and social interaction (Chang and Chuang, 2011; Chiu, Hsu, and Wang, 2006). For instance, Liu and Jansen, (2013) found that reputation and reciprocity are important factors for influencing an individual's knowledge sharing. Hung, Durcikova, Lai and Lin (2011 p. 416) note that the ‘existence of a built-in reputation feedback mechanism is necessary to support knowledge sharing'.
In spite of the availability of the list of benefits from the literature, a key challenge is how knowledge sharing sites can assist knowledge contributors in receiving these benefits and make the knowledge exchange more sustainable. The above discussion indicates that social exchange theory might offer one of the best ways to understand the effort–reward mechanism in an online social question and answer context. Social exchange theory assumes that a mechanism to reward knowledge contributors for their effort of sharing knowledge is the key to sustaining a knowledge community and giving its contributors a justification for their continued contribution (Liang, Liu and Wu, 2008; Liao, 2008; Liu, 2008; Ma and Chan, 2014). Thus, we assume the existence of this reward mechanism in a knowledge sharing community and seek to explore the features of the mechanism. In line with previous studies, we postulate that the rewards of knowledge sharing can be symbolic, such as reputation or a sign of popularity in a community.
Specifically, we argue that, from the social exchange theory viewpoint, the cost–benefit analysis of individuals could also be considered a matter of investment and reward. Users invest their time and effort (the cost) in exchange for rewards like reputation and reciprocity. For knowledge contributors, it is important to facilitate an effective and smooth transition from their investment to perceivable output that produces rewards. If knowledge contributors can obtain a sense of being rewarded, they are more likely to engage in future knowledge sharing. In line with previous studies on knowledge sharing (for a review see Cheung et al., 2013), we argue that the effectiveness of the input–output reward mechanism is key to maintaining a knowledge community and ensuring it prospers.
Rewarding a knowledge contributor: number of followers
In modern social question and answer sites, it is worth noting that users are given opportunities to establish a followee–follower relationship, which lays an important basis for their future social exchange activities. Normally, when a user makes an important contribution that other members value, they would consider following the user. In this vein, the number of followers a contributor has commonly represents a sign of reputation in a community.
This function of the followee–follower relationship has been widely implemented in social question and answer sites. For instance, in Zhihu and Quora, the followers may see in their feeds that followees like an answer or comment on an answer, or the followees may follow a new question or request answers to their questions. In a similar way, in Yahoo Answers, the actions of the users being followed, like answers, questions, votes, best answer ratings and awards, are automatically indicated on the follower’s newsfeed (Kayes, Kourtellis, Quercia, Iamnitchi and Bonchi, 2015b).
Previous studies indicate that the number of followers might represent a measurable goal for knowledge contributors to share their knowledge. For instance, Gazan (2011) elaborated on the future directions of social question and answer research and recommended applying economic and game theory models to the social question and answer research context. Gazan (2011, p. 10) noted that ‘participation in SQA [social question and answer] sites can be modeled as a game, where the goal might be to attract friends and followers...'. In this light, an effort to identify behavioural patterns on social question and answer sites ‘would extend existing research threads, inform the design of any site reliant on user-generated content, and increase understanding of participant motivation and interactions’ (Gazan, 2011, p. 10).
In earlier studies, the number of followers has been widely regarded as a proxy of wealth and power in a community (Kayes, Kourtellis, Quercia, Iamnitchi and Bonchi, 2015a). Users with a high number of followers are more popular and central in a network (Kayes et al., 2015a; Kayes, Qian, Skvoretz, and Iamnitchi, 2012). These popular users tend to have a larger audience when they post a question, because their questions will automatically appear on a larger number of follower’s newsfeeds. As a result, users with a large amount of followers were found to receive significantly more responses when they posted a question on different social question and answer platforms (Burton, Tanner and Giraud-Carrier, 2012; Liu and Jansen, 2013; Morris, Teevan and Panovich, 2010; Paul, Hong and Chi, 2011). Burton, Tanner and Giraud-Carrier (2012) found that a questioner with more followers tends to receive significantly more responses and in a significantly shorter time than those with fewer followers. Arguably, users with a high number of followers tend to be located at the centre of their community, enjoy a high reputation, and are perceived to be more trustworthy by other members (c.f., Burton et al., 2012; Liu and Jansen, 2013; Morris et al., 2010; Paul et al., 2011). Based on the above discussions, this study employs the number of followers as a dependent variable measuring user popularity in the research framework.
Research model and hypotheses development
To model the input–output reward mechanism, a research framework that takes into account the actions of both knowledge providers and knowledge consumers has been proposed. Because users’ actions in a knowledge community are constrained by systems functions, an introduction to the availability of different knowledge input methods in Zhihu is necessary before discussing any specific hypotheses.
Like many other knowledge communities, Zhihu users have three main alternatives for contributing to their community, including i) posting questions, ii) publishing articles and iii) answering questions. An article in Zhihu is like a blog but focuses on expressing an opinion or a thought on a free topic in a very systematic manner. Through writing articles and answering questions, users share their knowledge with others. The level of a users’ education is also included in the framework.
There are three possible reward methods in Zhihu. Specifically, users can choose to i) give a like. ii) express thanks for articles (or do both), or iii) follow the author of an answer, a question or an article. Note that users are unable to express thanks to a question-asker in Zhihu, but it is possible to follow a question-asker by clicking on the asker’s profile. The number of followers represents a user’s popularity or power in a community.
Instead of just being followed, a user can choose to follow other users as well. This function allows a closer social interaction between a user and their followers in which a reciprocal relationship is facilitated. Users may invite specific others to answer their questions, even if they do not yet follow one another. A summary of the definitions of the key variables is provided in Table 1.
Types | Variables | Definition |
---|---|---|
Input variables | Question-asking | The number of questions posted by a user. |
Article-publishing | The number of articles published by a user. | |
Question-answering | The number of answers provided by a user. | |
Output variables | Appreciation: likes | The number of likes that a user received from publishing answers and articles, which is an indicator of appreciation that a user received from the community. |
Appreciation: thanks | The number of thanks that users received from publishing answers and articles, which is an indicator of appreciation that a user received from the community. | |
Number of followers | The number of followers a user has, which is an indicator of how popular a user is in the community. | |
Control variables | Number of people followed | The number of people a user followed. |
Education | The education level of a user |
Previous studies indicate that a good education might make people more knowledgeable in a particular field and thus more capable of answering certain types of questions, rendering them more active in a knowledge community. For instance, Chang and Wu (2013) found that level of education significantly impacts on the knowledge sharing intention. Alhalhouli, Hassan and Der (2014) and Chen and Cheng (2012) reported that education level exerts a positive impact on knowledge sharing attitude. In line with previous studies, it is reasonable to assume that people with a better education would have a more positive attitude toward knowledge sharing, and thus answer more questions. We hypothesise that:
H1. Education positively relates to the frequency of question-answering.
In Zhihu, a user’s activities, such as the posting of a question, will automatically appear in the feeds of his or her followers. As a result, users who follow a larger number of contributors are more likely to be notified of questions. This increases the possibility of users accessing questions that they are interested in and being more willing to write an answer compared with those users who only follow a small number of users. Thus, we propose that:
H2. The number of users followed positively relates to the frequency of question-answering.
Asking questions is an important user action in any knowledge community. In some knowledge communities like Zhihu, many users may have already answered a number of questions and been followed by others before they post their first question on the site, as we will show later in our data. For question askers who have followers, their questions will automatically be forwarded to the followers’ feeds. In this vein, reciprocity between question askers and followers may be triggered. Specifically, by contributing knowledge to a community, e.g., writing excellent answers to questions, users may attract more users, (including the question-askers), to follow them. When these users have questions, their followers may feel an obligation to answer their questions.
As many users have a mutual following relationship, receiving answers from a follower may motivate the user to answer the follower’s question in the future.
In line with social exchange theory, posting a question to ask for help from one’s followers initiates a reciprocal exchange process. By answering users’ questions, the followers may in return expect to invite the question-askers to answer their questions in the future. With the support of the feed function, users can easily receive answer requests from someone they follow – and who may also be their follower, thus enabling a reciprocal question-answering relationship. Based on the theory, a frequent exchange of knowledge between two parties would enable a stronger interpersonal relationship between the user and their followers, enhancing their mutual commitment to perform reciprocal knowledge sharing activities (c.f., Cropanzano and Mitchell, 2005).
It is worth noting that Zhihu supports question-askers inviting specific users who are not necessarily their followers, to answer their questions. This enables the start of a new reciprocal relationship with these users, as they may request that they also have the opportunity to answer the question put to those initially invited to answer it. In this vein, we argue that those who posted questions to Zhihu are more likely to engage in reciprocity by answering questions posted by others, such as their followers, thus increasing the amount of answers they provide. We hypothesise:
H3. The frequency of question-asking positively relates to the frequency of question-answering.
In social question and answer sites, good questions and the users who ask them are important resources. Good questions are important triggers for eliciting excellent answers. Meanwhile, users may be interested in those question-askers who ask relevant and good questions. People may also share similar interests so have related questions that they would like to ask. As a result, users may be motivated to follow an individual that shares a like-minded curiosity with them. It has been widely acknowledged among scholars that social networks tend toward homogeneity (Garshick and Bohme, 2011; Saparito, Elam and Brush, 2013). Online networking is similar to bird migration activities in that birds of a feather flock together, i.e., social networking users make connections with like-minded users (Falls, 2007). According to this tenet, continued exposure to a network should increase one’s likelihood of finding other users that resemble oneself. Posting a relevant question offers an opportunity for like-minded users, including question-askers, to connect. In other words, increased exposure to other users in a community provides an alternative for others to recognise the user, thus making them more likely to be followed.
Furthermore, based on social exchange theory, people participate in knowledge sharing in exchange for rewards, in which answering other questions appears to be a key method for gaining incentives, such as followers. As a result, interesting and relevant questions that one can answer become a kind of scarce resource especially in Zhihu, as active Zhihu users are seemingly more eager to answer questions than to post them (Li, 2016). Thus, to increase their chance of finding relevant questions to answer, special attention should be paid to those users who asked interesting questions, such as following them. It is possible that users choose to follow others in order to be among the first to access new questions. Note that being the first to answer a question may offer particular advantages, such as giving the answer high visibility to an audience and being the first to offer a particular perspective. Thus, we assume that:
H4a. The frequency of question-asking positively relates to the number of followers.
Nonetheless, there is a risk that users follow a question-asker who does not intend to be an active user and who may just ask one or two questions and disappear. Hence, it is possible that following non-active users will not help build a mutually beneficial relationship between users or lead to any reciprocal rewards in the future. In this vein, the level of up-votes, i.e. the number of likes that a question asker receives from their previous activities becomes a sign of a good question-asker, making them much more likely to remain on the site and ask questions in the future. We argue that question-askers who receive more appreciation from a community are more likely to be followed by other users, thus we hypothesise:
H4b. An interaction effect between the level of appreciation and the frequency of question-asking positively relates to the number of followers.
In Zhihu, users can write articles to express their unique opinion or thought. Features that allow article writing offer users a way to share their knowledge with no restriction on topics or time. This is different to answering a question as the content is limited to the topic of the question and the responder may be expected to provide a response in a timely manner. Posting an article in Zhihu is, to a large extent, like writing a blog, which is one of the most prominent ways online users express their own ideas and thoughts (c.f., Chen, 2012; Dalhues, 2014).
Compared with posting answers, article authors have more time to prepare their writing on a specific topic, which is normally relevant to their own interests or expertise, resulting in higher quality content. Publishing articles enhances the visibility of a user, so that the user may better be remembered as an expert in a specific field. As a result, they are more likely to be invited to answer questions relevant to their expertise.
For article authors, being regarded as a professional and able to answer questions relevant to their own topics may engender a feeling of achievement and of being valued. These positive feelings, together with the relevance of the question to their expertise, may motivate the user to answer the question. This suggests a positive relationship between publishing articles and posting answers. Based on the above discussion, we hypothesise that:
H5. The frequency of article-publishing positively relates to the frequency of question-answering.
When users publish articles or answers in Zhihu, other users are allowed to express their appreciation by giving their likes and thanks. Users who post more answers and articles are more likely to be exposed to other community members and are more likely to attract the attention of other users. This increases their likelihood of receiving likes and thanks from other users.
Furthermore, according to social exchange theory, people participate in knowledge sharing in exchange for rewards. Given that economic rewards are missing from online knowledge communities, intrinsic incentives, such as appreciation from knowledge-receivers, are therefore an important reward for their behaviour (c.f., Bock and Kim, 2001; Liao, 2008). Previous studies have indicated a connection between people’s knowledge sharing and rewards, e.g., the gaining of appreciation or respect (Bock and Kim, 2001; Liao, 2008). Thus, we hypothesise that:
H6a. The frequency of article-publishing positively relates to the level of appreciation received.
H6b. The frequency of question-answering positively relates to the level of appreciation received.
Appreciation reflects the number of likes and thanks that a user receives for publishing answers and articles. In social question and answer sites, users may be inspired by the answers provided by a user and thus become interested in following them. From a utilitarian perspective, users may want to follow a knowledgeable individual in order to access any further content they produce, such as that they place in their feeds. This is similar to the behaviour of following famous people on Twitter. Furthermore, it is logical for users to follow a specific individual if they appreciated their contribution.
The above thinking is in line with social exchange theory because expressing likes and thanks to a user may be considered a way of rewarding that user for the knowledge they shared. Specifically, knowledge contributors exchange their knowledge for the reward of likes granted by knowledge receivers; the knowledge receivers reward the knowledge sharing by expressing their thanks and likes. According to the social exchange theory, satisfied social exchange will motivate the development of a more interpersonal relationship between the parties, such as trusting, loyal and mutual commitments (Cropanzano and Mitchell, 2005). In this vein, a reciprocal relationship can be regarded as a natural outcome of knowledge exchange. For instance, by reading a highly sophisticated answer, a user may perceive the responder to be reliable and knowledgeable and will therefore be willing to develop a more interpersonal relationship regarding future knowledge sharing. Thus, we hypothesise:
H7. The level of appreciation received positively relates to the number of followers a knowledge contributor has.
Based on the above hypotheses and discussion, a research framework was structured as depicted in Figure 1.
Research method
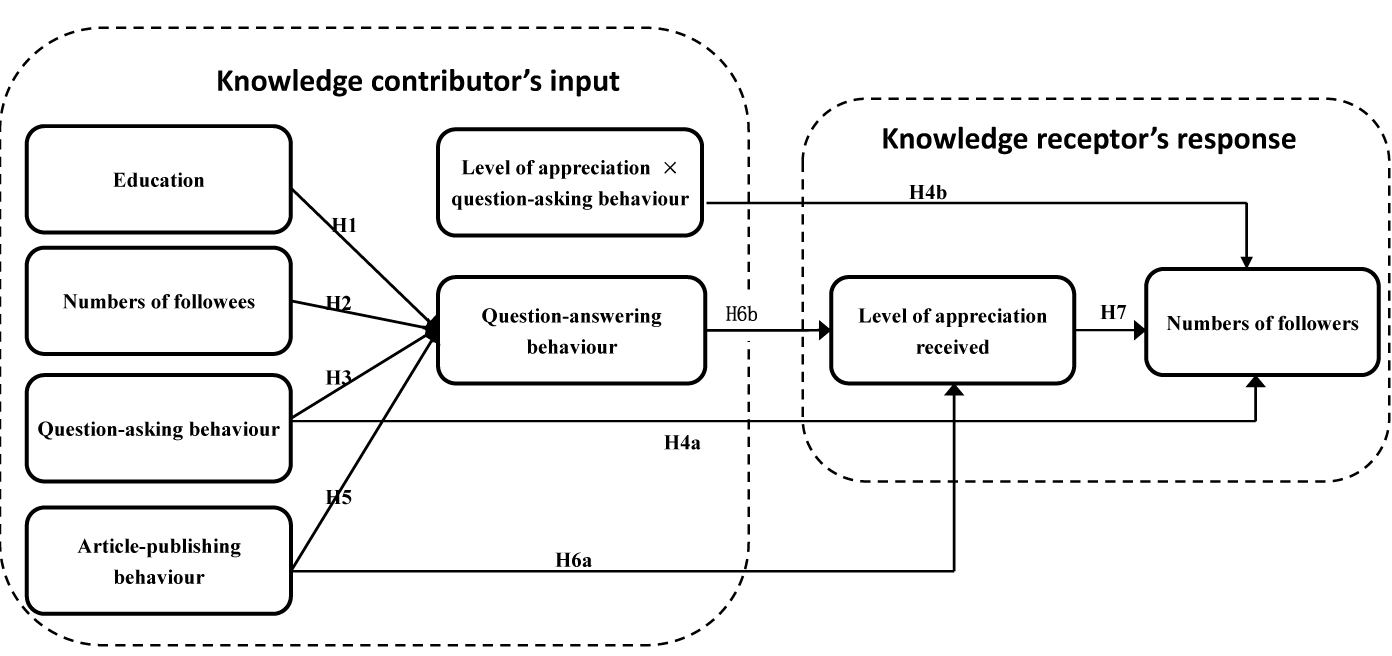
We collected research data from Zhihu by using LocoySpider, a Web-crawling program. Note that topics in Zhihu are divided into thirty-three different knowledge fields. We collected the profile information of the users who asked questions on chosen topics that are recognized by Zhihu, taken from six randomly selected knowledge fields, including cinema, art, culture, football, photography and chemistry.
Specifically, for each user profile, we extracted the demographic attributes and performance attributes of the user. The demographic attributes include each user’s identity, sex and education. User performance attributes cover the number of questions, answers, articles, collections, public editions, likes, thanks and followers. Users normally provide the school or university they graduated from to indicate their education level. By identifying the keywords (e.g., existence of keyword university degree points to a bachelor level of education), we classified user’s education level into six levels as a numeric measure, ranging from primary school [1] to doctorate [6]. By excluding observations with a missing value in their education levels, 33,974 valid profiles from registered users were retained for analysis. Among the users, 73.4% are male and 95.15% have a bachelor’s degree. As shown in Table 2, the average number of questions posted by each user is 4.22, while the average number of answers offered by each user is 54.6. This indicates that Zhihu users are more willing to answer a question than post one.
Items | Sample means | Standard deviation |
---|---|---|
Number of questions | 4.22 | 15.49 |
Number of answers | 54.60 | 153.87 |
Number of articles | 0.64 | 7.47 |
Number of likes | 1570.71 | 13039.02 |
Number of thanks | 354.23 | 2725.70 |
Number of users followed | 97.81 | 38.76 |
Number of followers | 831.22 | 9901.99 |
Education | 4.01 | 0.43 |
We plot the distribution of question-asking, question-answering and article-publishing in order to visualise the aim of those users who visited the site. As shown in Figure 2, there is an apparent discrimination in the behaviour of people visiting the site: some users may prefer to ask questions while some like publishing articles or answers.
Results of the measurement model
Based on Table 1, question-asking, question-answering , article-publishing, education and popularity (the number of followers) are adopted as formative variables, while appreciation is a reflective variable. A principal component analysis with Varimax rotation method was employed to estimate the convergent and discriminant validity of the proposed variables using the software R. The results show that all of the factor loadings are more than 0.5 (Straub, 1989), with a cumulative variance of 99%, as shown in Table 3. The indicators of appreciation or giving likes and expressing thanks, have very high factor loading values (factor loading > 0.94) on the same factor, which supports our use of the two items as reflective measurements. Each item had a higher loading on its corresponding factor than the cross-loadings of other factors, supporting both the convergent and discriminant validity of the proposed variables. Based on the use of SmartPLS 3.0, the Cronbach’s alpha, composite reliability and average variance extracted values were extracted, which are 0.955, 0.978 and 0.957, respectively. The three values are above their respective threshold values of 0.7, 0.8 and 0.5, suggesting good reliability (Fornell and Larcker, 1981; Hair, Hopkins, Georgia and College, 2008). The correlation matrix of the variables is provided in Table 4. All the above results support the validity of our measurement model.
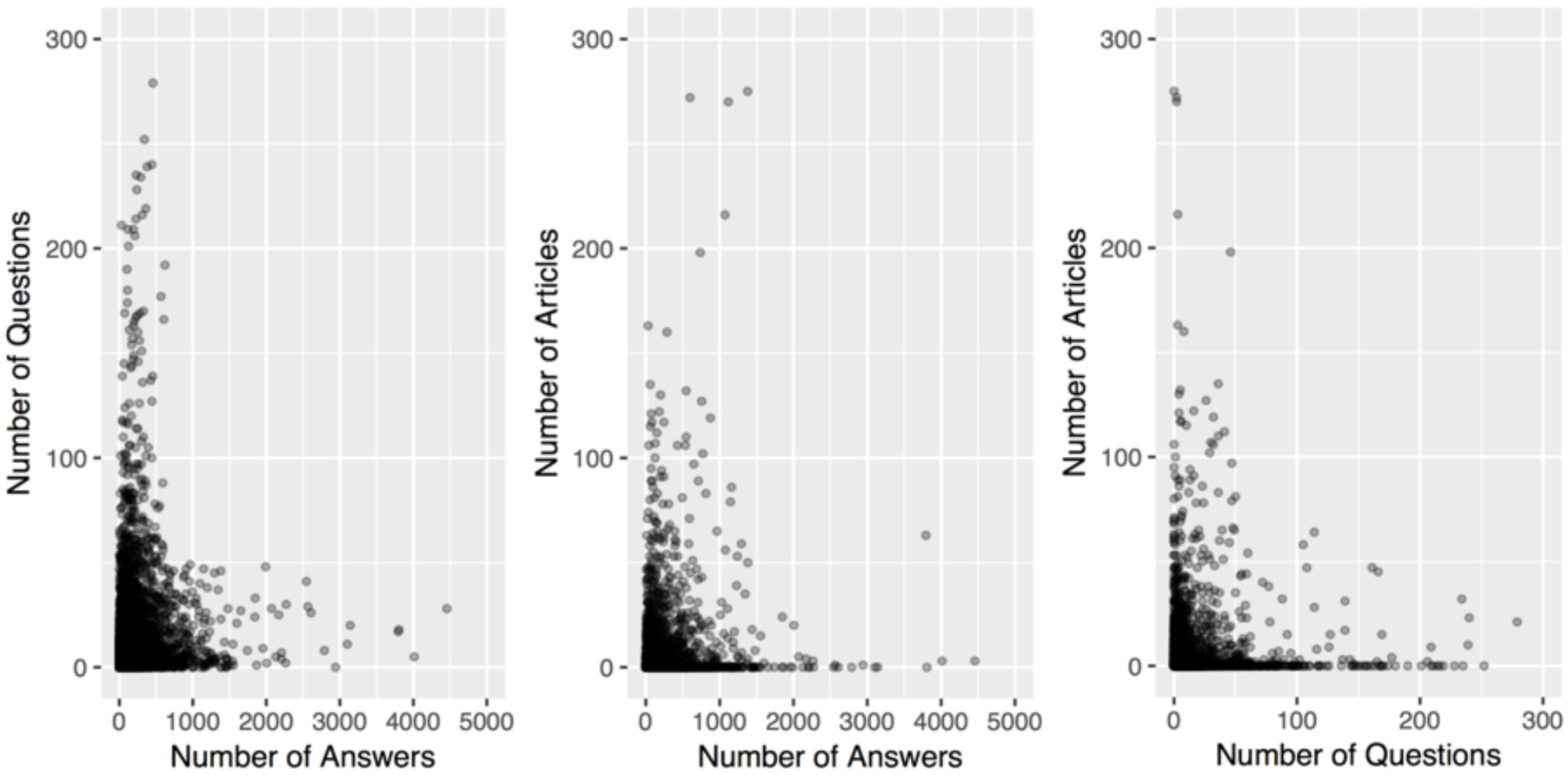
Figure 2: Plotting the relationships between three knowledge input activities.
Component | 1 | 2 | 3 | 4 | 5 | 6 | 7 |
---|---|---|---|---|---|---|---|
Education | 0.000 | 0.000 | 0.011 | 0.008 | 0.006 | 1.000 | -0.001 |
Number of questions | 0.031 | 0.041 | 0.990 | 0.077 | 0.095 | 0.011 | 0.043 |
Number of answers | 0.133 | 0.059 | 0.080 | 0.980 | 0.091 | 0.009 | 0.060 |
Number of articles | 0.162 | 0.979 | 0.042 | 0.059 | 0.033 | 0.000 | 0.090 |
Number of likes | 0.941 | 0.120 | 0.022 | 0.098 | 0.070 | 0.001 | 0.205 |
Number of thanks | 0.947 | 0.101 | 0.024 | 0.085 | 0.076 | 0.000 | 0.193 |
Number of followees | 0.103 | 0.032 | 0.097 | 0.089 | 0.985 | 0.007 | 0.024 |
Number of followers | 0.473 | 0.121 | 0.060 | 0.080 | 0.030 | -0.002 | 0.866 |
Variables | QA | AP | APB | Edu | FOLER | FOLEE |
---|---|---|---|---|---|---|
Question-answering (QA) | – | |||||
Appreciation (AP) | 0.247 | 0.978 | ||||
Article-publishing (AP) | 0.149 | 0.294 | – | |||
Education (Edu) | 0.018 | 0.002 | 0.001 | – | ||
Number of followers (FOLER) | 0.208 | 0.658 | 0.281 | -0.001 | – | |
Number of Followees (FOLEE) | 0.201 | 0.192 | 0.092 | 0.015 | 0.116 | – |
Question-asking (QAS) | 0.173 | 0.081 | 0.098 | 0.023 | 0.125 | 0.202 |
Note: Table 4 shows the correlation coefficients and the square root of the AVEs for latent variable Appreciation (bold on the diagonal). |
A structural equation modeling technique was adopted to estimate the research model by the partial least squares approach, for which SmartPLS 3.0 was employed. Previous studies have indicated that this approach excels in dealing with cases of non-normal data, small sample sizes as well as the formative variable in the model (Fornell and Bookstein, 1982; Dijkstra, 2010; Henseler, Ringle and Sinkovics, 2009; Peng and Lai, 2012; Hair, Sarstedt, Ringle and Mena, 2012). The approach is less restricted when analysing non-normal data because its algorithm transforms non-normal data via the central limit theorem (Hair et al., 2008). In the data, variables like questions and articles are non-normal distribution, which is left-censored at 0. Thus, the use of the partial least squares approach is appropriate for this study.
Results of the structural model
As shown in Table 5, almost all the hypotheses are supported, except for H4a. Specifically, the frequency of question-asking (β = 0.127, p < 0.001), the number of people followed (β = 0.164, p < 0.001) and education (β = 0.013, p < 0.05) significantly relate to the frequency of question-answering, therefore supporting hypotheses H1-3. The frequency of article-publishing significantly relates to the frequency of question-answering (β = 0.121, p < 0.001) and appreciation (β = 0.263, p < 0.001), respectively. Thus, hypotheses H5 and H6a are supported. The frequency of question-answering is found to significantly affect appreciation (β = 0.208, p < 0.001), which in turn significantly influences the number of followers (β = 0.523, p < 0.001). The results support hypotheses H6b and H7 as well. Consistent with our expectation, the interaction effect between question-asking and appreciation significantly influences popularity (β = 0.159, p < 0.001), thereby supporting hypothesis H4b. Figure 3 provides a visualisation of the results. Overall, the framework explains 7.3%, 12.9% and 49.3% of the variance of question-answering, appreciation and the number of followers, respectively.
Paths | Path coefficient | Findings |
---|---|---|
H1: Education → Question-answering | 0.013 * | Supported |
H2: Number of followees →Question-answering | 0.164 *** | Supported |
H3:Question-asking → Question-answering | 0.127 ** | Supported |
H4a:Question-asking →Popularity | not signif. | Not supported |
H4b:Question-asking →Appreciation →popularity | 0.159 *** | Supported |
H5:Article-publishing →Question-answering | 0.121 *** | Supported |
H6a: Article-publishing → Appreciation | 0.263 *** | Supported |
H6b: Question-answering → Appreciation | 0.208 *** | Supported |
H7: Appreciation → Popularity | 0.523 *** | Supported |
Note: *: P < 0.05; **: P < 0.01; ***: P < 0.001 |
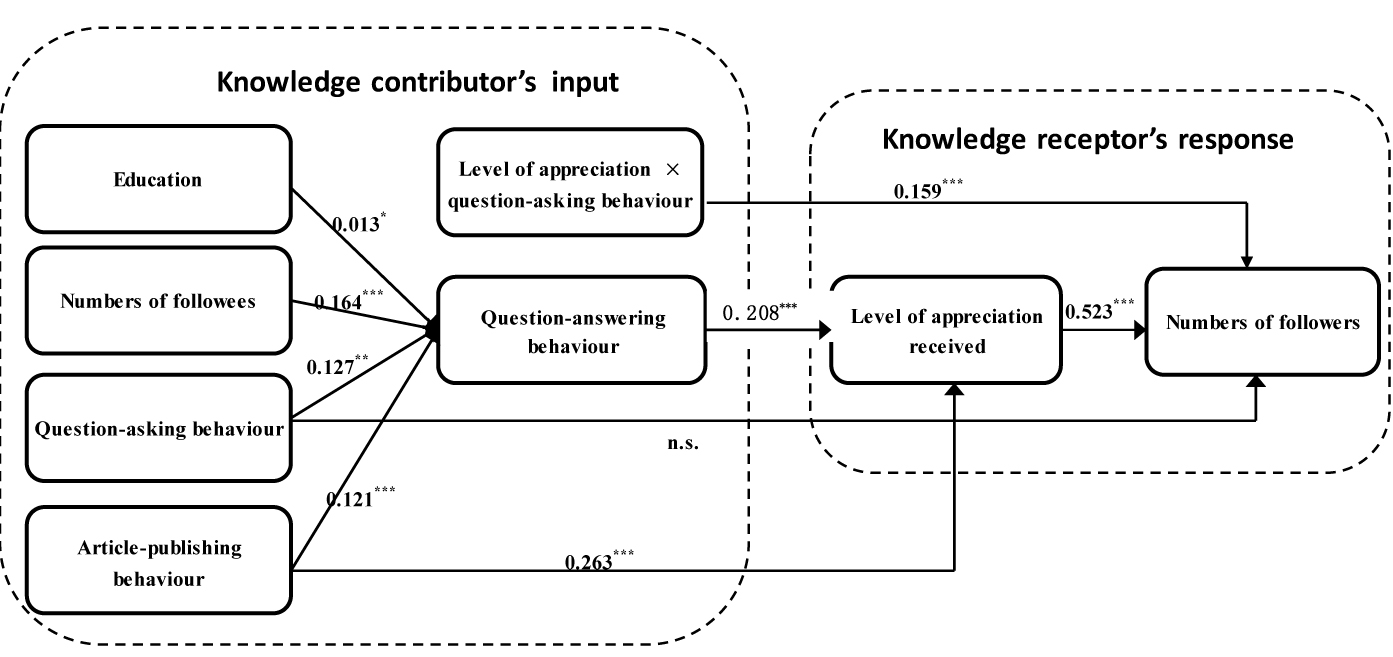
Figure 3: Results (*: p < 0.05; **: p < 0.01; ***: p < 0.001; n.s..: not significant)
Discussion and conclusion
Social exchange theory emphasises that knowledge contributors must be rewarded in order to retain them for future contributions, thus this research explores the details of how a reward system works in the context of a popular social question and answer website, Zhihu. The results offer a number of interesting findings.
First, we find that education exhibits a marginal effect on question-answering behaviour. In other words, on social question and answer sites, users who are more educated are only marginally more likely to answer questions than other members are. A possible explanation is that some questions posted on social question and answer may demand users reflect on their personal experience rather than education. Hence, higher education does not necessarily lead to a better capability to answer questions in Zhihu. This finding provides partial support for previous studies (Alhalhouli et al., 2014; Chen and Cheng, 2012).
Second, the results suggest that users who follow more people are more likely to answer questions. With regard to the feed function in Zhihu, users with more followees are, in fact, more likely to receive questions, enabling them to offer more answers. Psychologically, the intention to reciprocate a follower may play a role in motivating question-answering behaviour. Normally, users become the followers of a knowledge contributor by reading their articles or answers, the followed may therefore want to reciprocate the followee by answering their questions, an act which is in line with social exchange theory. Based on the analysis of the Zhihu users’ log data, this finding provides new support for previous studies on the positive effect of reciprocity regarding knowledge sharing (Chiu et al., 2006; Ye et al., 2006), especially as this is not a survey-based study unlike most of the previous studies on the issue.
Third, users who ask more questions also have the tendency to answer more questions. From the viewpoint of social exchange theory, when a user receives help from their community, they are more likely to return the favour and answer the questions asked by the community’s members. This reciprocal behaviour is supported by the fellowship mechanism in Zhihu. By answering each other’s questions, a stronger interpersonal relationship can be generated, resulting in a stronger interdependence between question-asking and question-answering of users.
Fourth, writing an article allows users a way to share their unique knowledge with others and also a channel for raising their reputation in the community. Publishing articles brings appreciation to authors while making their expertise more visible in a community. Meanwhile, by making their expertise better recognised by others, they are more likely to be invited to answer questions. Compared to answering questions, publishing articles is less restricted in terms of topic and time limit. Thus, it is more likely that the articles are of a higher quality than the answers given to questions. We observed a relatively high coefficient value regarding published article appreciation (β = 0.263, p< 0.001) when compared to that for answering questions (β = 0.208, p < 0.001), which supports the theory.
Fifth, obtaining other users’ appreciation is a key determinant regarding an individual being followed by others. The results indicated that, on average, for every answer and article posted, users would receive about 22.3 likes, and for every like, users would attract approximately 1.04 followers on Zhihu. In other words, if a user offers good answers to a question, it is very likely the user will be rewarded by being followed.
Sixth, in line with our expectation, the interaction effect between question-asking and appreciation significantly relates to the number of followers, albeit no direct effect from question-asking was found with regard to the number of followers. This suggests that contributing knowledge, e.g., answering questions, is not necessarily the only way to make a user more popular in a community, asking questions can also increase popularity. However, this effect only works for those who have already made a substantial contribution to their community, such as receiving a good number of likes and thanks. Given that many users may wait for interesting or challenging questions to answer, the act of offering questions is appreciated by community members, which attracts followers for the question-asker.
Implications for researchers
The results offer a number of useful insights for academia. Based on the social exchange theory, the study highlights the importance of converting the knowledge input of an online knowledge community’s users into the output gained from knowledge receivers. We hypothesised a reward mechanism and quantified the conversion rate in the context of Zhihu from the cost and reward perspectives of the theory. The proposed conversion mechanism provides a possible instrument for understanding the social interaction of user behaviour in a knowledge community.
Furthermore, our results reveal the existence of interdependences between different user behaviours in a knowledge community. We found that following other users and asking questions makes a user more likely to answer questions. In other words, following others and answering their questions may indicate the expectation that the followed should then reciprocate, or that the followed are obliged to the follower and should reward them in the future.
Third, our results highlight the importance of understanding question-asking in future studies of social question and answer sites. Question-asking not only triggers question-answering, but also increases the effect of appreciation, as seen by an increase in the number of followers. Given that most of the previous studies on social question and answer sites have focused on users who answer questions but not on askers of questions, we call for more research attention to be paid to this group. According to social exchange theory, question-asking triggers the start of reciprocal behaviour in a knowledge community and the successful exchange of knowledge motivates the development of more interpersonal relationships, thus leading to the sustainable development of the community.
Finally, the study contributes to new insights into social exchange theory. While most of the previous studies seek to validate social exchange theory by examining user perceptions, this study applies theory to validate the reward mechanism empirically by using a large amount of use log data collected from a popular knowledge community. Social exchange can be supported by different system functions in a more complex manner than we may expect, thus this study reports a few practical mechanisms for facilitating social exchange in knowledge-based communities. For instance, it is possible to reward article authors by treating them as experts because asking them questions may bring them a feeling of being recognised and valued. Also, asking people questions may indicate to users that their knowledge is being recognised and appreciated and, in certain contexts, that may be perceived as a reward. Our findings highlight the importance of question-asking in triggering the start of a reciprocal process that creates a future interpersonal relationship.
Implications for practitioners
The study offers insights for practitioners. First, we recommend knowledge community operators pay attention to how knowledge contributors are rewarded for their knowledge input and how the conversion rate of users being rewarded is quantified. This conversion rate may reflect the overall performance of a knowledge community. We argue that the higher the conversion rate, the more prosperous the community will be. If this conversion rate is low, users are likely to become less motivated to contribute their knowledge, further reducing the attractiveness of the knowledge community. Meanwhile, this conversion rate may offer a useful index for comparing the performances of different knowledge communities.
Furthermore, in addition to motivating users to answer questions, community operators should also motivate users to write articles and ask questions. Both actions may trigger social interaction between users, which forms a basis for establishing interpersonal relationships. By creating different opportunities for social interaction with others, the users may become more attached to a knowledge community and more willing to reciprocate.
To summarise, we call for more research attention on studying i) question-askers and ii) the reward mechanisms of social question and answer sites. We argue that the effectiveness of the reward mechanisms may determine the future of specific social question and answer sites, because question-askers are important resources for triggering increases in social exchange activities in a community. The social exchange theory provides a valid theoretical basis regarding how question-answering behaviour is triggered and converted into reputation. Hence, we used a research framework that could exploit a large empirical dataset. The results highlight the importance of understanding the interaction between knowledge contributors and knowledge receivers on social question and answer sites. Given the dearth of research on this issue, our study may serve as a useful reference point for future research. In addition, we call for more research effort into the behaviour of question-askers as well as the effect that being like-minded has on a knowledge community. We argue that question-askers are an important resource for social question and answer sites.
Acknowledgements
This work is part of the international cooperative project supported by the Fundamental Research Funds for the Central Universitiess through research grant number No.2662018PY095.
About the authors
Juan Chen is an associate professor of information management and information system at the college of public administration, Huazhong Agricultural University. Her research interests are in social media, user experience, and government network public opinion guidance. She can be contacted at chenjuan@mail.hzau.edu.cn
Yong Liu is an assistant professor of information system at Aalto University School of Business, Department of Information and Service Management, Finland. His research interests cover the areas of big data social science, electronic commerce, mobile commerce, social media and eWOM. He can be contacted at yong.liu@aalto.fi
Hongxiu Li is an assistant professor of business data analytics at the Department of Industrial and Information Management, Tampere University of Technology, Finland. Her research interests cover the areas of e-service, electronic commerce, social media, eWOM and big data. She can be contacted at Hongxiu.li@tut.fi
References
- Alhalhouli, Z.T., Hassan, Z.B.H. & Der, C.S. (2014). Factors affecting knowledge sharing behavior among stakeholders in Jordanian hospitals using social networks. International Journal of Computer and Information Technology, 3(5), 919–928.
- Aryee, S., Budhwar, P. S. & Chen, Z. X. (2002). Trust as a mediator of the relationship between organizational justice and work outcomes: test of a social exchange model. Journal of Organizational Behavior, 23(3), 267–285.
- Bock, G.-W. & Kim, Y.-G. (2001). Breaking the myths of rewards: an exploratory study of attitudes about knowledge sharing. Proceedings of Pacific Asia Conference on Information Systems (PACIS) (pp. 1112-1125). New York, NY: bepress.
- Burton, S. H., Tanner, K. W. & Giraud-Carrier, C. G. (2012). Leveraging social networks for anytime-anyplace health information. Network Modeling Analysis in Health Informatics and Bioinformatics, 1(4), 173–181.
- Chai, S., Das, S. & Rao, H. R. (2011). Factors affecting bloggers’ knowledge sharing: an investigation across gender. Journal of Management Information Systems, 28(3), 309–342.
- Chang, C.-C. & Wu, C.-C. (2013). Multilevel analysis of work context and social support climate in libraries. Aslib Proceedings, 65(6), 644–658.
- Chang, H. H. & Chuang, S. S. (2011). Social capital and individual motivations on knowledge sharing: participant involvement as a moderator. Information and Management, 48(1), 9–18.
- Chen, C. J. & Hung, S. W. (2010). To give or to receive? Factors influencing members’ knowledge sharing and community promotion in professional virtual communities. Information and Management, 47(4), 226–236.
- Chen, G. M. (2012). Why do women write personal blogs? Satisfying needs for self-disclosure and affiliation tell part of the story. Computers in Human Behavior, 28(1), 171–180.
- Chen, W. & Cheng, H. (2012). Factors affecting the knowledge sharing attitude of hotel service personnel. International Journal of Hospitality Management, 31(2), 468–476.
- Cheung, C. M. K., Lee, M. K. O. & Lee, Z. W. Y. (2013). Understanding the continuance intention of knowledge sharing in online communities of practice through the post-knowledge-sharing evaluation processes. Journal of the American Society for Information Science and Technology, 64(7), 1357–1374.
- Chiu, C.-M., Hsu, M.-H. & Wang, E. T. G. (2006). Understanding knowledge sharing in virtual communities: an integration of social capital and social cognitive theories. Decision Support Systems, 42(3), 1872–1888.
- Constant, D., Sproull, L. & Kiesler, S. (1996). The kindness of strangers: the usefulness of electronic weak ties for technical advice. Organization Science, 7(2), 119–135.
- Cook, K. S., Emerson, R. M. & Gillmore, M. R. (1983). The distribution of power in exchange networks: theory and experimental results. American Journal of Sociology, 89(2), 275–305.
- Cropanzano, R. & Mitchell, M. S. (2005). Social exchange theory: an interdisciplinary review. Journal of Management, 31(6), 874–900.
- Cropanzano, R., Prehar, C. & Chen, P. Y. (2002). Using social exchange theory to distinguish procedural from interactional justice. Group & Organization Management, 27(3), 324–351.
- D’Angelo, A. (2016). How many people use Quora? Retrieved from https://www.quora.com/How-many-people-use-Quora-7/answer/Adam-DAngelo?srid=Sy&share=c9a1688b (Archieved by WebCite®® at http://www.webcitation.org/6ywZRxKFH)
- Dalhues, K. (2014). Treehugging 2.0: powerful niche media in the battle for a better future? Hamburg, Germany: Anchor Academic Publishing.
- Dijkstra T. K. (2010). Latent variables and indices: Herman Wold’s basic design and partial least squares. In V. Esposito Vinzi, W. W. Chin, J. Henseler, H. Wang (Eds.), Handbook of partial least squares (pp. 23–46). Berlin: Springer-Verlag.
- Falls, J. (2007, November 19). What’s after Facebook or is the migration over. Social Media Explorer Retrieved from https://socialmediaexplorer.com/social-media-marketing/whats-after-facebook-or-is-the-migration-over/ (Archived by WebCite®® at: http://www.webcitation.org/6ywZjnKPj)
- Fornell, C. & Bookstein, F. L. (1982). Two structural equation models: LISREL and PLS applied to consumer exit-voice theory. Journal of Marketing Research, 19(4), 440–452.
- Fornell, C. & Larcker, D. (1981). Evaluating structural equation models with unobservable variables and measurement error. Journal of Marketing Research, 18(1), 39–50.
- Garshick, R. & Bohme, N. (2011). Integrated or isolated ? The impact of public housing redevelopment on social network homophily. Social Networks, 33(2), 152–165.
- Gazan, R. (2011). Social question and answer. Journal of the Association for Information Science and Technology, 62(12), 2301–2312.
- Hair, J. F., Hopkins, L., Georgia, M. & College, S. (2008). Partial least squares structural equation modeling (PLS-SEM). An emerging tool in business research. European Business Review, 26(2), 106–121.
- Hair, J. F., Sarstedt, M., Ringle, C. M. & Mena, J. A. (2012). An assessment of the use of partial least squares structural equation modeling in marketing research. Journal of the Academy of Marketing Science, 40(3), 414–433.
- Henseler, J., Ringle, C. M. & Sinkovics, R. R. (2009). The use of partial least squares path modeling in international marketing. Advances in International Marketing, 20(1), 277–319.
- Herreros, F. (2004). The problem of forming social capital: why trust? New York, NY: Palgrave Macmillan.
- Hung, S.-Y., Durcikova, A., Lai, H.-M. & Lin, W.-M. (2011). The influence of intrinsic and extrinsic motivation on individuals’ knowledge sharing behavior. International Journal of Human-Computer Studies, 69(6), 415–427.
- Jin, X.-L., Lee, M. K. O. & Cheung, C. M. K. (2010). Predicting continuance in online communities: model development and empirical test. Behavior & Information Technology, 29(4), 383–394.
- Kayes, I., Kourtellis, N., Quercia, D., Iamnitchi, A. & Bonchi, F. (2015a). Cultures in community question answering. In Yeliz Yesilada, Rosta Farzan, Geert-Jan Houben,(Eds.), Proceedings of the 26th ACM Conference on Hypertext & Social Media (pp. 175–184). New York, NY: ACM New York.
- Kayes, I., Kourtellis, N., Quercia, D., Iamnitchi, A. & Bonchi, F. (2015b). The social world of content abusers in community question answering. In Aldo Gangemi, Stefano Leonardi, Alessandro Panconesi, (Eds.), Proceedings of the 24th International Conference on World Wide Web (pp. 570–580). New York, NY: ACM
- Kayes, I., Qian, X., Skvoretz, J. & Iamnitchi, A. (2012). How influential are you: detecting influential bloggers in a blogging community. In Aberer, K., Flache, A., Jager, W., Liu L., Tang J., Gueret C., (Eds.), Proceedings of the 4th International Conference on Social Informatics, (pp. 29–42). Berlin: Springer-Verlag.
- Li, M. (2016). The fifth anniversary of Zhihu: tanglement before the explosive growth (In Chinese). ifanr.comRetrieved from http://www.ifanr.com/656966 (Archived by WebCite® at: http://www.webcitation.org/6ywZrMrDe)
- Liang, T-P., Liu, C-C. & Wu, C-H. (2008). Can social exchange theory explain individual knowledge sharing behavior? A meta analysis. In Proceedings of ICIS 2008 (18 pages). NewYork, NY: Curran Associates.
- Liao, L. F. (2008). Knowledge-sharing in R&D departments: a social power and social exchange theory perspective. International Journal of Human Resource Management, 19(10), 1881–1895.
- Liu, C.-C. (2008). Why individuals share their knowledge: extending social exchange theory with personal traits and task characteristics. In Proceedings of the 12th Pacific Asia Conference on Information Systems (pp. 1–13). New York, NY: bepress.
- Liu, Z. & Jansen, B. J. (2013). Factors influencing the response rate in social question and answering behavior. In Amy Bruckman, Scott Counts, Cliff Lampe & Loren Terveen, (Eds.), Proceedings of the 2013 conference on computer supported cooperative work (pp. 1263–1274). New York, NY: ACM
- Ma, W. W. K. & Chan, A. (2014). Knowledge sharing and social media: altruism, perceived online attachment motivation, and perceived online relationship commitment. Computers in Human Behavior, 39(1), 51–58.
- Morris, M. R., Teevan, J. & Panovich, K. (2010). A comparison of information seeking using search engines and social networks. In Marti Hearst, William Cohen & Samuel Gosling, (Eds.), Proceedings of the Fourth International Conference on Weblogs and Social Media (pp. 23–26).Menlo Park, CA: The AAAI Press
- Nielsen, J. (2006). The 90-9-1 rule for participation inequality in social media and online communities. Fremont, CA: Nielsen Norman Group. Retrieved from https://www.nngroup.com/articles/participation-inequality/ (Archived by WebCite® at: http://www.webcitation.org/6ywZxUvuz)
- Oh, S., Zhang, Y. & Park, M. S. (2016). Cancer information seeking in social question and answer services: identifying health-related topics in cancer questions on Yahoo! Answers. Information Research, 21(3), paper 718. Retrieved from http://www.informationr.net/ir/21-3/paper718.html (Archived by WebCite® at: http://www.webcitation.org/6ywa4iDgP)
- Paul, S., Hong, L. & Chi, E. H. (2011). Is Twitter a good place for asking questions? A characterization study. In Nicholas Nicolov, James G. Shanahan, Lada Adamic, Ricardo Baeza-Yates & Scott Counts, (Eds.), Proceedings of the Fifth International AAAI Conference on Weblogs and Social Media (pp. 578–581). Menlo Park, CA: AAAI Press
- Peng, D. X. & Lai, F. (2012). Using partial least squares in operations management research: apractical guideline and summary of past research. Journal of Operations Management, 30(6), 467–480.
- Preece, J. (2001). Sociability and usability in online communities: determining and measuring success. Behavior & Information Technology, 20(5), 347–356.
- Rubin, G. (2010, September 6). To make a friend, ask someone for a favor. Huffington Post Retrieved from https://www.huffingtonpost.com/gretchen-rubin/balanced-life-to-make-a-f_b_702655.html (Updated Dec 06, 2017) (Archived by WebCite® at: http://www.webcitation.org/6ywaFP5l3)
- Samuelson, P. A. (1954). The pure theory of public expenditure. The Review of Economics and Statistics, 36(4), 387–389.
- Saparito, P., Elam, A. & Brush, C. (2013). Bank–firm relationships: do perceptions vary by gender? Entrepreneurship Theory and Practice, 37(4), 837–858.
- Simmons, M. (2013, September 11). How asking for favors can build your relationships. Forbes Retrieved from https://www.forbes.com/sites/michaelsimmons/2013/09/11/how-asking-for-favors-can-build-your-relationships/#4ca370a35f71 (Archived by WebCite® at: http://www.webcitation.org/6ywaJZa3f)
- Straub D. (1989). Validating instruments in MIS research. MIS Quarterly, 13(2), 147–169.
- Wang, J. & Chiang, M. (2009). Social interaction and continuance intention in online auctions :a social capital perspective. Decision Support Systems, 47(4), 466–476.
- Wasko, M. M. & Faraj, S. (2005). Why should I share? examining social capital and knowledge contribution in electronic networks of practice. MIS Quarterly, 29(1), 35–57.
- Wasko, M. M. & Teigland, R. (2004). Public goods or virtual commons? Applying theories of public goods, social dilemmas, and collective action to electronic networks of practice. The Journal of Information Technology Theory and Application, 6(1), 25–41.
- Wayne, S. J., Shore, L. M. & Liden, R. C. (1997). Perceived organizational support and leader-member exchange: a social exchange perspective. The Academy of Management Journal, 40(1), 82–111.
- Ye, S., Chen, H. & Jin, X. (2006). Exploring the moderating effects of commitment and perceived value of knowledge in explaining knowledge contribution in virtual communities. In Proceedings of PACIS 2006 (pp.239-254). New York, NY: bepress.