Network relationships of U.S. government health agencies in relation to the Covid-19 pandemic
Seungil Yum
Introduction. Government institutes, such as the Centers for Disease Control and Prevention and the US Department of Health and Human Services, play important but different roles dealing with COVID-19 in the USA.
Method. This study used NodeXL between June 7 and June 8 to visualise the social networks of the Centers and the Department by collecting 5,166 (the Centers) and 2,940 tweets (the Department).
Analysis. Examined the characteristics of centrality of the two networks and used cluster analysis to identify communication groups.
Results. Findings confirmed that the Centers demonstrate characteristics of people-based online networks, whereas the Department appears as an institutes-based online networks. Second, offline social networks play an essential role in online social networks. Third, the Centers had a greater impact on the networks in the Department network than the effect the Department had on the Centers. Fourth, some key players, such as the World Health Organization, play a pivotal role in both networks.
Conclusions. Governments should explore the different types of health information of government institutes to effectively cope with the COVID-19 pandemic.
DOI: https://doi.org/10.47989/irpaper883
Introduction
Since 2019, coronavirus (SARS-CoV-2, COVID-19) has spread to all over the world, making this a priority for government policy and pandemic control (Bruns et al., 2020; Gruzd and Mai, 2020; Jiang et al., 2020; Mizumoto et al., 2020; Ndinojuo, 2020; Sanders et al., 2020; Singhal, 2020; Sohrabi et al., 2020; Yum, 2020). There were different approaches by governments (Arentz et al., 2020; Clerkin et al., 2020; Fang et al., 2020; Salehi et al., 2020; Wu and McGoogan, 2020). Many countries increased the scale of response in March 2020 (Hale et al., 2020).
This study explores the information role of the Centers for Disease Control and Prevention (hereafter, the Centers) and the Department of Health and Human Services (hereafter, the Department of Health) in the USA, using social network analysis based on Twitter, which was one of the most popular online network services in early 2020 (Khan et al., 2021).
Literature review
COVID-19 is an infectious disease, and the most common symptoms of COVID-19 are fever, dry cough, anosmia, and tiredness, but symptoms vary in severity, and asymptomatic people with COVID-19 can infect their close contacts (Yu and Yang, 2020). Comprehensive studies on transmission from asymptomatic patients are difficult to conduct, as they require testing of large population cohorts and more data are needed to better understand and quantify the transmissibility of COVID-19 (World Health Organization, 2020a).
The World Health Organization plays a leading role in guidelines and responses for COVID-19 and published a comprehensive package of guidance documents for countries, covering topics related to the management of COVID-19 on 10 January 2020. The Organization declared the novel coronavirus outbreak a public health emergency of international concern with the highest level of alarm on 30 January 2020. The Organization issued a consolidated package of existing guidance covering the preparedness, readiness and response actions for four different transmission scenarios: no cases, sporadic cases, clusters of cases and community transmission on March 7 (World Health Organization, 2020b). Table 1 outlines the roles of national and international organizations in response to the pandemic.
Dimension | Region | Name | COVID-19 relevant information facet |
---|---|---|---|
International | World | World Health Organization | The leading organization involved in the global coordination for mitigating the COVID-19 pandemic |
Europe | European Centre for Disease Prevention and Control | Provides the weekly surveillance report on COVID-19 in the EU/EEA and the UK using the available data compiled from multiple sources. | |
Africa | Africa Centres for Disease Control and Prevention | Strengthens the capacity and capability of Africa's public health institutions as well as partnerships to detect and respond quickly and effectively to COVID-19. | |
National | Canada | Public Health Agency of Canada | Releases total cases, active cases, recovered cases, number of people tested or deaths in Canada over time. |
The UK | Public Health England | Publishes national COVID-19 surveillance reports, including weekly summary of findings monitored through various COVID-19 surveillance systems. | |
The USA | Centers for Disease Control and Prevention | Conduct critical science and provides health information that protects the USA against COVID-19. For example, the Centers provide the COVID Data Tracker for visualizing cases, deaths, and trends of COVID-19 in the U.S. | |
United States Department of Health and Human Services | Works with state, local, tribal and territorial governments, public health officials, health care providers, researchers, private sector organizations and the public to execute a whole-of-America response to the COVID-19 pandemic to protect the health and safety of the American people. |
In the USA, the Centers and the Department of Health play an essential role in controlling the COVID-19 pandemic and are also connected with each other to cope with the COVID-19 pandemic. The Centers are a scientific health protection agency to protect people from health, safety and security threats. Its main goal is to protect public health and safety through the control and prevention of disease, injury, and disability in the USA and internationally. It especially focuses its attention on infectious disease, food-borne pathogens, environmental health, occupational safety and health, health promotion, injury prevention and educational activities designed to improve the health of United States citizens. The Centers also conduct research and provide information on the COVID Data Tracker for visualising cases, deaths, and trends of COVID-19 in the USA.
The Department of Health is a cabinet-level executive branch department of the US federal government with the goal of protecting the health of people and providing essential human services. Its mission is improving the health, safety, and well-being of people. The Department of Health works with state, local, tribal and territorial governments, public health officials, health care providers, researchers, private sector organizations and the public to execute a whole-of-America response to the COVID-19 pandemic. Both organizations work with many other organizations but the nature of their social networks for this pandemic response has not been investigated.
Research method
This study employed social network analysis to examine the informational role of the Centers andthe Department of Health for COVID-19. This is an interdisciplinary method developed mainly by sociologists to show the importance of relationships, such as multiplexity, centrality, mutuality, or reciprocity, among interacting units, such as individuals, groups, organizations, or societies (Cantner and Graf, 2006; see also Aggarwal, 2011; Bruggeman and Bruggeman, 2008; Butts, 2008; Durland and Fredericks, 2005; Scott, 1988; Van der Hulst, 2009; Zaphiris and Pfeil, 2007 for the basics of social network analysis). Social network analysis has been applied in information research (du Preez and Meyer, 2016; Johnson, 2019; McBirnie and Urquhart, 2011 (who employ network analysis to highlight the form of interaction patterns in serendipity); McKenzie, 2005; Mowbray et al., 2017; Munoz-Munoz and Miron-Valdivieso, 2017; Park and Park, 2016).
This study used Twitter data to demonstrate social networks of people for COVID-19 across public key players, such as institutes, politicians, media and organizations. Twitter has been widely employed for library and information science research (Hicks et al., 2020; Kjellberg et al., 2016; Stvilia and Gibradze, 2014; Sullivan, 2019; Torres-Salinas et al., 2011). Ewbank (2015) employs a social media analysis of #savelibraries and #getESEAright to explore library advocacy through Twitter.
This study employed NodeXL to examine social networks of the Centers and the Department of Health for COVID-19. NodeXL is a visualisation software program, which supports social networks and content analysis. NodeXL allows scholars to collect, analyse, and visualise a variety of networks containing the elements of a graph structure, such as edges and nodes (Bonsignore et al., 2009). NodeXL provides various visualisation tools and graph drawing layout algorithms, such as Fruchterman-Reingold and Harel-Koren. NodeXL can be used for collecting data from a variety of social media platforms, such as Twitter, YouTube, Flickr, and MediaWiki. NodeXL has been extensively employed as a social network method in various academic fields (Kim et al., 2013).
This study identified the top twenty Twitter accounts for the period between June 3 and June 10, 2020 based on the keyword COVID-19 and the Twitter accounts of the Centers and the Department of Health. NodeXL allows scholars to access Twitter for COVID-19 since 2019 regardless of the research period. The selected data set for the analyses (June 7 and June 8) was based on the number of Twitter users, communications, and suitable content. This is because there would be some differences for Twitter data between June 3 and June 10, 2020 since some users upload, delete, retweet, mention, or reply to COVID-19 during the period. For example, this study chose 10,010 users rather than 10,000 users (when other conditions are the same) for the best result, although it is a trivial change. In-degree centrality was used as an indicator of the main near neighbors to the Centers or the Department of Health (see Appendix Table 1 for descriptive statistics).
In-degree centrality, out-degree centrality, and betweenness centrality of the the Centers and the Department of Health networks were identified. In graph theory and network analysis, centrality refers to the positions of individual vertices within the network. Degree centrality is defined as the number of links incident upon a node (i.e., the number of ties that a node has). If the network is directed (i.e. ties have direction), then there are two separate measures of degree centrality, in-degree and out-degree (Hansen et al., 2020) (Figure 1).
Betweenness centrality shows how many times a vertex is located on the shortest path between two other vertices. The higher a user’s betweenness centrality, the more important they are for transmitting information through a network (Hansen et al., 2020) (see Figure 1). The three methods are calculated as follows:
(A) In-degree centrality: Cii=Σj;j ≠iyj,i
(B) Out-degree centrality: C0i=Σj;j ≠iyj,i
(C) Betweenness centrality: Cbi=Σj<kbj,k (i)/bj,k
Where Ci is the centrality of node i, bj,k = the number of the shortest links that pass through the node between j and k, and bj,k (i) = the number of the shortest links that pass through the node between j and k going through i. Directed graphs use the convention of connecting nodes with arrows that have arrow heads, showing who is directing the tie towards whom (Hanneman and Riddle, 2005).
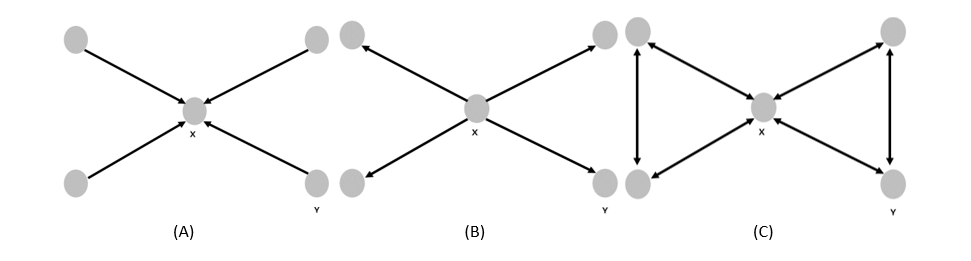
A: In-degree, B: Out-degree, C: Betweenness.
Next, this study employed cluster analysis by using the Clauset-Newman-Moore cluster algorithm (see Figures 5 and 6). Cluster analysis is a multivariate statistical methodology that explores data sets and identifies them into relatively homogeneous clusters that between-group variation is maximised and within-group variation is minimized. Each group should have objects that have common characteristics in the group and are as different from those in other groups as possible (Aldenderfer and Blashfield 1984; Everitt et al. 2001; Pálsdóttir, 2008). It is the main task in exploratory data mining and a common technique for statistical data analysis in many fields including social network analysis. Cluster analysis is also widely utilised for information research (Kim, 2010; Nelissen et al., 2017; Ortega Egea et al., 2007; Pálsdóttir, 2008; Sundling, 2017). For example, Ortega Egea et al. (2007) employ cluster analysis to distinguish the different groups of European Internet users. Kim (2010) uses cluster analyses to explore the develop a framework of information requirements for scientists based on 137 respondents. The Clauset-Newman-Moore cluster algorithm is one of the best methods for big data analysis (Vieira et al., 2014). This is because other traditional algorithms, such as the Kernighan–Lin algorithm, spectral partitioning, or hierarchical clustering, work well for some specific cases, but perform poorly in more general types. In contrast, the Clauset-Newman-Moore cluster algorithm is considerably faster than most well-known algorithms and allows scholars to explore huge social networks (Clauset et al., 2004). The equation is as follows:

Where Q is the modularity, 1/2m ∑vw[Avw is the number of edges in the graph, v and w are nodes, Kv is the degree of a node v, δ function δ(i, j) is 1 if i = j and 0 otherwise, and C is the community.
Results
In Figures 2-6, graphs represent networks of communication for COVID-19 between the Centers and the Department of Health according to in-degree centrality, out-degree centrality, and betweenness centrality, and the Clauset-Newman-Moore cluster algorithm. The graphs are made up of vertices and edges. Figures 2-4 show that the social networks of the Centers and the Department of Health are differentiated by centrality types. In this study, a circle represents the vertex that is each Twitter user. The labels of the top twenty Twitter accounts are identified in Table 3 for the Centers and Table 4 for the Department of Health. A line represents the edge that is a communication network extending from one Twitter user to another Twitter user. Each communication has two or more tweets to which it is attached, called its endpoints. Twitter users in the inner circle tend to play a more important role in social networks more than those in the outside circle. In-degree centrality in Figure 2 describes the number of communication networks that others have initiated with a Twitter user. For instance, if the Centers were mentioned 100 times by other Twitter users, its in-degree centrality would be 100. Out-degree centrality in Figure 3 visualises the number of communication networks that a Twitter user has initiated with others. If the Department of Health mentioned 100 other users in its tweet, its out-degree centrality would be 100. Betweenness centrality in Figure 4 shows how many times a Twitter user is placed on the shortest path between other Twitter users. For example, the more people depend on the Centers to make social networks with other Twitter users, the higher the value of the Centers' betweenness centrality.
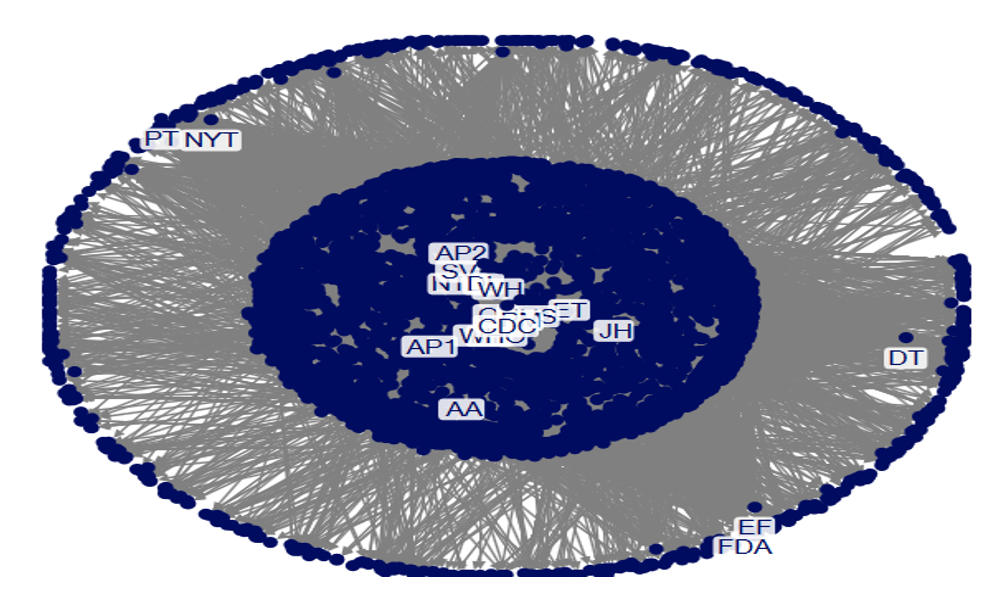
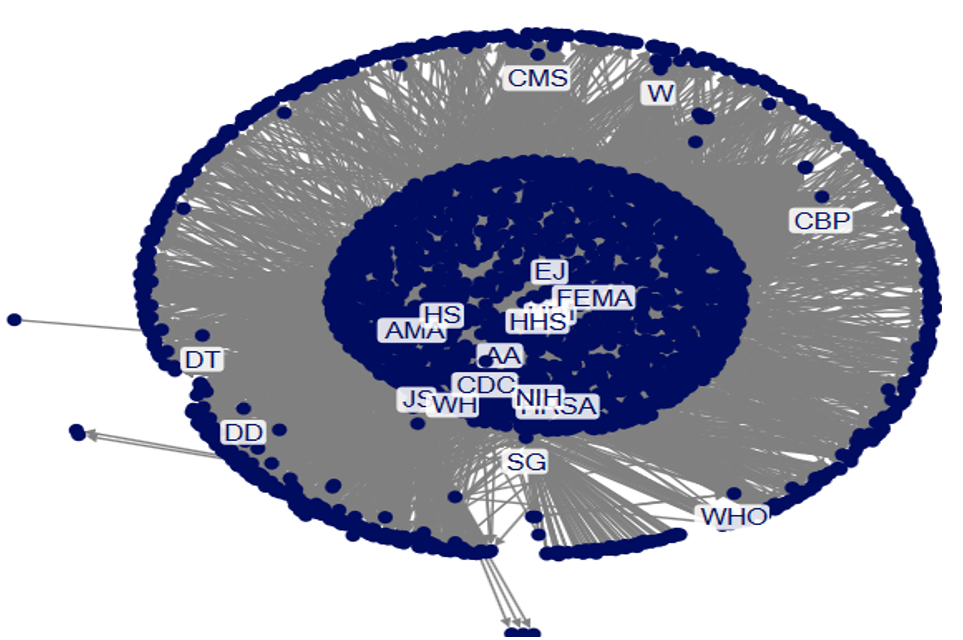
Figure 2: Social networks based on in-degree centrality (top: the Centers and bottom: the Department of Health)
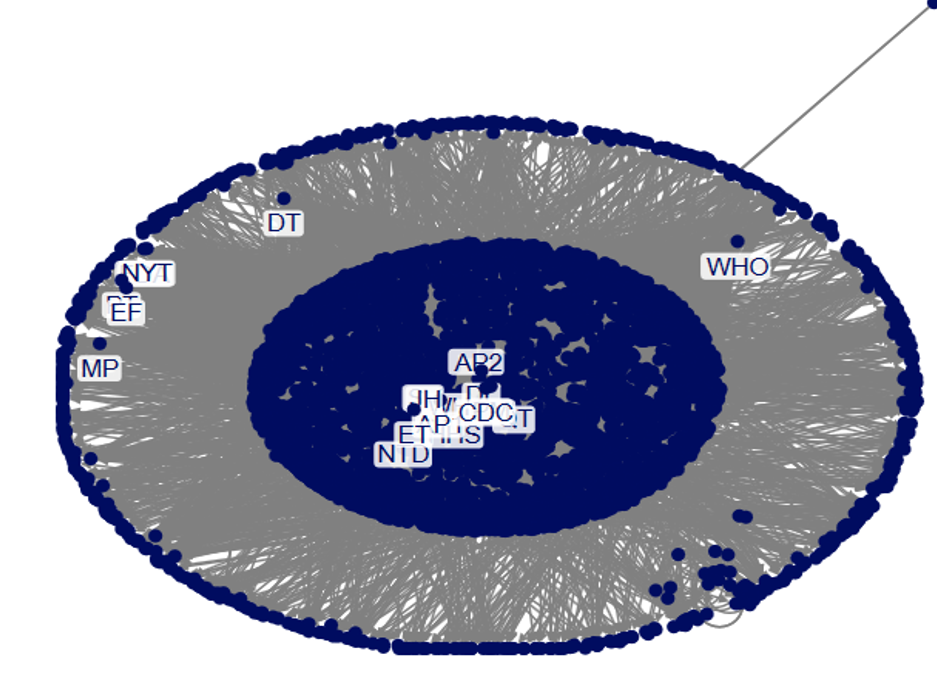
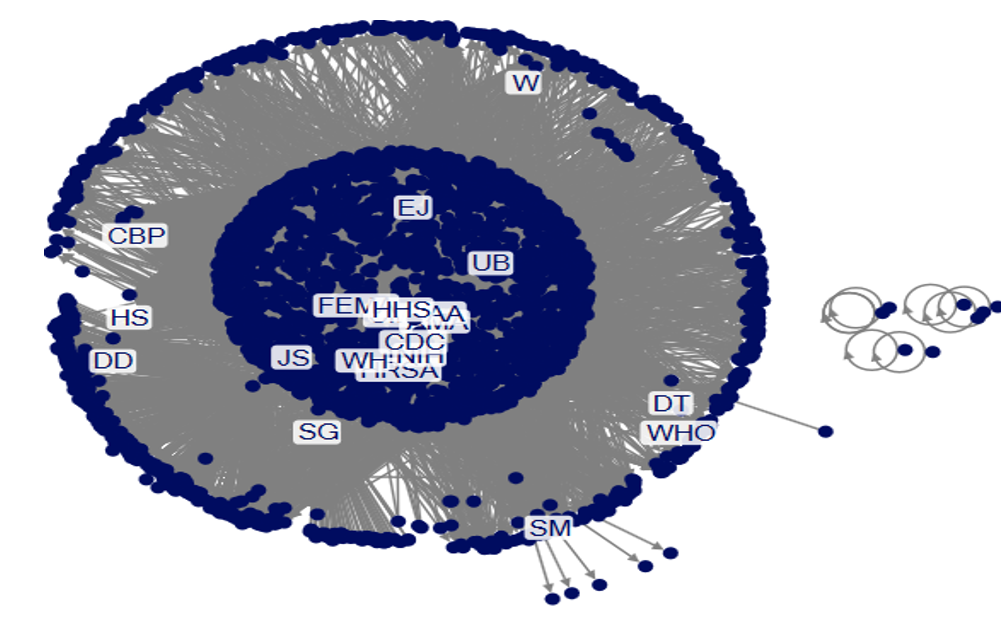
Figure 3: Social networks based on out-degree centrality (top: the Centers and bottom: the Department of Health)
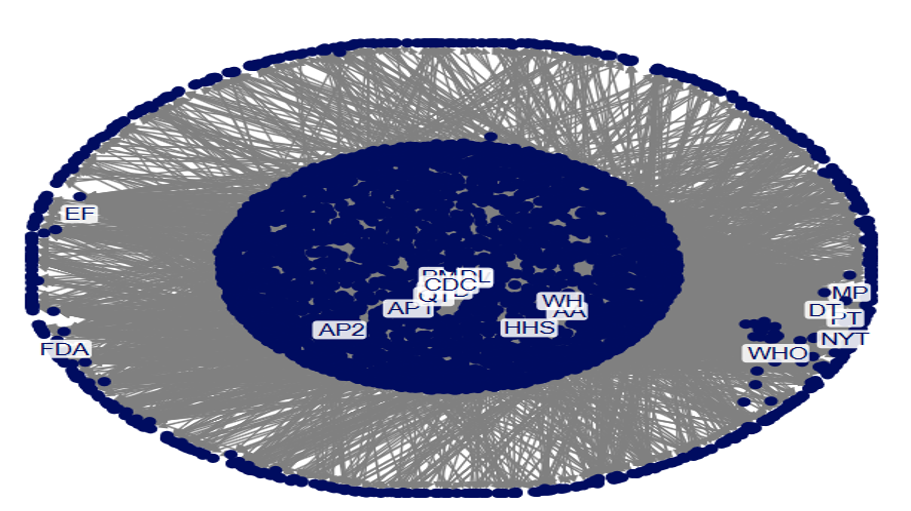
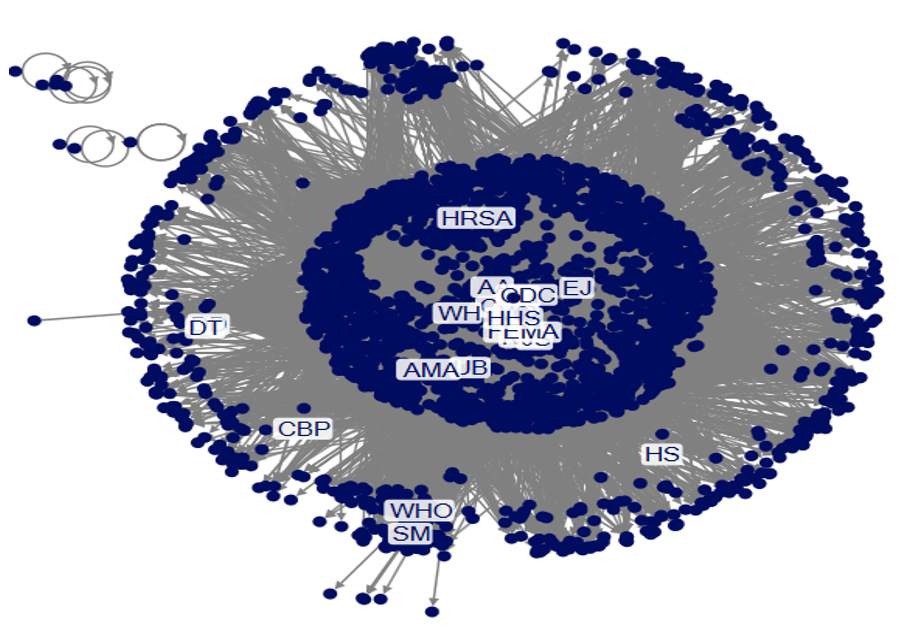
Figure 4. Social networks based on betweenness centrality (top: the Centers and bottom: the Department of Health)
Figure 5 highlights the social networks of the Centers and the Department of Health based on the Clauset-Newman-Moore cluster algorithm by laying out the graph in the entire graph pane. The algorithm tries to place collections of densely connected Twitter users into separate groups. The same color Twitter users represent that they belong to the same group created by the Clauset-Newman-Moore cluster algorithm. Each Twitter user is assigned to exactly one group with the same color. The number of Twitter user and communications in each group is highly differentiated by the characteristics of groups. In the Centers networks, the Centers are in the central part of the whole networks, and most key players are concentrated in the inner circle. Some key players, such as Donald Trump (DT), The New York Times (NYT), and US FDA (FDA), are placed in the right side of the inner circle. In the Department of Health networks, while the Department of Health is placed in the central part of the inner circle, the key players show more dispersed patterns. For instance, six key players are placed between the inner circle and the outer circle, and they are all around the inner circle.
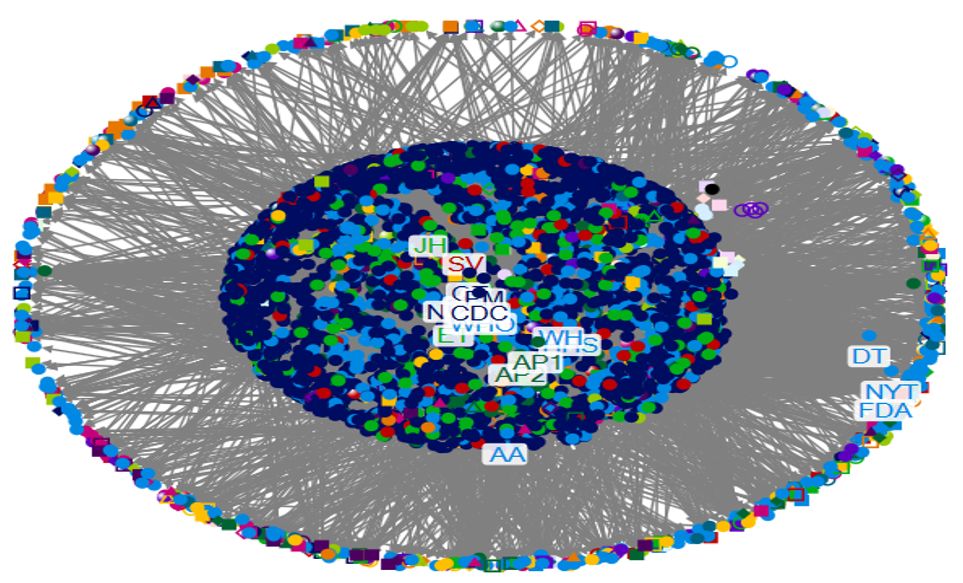
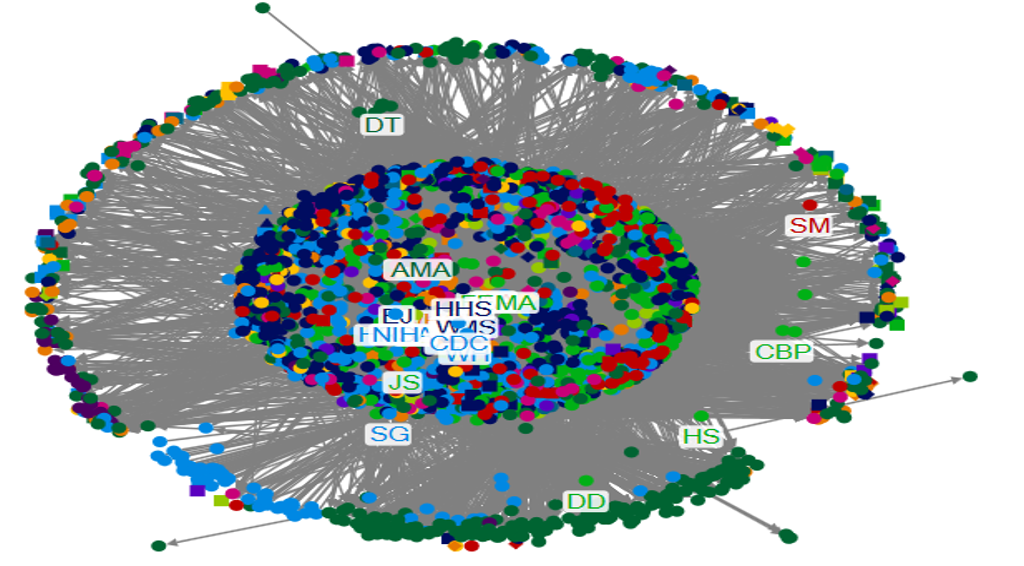
Figure 5: Social networks for the typical case (top: the Centers and bottom: the Department of Health)
Note. Dot colour identified by same-colour label on diagram.
The Centers and the Department of Health also show different social networks for COVID-19 according to groups (see Figure 6). Figure 6 places the top twenty key players and each sub-group in the boxes to improve the clarity of the network visualization. In the Centers networks, group 1 shows dominant communication networks with other groups. Half of the key players (ten out of twenty), such as the World Health Organization, Donald Trump, and the White House, are in group 2 (see Table 4 and 5 for the groups). In contrast, key players are relatively distributed to many groups in the Department of Helath network (e.g. four key players are in group 1, and five key players are concentrated in group 2). Table 3 demonstrates that the Centers and the Department of Health show different characteristics of vertices and unique edges within the top ten groups.
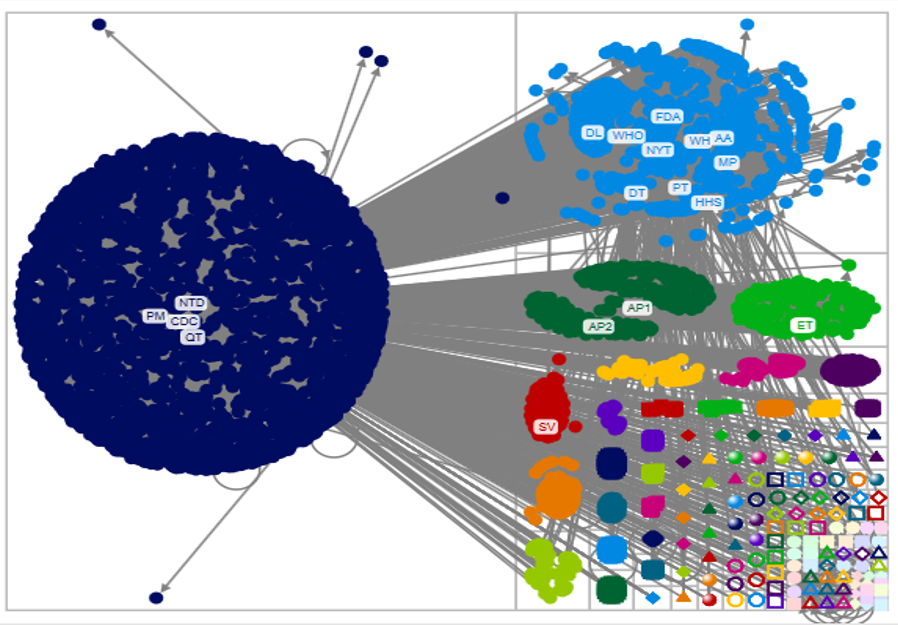
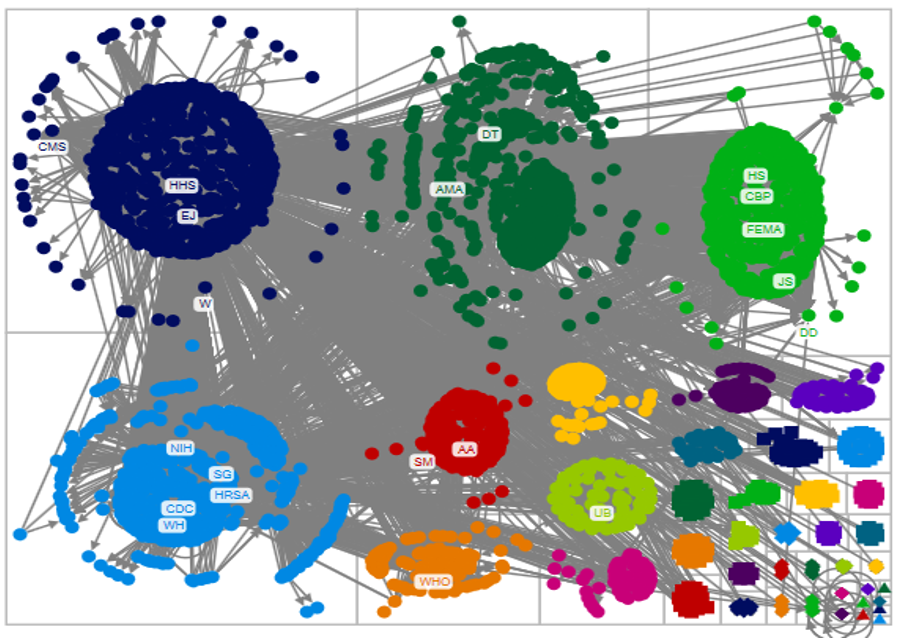
Figure 6. Social networks according to groups (top: the Centers and bottom: the Department of Health
Note. Dot colour identified by same-colour label on diagram.
Group | Centers for Disease Control | Department of Health | ||||||
---|---|---|---|---|---|---|---|---|
Vertices | Unique edges | Proportion of vertices | Proportion of unique edges | Vertices | Unique edges | Proportion of vertices | Proportion of unique edges | |
G1 | 2985 | 4031 | 57.8 | 40.7 | 616 | 1198 | 21.0 | 12.2 |
G2 | 879 | 2185 | 17.0 | 22.0 | 556 | 1071 | 18.9 | 10.9 |
G3 | 191 | 439 | 3.7 | 4.4 | 542 | 1029 | 18.4 | 10.5 |
G4 | 152 | 436 | 2.9 | 4.4 | 456 | 2473 | 15.5 | 25.2 |
G5 | 73 | 138 | 1.4 | 1.4 | 155 | 455 | 5.3 | 4.6 |
G6 | 59 | 105 | 1.1 | 1.1 | 107 | 292 | 3.6 | 3.0 |
G7 | 55 | 78 | 1.1 | 0.8 | 63 | 118 | 2.1 | 1.2 |
G8 | 55 | 83 | 1.1 | 0.8 | 61 | 152 | 2.1 | 1.6 |
G9 | 45 | 58 | 0.9 | 0.6 | 56 | 207 | 1.9 | 2.1 |
G10 | 34 | 61 | 0.7 | 0.6 | 40 | 42 | 1.4 | 0.4 |
Sum | 4528 | 7614 | 87.7 | 76.8 | 2652 | 7037 | 90.2 | 71.8 |
Total | 5166 | 9915 | 100 | 100 | 2940 | 9804 | 100 | 100 |
Also, the Centers' networks and the Department of Health's networks show different characteristics for COVID-19 (see Appendix Table 2 and Table 3). The most important difference between them is that the Centers shows people-based networks, whereas the Department of Health exhibits institute-based networks, as would be expected given their functions and scope of activities. For instance, twelve out of the top twenty accounts are for individuals in the Centers' networks, whereas the same number of institutes play a key role in the Department of Health's networks. This is probably attributable to the fact that the Centers consists of researchers to conduct research and provide information on diseases, whereas the Department of Health consists of institutes, such as the Centers, Food and Drug Administration, National Institutes of Health, and Health Resources and Services Administration. Many studies also find that offline networks play an important role in online networks (e.g., Dunbar et al., 2015; Huang et al., 2014; Kavanaugh et al., 2005; Li and Du, 2017; Reich et al., 2012). The Centers' and the Department of Health's organizational charts support the findings of this study and previous research (Figures 7 and 8). The Centers show the people-based networks, whereas the Department of Health exhibits the institutes-based networks in their offline networks. The finding highlights that offline social networks play an essential role in online social networks.
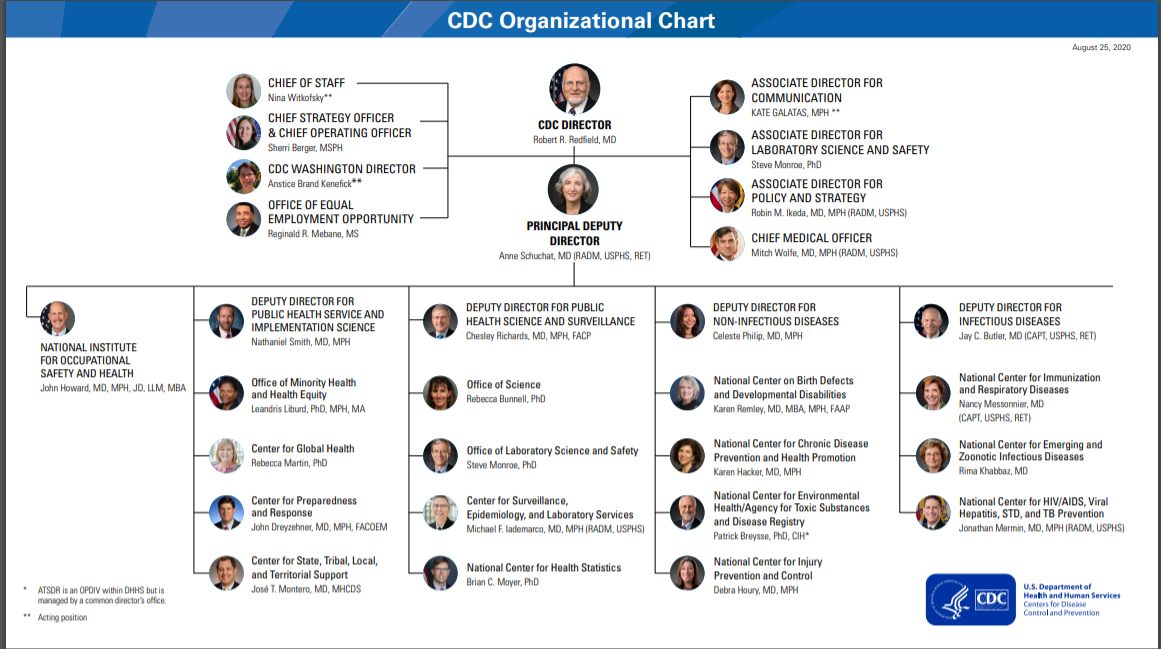
Figure 7: The Centers' organizational chart (U.S. Centers..., 2020)
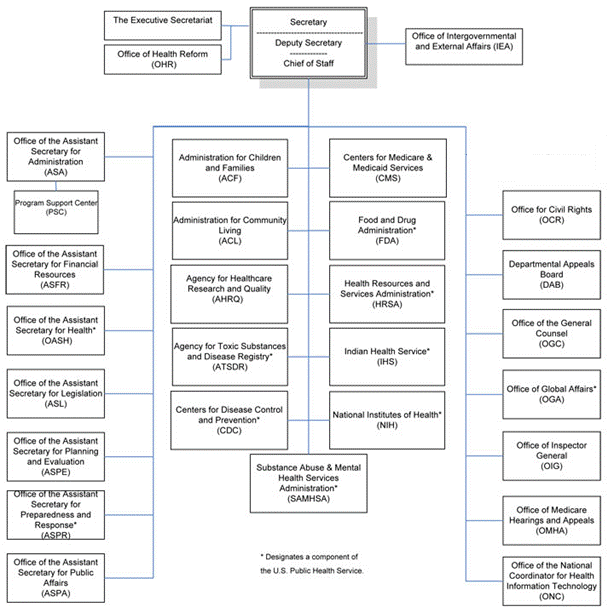
Figure 8: The Department of Health's organizational chart (U.S. Department, 2020)
Limitations
This study has some limitations as follows: first, this study employed the NodeXL software between June 3 and June 10, 2020. Different time periods and different software might result in different results. Secondly, this study only analyses the social networks relating to COVID-19 on Twitter. Future research should explore other popular online platforms, such as Facebook, YouTube, or Instagram. Finally, this study only examines two government institutes in the USA.Conclusions
This study shows five important results as follows:
- This study finds that the Centers networks and the Department of Health networks show different characteristics for COVID-19. The Centers show people-based networks, whereas the Department of Health exhibits institutes-based networks. The result is consistent with their offline social networks. The finding highlights that offline social networks play an essential role in online social networks.
- The Centers and the Department of Health have a different magnitude of importance as a key player role in each other's networks. For example, the Department of Health plays an essential role as the thirteenth key player in the Centers networks, whereas the Centers exert a significant impact on the networks as the second key player in the Department of Health networks. These differences exist because of the different mandates of the Centers and the Department of Health. To be specific, the Centers are the US federal agency that leads COVID-19 research, whereas the Department of Health is the cabinet-level executive branch department of the US federal government that relies on the COVID-19 research of the Centers.
- Some key players play a pivotal role both in the Centers' and Department of Health networks. For instance, World Health Organization ranks third in the Centers' networks and eighteenth in the networks of the Department of Health.
- Social networks of key players are differentiated by centrality types both in the Centers and the Department of Health networks. For example, they are in different places according to institutes and centrality types.
- The Centers and the Department of Health show different characteristics of social networks according to groups. While group 1 plays a main role in the Centers' networks, many groups play an important role in the Department of Health networks.
The results are consistent with the expectation of this study, that is, the Centers and the Department of Health play a different information roles in COVID-19 and have different characteristics of social networks for COVID-19 that reflect the nature of their missions, range of activities and types of functioning.
This study also demonstrated functions of NodeXL, such as in-degree/out-degree/ betweenness centrality, cluster analysis and the Clauset-Newman-Moore cluster algorithm.
Acknowledgements
My thanks to the reviewers and the Regional Editor for their helpful suggestions.
About the author
Seungil Yum is a Ph.D. candidate at the University of Florida with interests in urban economics, economic development, transportation, culture, environment, big data analysis, social network, analysis, and creativity. He can be contacted at yumseungil@ufl.edu.
References
- Aggarwal, C. C. (2011). An introduction to social network data analytics. Springer.
- Aldenderfer, M.S. & Blashfield, R.K. (1984). Cluster analysis. Sage University Papers.
- Arentz, M., Yim, E., Klaff, L., Lokhandwala, S., Riedo, F. X., Chong, M., & Lee, M. (2020). Characteristics and outcomes of 21 critically ill patients with COVID-19 in Washington State. JAMA, 323(16), 1612-1614. https://doi.org/10.1001/jama.2020.4326
- Bonsignore, E. M., Dunne, C., Rotman, D., Smith, M., Capone, T., Hansen, D. L., & Shneiderman, B. (2009). First steps to NetViz Nirvana: evaluating social network analysis with NodeXL. In Proceedings 2009 International Conference on Computational Science and Engineering, Vancouver, BC, Canada, 29-31 August, 2009. (pp. 332-339). IEEE Computer Society. https://doi.org/10.1109/CSE.2009.120
- Bruggeman, J., & Bruggeman, J. (2008). Social networks: an introduction. Routledge.
- Bruns, A., Harrington, S., & Hurcombe, E. (2020). ‘Corona? 5G? or both?' The dynamics of COVID-19/5G conspiracy theories on Facebook. Media International Australia, 177(1), 12-29. https://doi.org/10.1177/1329878X20946113
- Butts, C. T. (2008). Social network analysis: a methodological introduction. Asian Journal of Social Psychology, 11(1), 13-41. https://doi.org/10.1111/j.1467-839X.2007.00241.x
- Cantner, U., & Graf, H. (2006). The network of innovators in Jena: an application of social network analysis. Research Policy, 35(4), 463-480. https://doi.org/10.1016/j.respol.2006.01.002
- Clerkin, K. J., Fried, J. A., Raikhelkar, J., Sayer, G., Griffin, J. M., Masoumi, A., Jain, S.S., Burkhoff, D., Kumaraiah, D., Rabbani, L., Schwartz, A., & Uriel, N. (2020). COVID-19 and cardiovascular disease. Circulation, 141(20), 1648-1655. https://www.ahajournals.org/doi/epub/10.1161/CIRCULATIONAHA.120.046941 (Archived by the Internet Archive at https://bit.ly/2TLbEBb) https://doi.org/10.1161/CIRCULATIONAHA.120.046941
- Clauset, A., Newman, M. E., & Moore, C. (2004). Finding community structure in very large networks. Physical Review E, 70(6), 066111. https://doi.org/10.1103/PhysRevE.70.066111
- du Preez, M. & Meyer, H.W.J. (2016). Consulting engineers' social networks and their collaborative information behaviour. In Proceedings of ISIC, the Information Behaviour Conference, Zadar, Croatia, 20-23 September, 2016: Part 1. Information Research, 21(4), paper isic1611. http://InformationR.net/ir/21-4/isic/isic1611.html (Archived by WebCite® at http://www.webcitation.org/6mHiEbvTV)
- Dunbar, R. I., Arnaboldi, V., Conti, M., & Passarella, A. (2015). The structure of online social networks mirrors those in the offline world. Social Networks, 43, 39-47.
- Durland, M. M., & Fredericks, K. A. (2005). An introduction to social network analysis. New Directions for Evaluation, 2005(107), 5-13. https://doi.org/10.1002/ev.157
- Everitt, B.S., Landau, S. & Leese M. (2001). Cluster analysis (4th ed). Arnold.
- Ewbank, A. D. (2015). Library advocacy through Twitter: a social media analysis of #Savelibraries and #getESEAright. School Libraries Worldwide, 21(2), 26-38. https://scholarworks.montana.edu/xmlui/bitstream/handle/1/14750/Ewbank_SLW_2015_A1b.pdf
- Fang, Y., Nie, Y., & Penny, M. (2020). Transmission dynamics of the COVID‐19 outbreak and effectiveness of government interventions: a data‐driven analysis. Journal of Medical Virology, 92(6), 645-659. https://doi.org/10.1002/jmv.25750
- Gruzd, A., & Mai, P. (2020). Going viral: how a single tweet spawned a COVID-19 conspiracy theory on Twitter. Big Data & Society, 7(2),1-9. https://doi.org/10.1177/2053951720938405
- Hale, T., Angrist, N., Cameron-Blake, E., Hallas, L., Kira, B., Majumdar, S., Petherick, A., Phillips, T., Tatlow, H. & Webster, S. (2020). Variation in government responses to COVID-19. Blavatnik School of Government, University of Oxford. (Working Paper). https://www.bsg.ox.ac.uk/sites/default/files/2020-10/BSG-WP-2020-032-v8.pdf (Archived by the Internet Archive at https://bit.ly/384vUpS)
- Hanneman, R. A., & Riddle, M. (2005). Introduction to social network methods. University of California.
- Hansen, D., Shneiderman, B., Smith, M., Himelboim, I. (2020). Analyzing social media networks with NodeXL: insights from a connected world. (2nd ed). Morgan Kaufmann.
- Hicks, D., Cavanagh, M. F., & VanScoy, A. (2020). Social network analysis: a methodological approach for understanding public libraries and their communities. Library & Information Science Research, 42(3), 1-8. https://doi.org/10.1016/j.lisr.2020.101029
- Huang, G. C., Unger, J. B., Soto, D., Fujimoto, K., Pentz, M. A., Jordan-Marsh, M., & Valente, T. W. (2014). Peer influences: the impact of online and offline friendship networks on adolescent smoking and alcohol use. Journal of Adolescent Health, 54(5), 508-514. https://doi.org/10.1016/j.jadohealth.2013.07.001
- Jiang, F., Deng, L., Zhang, L., Cai, Y., Cheung, C. W., & Xia, Z. (2020). Review of the clinical characteristics of coronavirus disease 2019 (COVID-19). Journal of General Internal Medicine, 35(5), 1545–1549. https://doi.org/10.1007/s11606-020-05762-w
- Johnson, J.D. (2019). Network analysis approaches to collaborative information seeking in inter-professional health care teams. Information Research, 24(1), paper 810. http://InformationR.net/ir/24-1/paper810.html (Archived by WebCite® at http://www.webcitation.org/76tOdYuEss)
- Kavanaugh, A., Carroll, J. M., Rosson, M. B., Zin, T. T., & Reese, D. D. (2005). Community networks: where offline communities meet online. Journal of Computer-Mediated Communication, 10(4), JCMC10417. https://doi.org/10.1111/j.1083-6101.2005.tb00266.x
- Khan, H. U., Nasir, S., Nasim, K., Shabbir, D., & Mahmood, A. (2021). Twitter trends: a ranking algorithm analysis on real time data. Expert Systems with Applications, 164, 1-9. https://doi.org/10.1016/j.eswa.2020.113990
- Kim, S. (2010). A conceptual framework of information requirements for scientists using human biological samples. Information Research, 15(1), paper 427. http://InformationR.net/ir/15-1/paper427.html (Archived by the Internet Archive at https://bit.ly/34QiYly)
- Kim, J., Lee, E., Choi, J., Bae, Y., Ko, M., & Kim, P. (2013). Monitoring social relationship among Twitter users by using NodeXL. In RACS '13: Proceedings of the 2013 Research in Adaptive and Convergent Systems, Montreal, Quebec, Canada, October, 2013 (pp. 107-110). Association for Computing Machinery. https://doi.org/10.1145/2513228.2513275
- Kjellberg, S., Haider, J., & Sundin, O. (2016). Researchers' use of social network sites: a scoping review. Library & Information Science Research, 38(3), 224-234. https://doi.org/10.1016/j.lisr.2016.08.008
- Li, F., & Du, T. C. (2017). The effectiveness of word of mouth in offline and online social networks. Expert Systems with Applications, 88, 338-351. https://doi.org/10.1016/j.eswa.2017.07.004
- McBirnie, A., & Urquhart, C. (2011). Motifs: dominant interaction patterns in event structures of serendipity. Information Research, 16(3), paper 494. http://InformationR.net/ir/16-3/paper494.html (Archived by the Internet Archive at https://bit.ly/3mXPI2v)
- McKenzie, M.L. (2005). Managers look to the social network to seek information. Information Research, 102) paper 216. http://InformationR.net/ir/10-2/paper216.html (Archived by the Internet Archive at https://bit.ly/3kUdThm)
- Mizumoto, K., Kagaya, K., Zarebski, A., & Chowell, G. (2020). Estimating the asymptomatic proportion of coronavirus disease 2019 (COVID-19) cases on board the Diamond Princess cruise ship, Yokohama, Japan, 2020. Eurosurveillance, 25(10), 2000180. https://doi.org/10.2807/1560-7917.ES.2020.25.10.2000180
- Mowbray, J, Hall, H, Raeside, R & Robertson, P (2017). The role of networking and social media tools during job search: an information behaviour perspective. Information Research, 22(1), CoLIS paper 1615. http://InformationR.net/ir/22-1/colis/colis1615.html (Archived by WebCite® at http://www.webcitation.org/6oJcmtjD9)
- Munoz-Munoz, A.M. & Miron-Valdivieso, M.D. (2017). Analysis of collaboration and co-citation networks between researchers studying 'violence involving women'. Information Research, 22(2), paper 758. http:// informationr.net/ir/22-2/paper758.html (Archived by WebCite® at http://www.webcitation.org/6r5U5gOsZ)
- Ndinojuo, B. C. E. (2020). 5G, Religion, and misconceptions in communication during Covid-19 in Nigeria. Jurnal The Messenger, 12(2), 97-110. http://dx.doi.org/10.26623/themessenger.v12i2.2282
- Nelissen, S., Van den Bulck, J. & Beullens, K. (2017). A typology of cancer information seeking, scanning and avoiding: results from an exploratory cluster analysis. Information Research, 222), paper 747. http://InformationR.net/ir/22-2/paper747.html (Archived by WebCite® at http://www.webcitation.org/6r2QayaVb)
- Ortega Egea, J.M., Recio Menéndez, M. and Román González, M.V. (2007). Diffusion and usage patterns of Internet services in the European Union. Information Research, 12(2) paper 302. http://InformationR.net/ir/12-2/paper302.html (Archived by the Internet Archive at https://bit.ly/2HWNAcg)
- Pálsdóttir, Á. (2008). Information behaviour, health self-efficacy beliefs and health behaviour in Icelanders' everyday life. Information Research, 13(1) paper 334. http://InformationR.net/ir/13-1/paper334.html (Archived by the Internet Archive at https://bit.ly/382QYge)
- Park, M.S. & Park, H. (2016). Topical network of breast cancer information in a Korean American online community: a semantic network analysis. Information Research, 21(4), paper 729. http://InformationR.net/ir/21-4/paper729.html (Archived by WebCite® at http://www.webcitation.org/6m5HD6NnJ)
- Reich, S. M., Subrahmanyam, K., & Espinoza, G. (2012). Friending, IMing, and hanging out face-to-face: overlap in adolescents' online and offline social networks. Developmental psychology, 482), 356-368. https://psycnet.apa.org/doi/10.1037/a0026980
- Salehi, S., Abedi, A., Balakrishnan, S., & Gholamrezanezhad, A. (2020). Coronavirus disease 2019 (COVID-19): a systematic review of imaging findings in 919 patients. American Journal of Roentgenology, 215(1), 1-7. https://www.ajronline.org/doi/full/10.2214/AJR.20.23034?journalCode=ajr
- Sanders, J. M., Monogue, M. L., Jodlowski, T. Z., & Cutrell, J. B. (2020). Pharmacologic treatments for coronavirus disease 2019 (COVID-19): a review. JAMA, 323(18), 1824-1836. https://doi.org/10.1001/jama.2020.6019
- Scott, J. (1988). Social network analysis. Sociology, 22(1), 109-127. https://doi.org/10.1177/0038038588022001007
- Singhal, T. (2020). A review of coronavirus disease-2019 (COVID-19). The Indian Journal of Pediatrics, 87(4), 281–286. https://doi.org/10.1007/s12098-020-03263-6
- Sohrabi, C., Alsafi, Z., O'Neill, N., Khan, M., Kerwan, A., Al-Jabir, A., Iosifidis. C. & Agha, R. (2020). World Health Organization declares global emergency: a review of the 2019 novel coronavirus (COVID-19). International Journal of Surgery, 76, 71–76. https://doi.org/10.1016/j.ijsu.2020.02.034
- Stvilia, B., & Gibradze, L. (2014). What do academic libraries tweet about, and what makes a library tweet useful? Library & Information Science Research, 36(3-4), 136-141. https://doi.org/10.1016/j.lisr.2014.07.001
- Sullivan, M. C. (2019). Leveraging library trust to combat misinformation on social media. Library & Information Science Research, 41(1), 2-10. https://doi.org/10.1016/j.lisr.2019.02.004
- Sundling, P. (2017). Library and information science according to the citing pattern of students: a bibliometric study. Information Research, 22(1), CoLIS paper 1622. http://InformationR.net/ir/22-1/colis/colis1622.html (Archived by WebCite® at http://www.webcitation.org/6oTQ6SrnX)
- Torres-Salinas, D., Cabezas-Clavijo, Á., Ruiz-Pérez, R., & López-Cózar, E. D. (2011). State of the library and information science blogosphere after social networks boom: a metric approach. Library & Information Science Research, 33(2), 168-174. https://doi.org/10.1016/j.lisr.2010.08.001
- U.S. Centers for Disease Control and Prevention. (2020). CDC organization chart. https://www.cdc.gov/about/organization/orgchart.htm (Archived by the Internet Archive at https://bit.ly/2HXyOC3)
- U.S. Department of Health and Human Services. (2020). Organizational chart Department of Health and Human Services. https://www.hhs.gov/about/budget/fy2017/performance/performance-plan-overview/index.html (Archived by the Internet Archive at https://bit.ly/3mKv9Gr)
- Van der Hulst, R. C. (2009). Introduction to social network analysis (SNA) as an investigative tool. Trends in Organized Crime, 12(2), 101-121. https://doi.org/10.1007/s12117-008-9057-6
- Vieira, V. D. F., Xavier, C. R., Ebecken, N. F. F., & Evsukoff, A. G. (2014). Performance evaluation of modularity based community detection algorithms in large scale networks. Mathematical Problems in Engineering, 2014, Article ID 502809. https://doi.org/10.1155/2014/502809
- World Health Organization. (2020a). Transmission of COVID-19 by asymptomatic cases.. World Health Organization. http://www.emro.who.int/health-topics/corona-virus/transmission-of-covid-19-by-asymptomatic-cases.html (Archived by the Internet Archive at https://bit.ly/2TM0HiL)
- World Health Organization. (2020b). Timeline: WHO's COVID-19 response. World Health Organization. https://www.who.int/emergencies/diseases/novel-coronavirus-2019/interactive-timeline#! (Archived by the Internet Archive at https://bit.ly/3jSh6g4)
- Wu, Z., & McGoogan, J. M. (2020). Characteristics of and important lessons from the coronavirus disease 2019 (COVID-19) outbreak in China: summary of a report of 72 314 cases from the Chinese Center for Disease Control and Prevention. JAMA, 323(13), 1239-1242. https://doi.org/10.1001/jama.2020.2648
- Yu, X., & Yang, R. (2020). COVID‐19 transmission through asymptomatic carriers is a challenge to containment. Influenza and Other Respiratory Viruses, 14(4), 474475. https://doi.org/10.1111/irv.12743
- Yum, S. (2020). Social network analysis for coronavirus (COVID‐19) in the United States. Social Science Quarterly, 101(4), 1642-1647. https://doi.org/10.1111/ssqu.12808
- Zaphiris, P., & Pfeil, U. (2007). Introduction to social network analysis. In Proceedings of HCI 2007 The 21st British HCI Group Annual Conference, University of Lancaster, UK, (pp. 1-2). BCS Learning and Development Ltd https://doi.org/10.14236/ewic/HCI2007.89 (https://bit.ly/3elv1u6 - Archived by the Internet Archive at https://bit.ly/3oOMa4f)
How to cite this paper
Appendices
Graph metric | The Centers | The Department |
---|---|---|
Graph type | Directed | Directed |
Maximum betweenness centrality | 25826489 | 240 |
Total edges | 12662 | 13672 |
Maximum edges in a connected component | 12636 | 13663 |
Unique edges | 9915 | 9804 |
Average betweenness centrality | 6507 | 3 |
Vertices | 5166 | 2940 |
Maximum vertices in a connected component | 5141 | 2932 |
Maximum in-degree | 4435 | 2091 |
Edges with duplicates | 2747 | 3868 |
Self-loops | 127 | 65 |
Maximum out-degree | 46 | 240 |
Connected components | 20 | 8 |
Single-vertex connected components | 15 | 6 |
Maximum geodesic distance (Diameter) | 4 | 5 |
Average geodesic distance | 2.272 | 2.535 |
Average in-degree | 2.073 | 3.595 |
Average out-degree | 2.073 | 3.595 |
Median out-degree | 2 | 3 |
Reciprocated edge ratio | 0.004 | 0.010 |
Reciprocated vertex pair ratio | 0.002 | 0.005 |
Graph density | 0.000 | 0.001 |
Minimum in-degree | 0 | 0 |
Median in-degree | 0 | 0 |
Minimum out-degree | 0 | 0 |
Minimum betweenness centrality | 0 | 0 |
Median betweenness centrality | 0 | 3 |
Label | In-degree | Name | Description | Examples of tweets in social networks | |
---|---|---|---|---|---|
1 | CDC | 4435 | CDC @CDCgov | Centers for Disease Control and Prevention provide health considerations and tools for operating during COVID-19. | BREAKING @CDCgov just announced they're adopting key tenants of our racial data bill. |
2 | PM | 628 | Phil Murphy @GovMurphy | Governor of New Jersey. | Governor Murphy declared a state of emergency in New Jersey. If you think this is 'just the flu' – you are 100% wrong. |
3 | WHO | 335 | WHO @WHO | World Health Organization. WHO plays a leading role in COVID-19 response. | How complicit is the #WHO in #China's #coronavirus cover-up? |
4 | DT | 195 | Donald Trump @realdonaldtrump | The US president. President Trump declared a national emergency against COVID-19. | When will the American people have some real data on deaths due to Covid19? |
5 | QT | 181 | QuickTake by Bloomberg @quicktake | Global news network. Bloomberg provides the latest coronavirus news, including live updates, maps and ... Health authorities around the world are fighting a pandemic of Covid-19. | Despite initial data from China that showed elderly people and those with other health conditions were most vulnerable, young people in their 20s to early 40s are falling seriously ill from the #coronavirus. |
6 | DL | 175 | David Leopold @davidleopold | Immigration attorney. He posts many tweets about COVID-19 policies for immigrants. | Another Trump lie. Turns out More than a dozen US researchers, physicians & public health experts @CDCgov were working at @WHO's Geneva HQ when #COVID19 emerged last |
7 | ET | 149 | Eric Topol @erictopol | American cardiologist. He is the Founder and Director of the Scripps Research Translational Institute and discusses the evolving COVID-19 pandemic. | The @CDCgov used to be regarded as exemplary, the model emulated by other countries. What happened w/ #COVID19? |
8 | EF | 145 | Eric Feigl-Ding @drericding | American scientist, currently a Visiting Scientist in the Department of Nutrition at the Harvard T.H. Chan School of Public Health. He is immensely popular on Twitter for COVID-19. | Thailand study says #Covid19 patients can be infectious even after their symptomatic recovery. |
9 | JH | 143 | Jim Harris @jimharris | Disruptive Innovation Speaker. He was leader of the Green Party of Canada from 2003 to 2006. He argues that the COVID-19 crisis is accelerating digital transformation. | 'Sweden chose to ignore the need for staying at home and social distancing'. |
10 | AP1 | 112 | Ayanna Pressley @ayannapressley | U.S. Representative. She has served as the U.S. Representative for Massachusetts's 7th congressional district since 2019. She discusses the urgent need for direct, emergency aid to state and local governments to manage the COVID-19. | @AyannaPressley @CDCgov Latest CDC reporting on #COVID19 race & age breakdown. |
11 | NTD | 93 | NTD News @news_ntd | America's new independent TV channel. The news frequently focuses on Chinese people for COVID-19. | A doctor is voicing his concern that the @CDCgov guideline on how to complete #COVID19 death certificates may influence certifiers to inflate the number of deaths from the disease. |
12 | WH | 91 | The White House @whitehouse | The official workplace of the president of the US. The White House provides Coronavirus Guidelines for America. | How complicit is the #WHO in #China's #coronavirus cover-up? |
13 | HHS | 86 | HHS @HHSGov | The U.S. Department of Health and Human Services. | Our top priority with the #COVID19 response is to ensure critical supplies get to the right people at the right time, based on analysis by @HHSGov and @CDCgov. |
14 | PT | 78 | President Trump @potus | 45th President of the US. President Trump declared a national emergency against COVID-19. | A scientific doc published nearly 10 yrs ago, Zinc inhibits #COVID19 activity & blocking its replication. |
15 | AP2 | 78 | Congresswoman Ayanna Pressley @reppressley | U.S. Representative. She has served as the U.S. Representative for Massachusetts's 7th congressional district since 2019. She discusses the urgent need for direct, emergency aid to state and local governments to manage the COVID-19. | @CDCgov is acting on calls from me |
16 | MP | 77 | Mike Pence @vp | Vice President of the United States. In February 2020, Pence was appointed chairman of the White House Coronavirus Task Force, established in response to the COVID-19 pandemic in the United States | Trump and the Coronavirus Task Force needs to stop getting in front of the science. @VP |
17 | AA | 76 | Alex Azar @secazar | United States Secretary of Health and Human Services. Azar informed associates that he had alerted Trump on January 18, 2020, regarding the potential danger from COVID-19. | 'Has the US lost its seat at the #GlobalHealth table in the midst of the #COVID19 response?' @SecAzar |
18 | SV | 69 | Seema Verma @seemacms | Administrator for the Centers for Medicare & Medicaid Services. She an American health policy consultant for COVID-19. | Nursing homes must now report to patients, their families and @CDCgov if there are cases of #COVID19 in their facilities, announces @SeemaCMS. |
19 | NYT | 67 | The New York Times @nytimes | American newspaper. The newspaper provides Live Covid-19 Updates and Tracker. | Thailand study says #Covid19 patients can be infectious even after their symptomatic recovery. |
20 | FDA | 66 | U.S. FDA @us_fda | Food and Drug Administration. The FDA is responsible for protecting and promoting public health against COVID-19. | The @CDCgov, @US_FDA, + @WHO all agree: tobacco use can put people at higher risk for severe illness from #COVID19. |
Label | In-degree | Name | Description | Examples of tweets in social networks | |
---|---|---|---|---|---|
1 | HHS | 2091 | HHS @HHSGov | United States Department of Health and Human Services. the Department of Health works with state, local, tribal and territorial governments, public health officials, health care providers, researchers, private sector organizations and the public to execute a whole-of-America response to the COVID-19 pandemic. | Our top priority with the #COVID19 response is to ensure critical supplies get to the right people at the right time, based on analysis by @HHSGov and @CDCgov. |
2 | CDC | 512 | CDC @CDCgov | Centers for Disease Control and Prevention. CDC provides health considerations and tools for operating during COVID-19. | BREAKING @CDCgov just announced they're adopting key tenants of our racial data bill. |
3 | FEMA | 495 | FEMA @fema | Federal Emergency Management Agency. The agency's primary purpose is to coordinate the response to a disaster that has occurred in the United States, such as COVID-19. | We are working closely with @HHSGov to support states #COVID19 testing capabilities. |
4 | HS | 308 | Homeland Security @dhsgov | United States Department of Homeland Security. It is the U.S. federal executive department responsible for public security, including COVID-19. | It could be WORSE in the fall. |
5 | CBP | 295 | CBP @cbp | U.S. Customs and Border Protection. It is the country's primary border control organization against COVID-19. | @CBP @DHSgov @CBPArizona Affecting all detained-no one deserves #COVID19 as cases of ill+dying. |
6 | WH | 254 | The White House @whitehouse | The official workplace of the president of the US. The White House provides Coronavirus Guidelines for America. | How complicit is the #WHO in #China's #coronavirus cover-up? |
7 | AA | 183 | Alex Azar @secazar | United States Secretary of Health and Human Services. | Azar informed associates that he had alerted Trump on January 18, 2020, regarding the potential danger from COVID-19. Has the US lost its seat at the #GlobalHealth table in the midst of the #COVID19 response? @SecAzar |
8 | SG | 179 | U.S. Surgeon General @surgeon_general | The operational head of the U.S. Public Health Service. The U.S. surgeon general provides a lot of COVID-19 information. | NEW: @HHSGov through @HRSAgov awards $90M to support 581 #RyanWhite HIV/AIDS Program recipients in their efforts to prevent or minimize the impact of #COVID19 pandemic on people with #HIV: |
9 | DT | 129 | Donald Trump @realdonaldtrump | The US president. President Trump declared a national emergency against COVID-19. | When will the American people have some real data on deaths due to Covid19? |
10 | DD | 105 | Department of Defense @deptofdefense | Executive branch department of the federal government. The Department of Defense restricted all domestic and overseas travel to control COVID-19. | This is particularly important as we #StayHome during #COVID19. |
11 | JS | 95 | The Joint Staff @thejointstaff | Senior uniformed leaders in the United States Department of Defense. The Joint Staff discusses the Defense Department's response to the COVID-19 pandemic. | #GenMilley delivers an opening statement re current @DeptofDefense #COVID19 response efforts. |
12 | NIH | 82 | NIH @nih | National Institutes of Health. the National Institutes of Health releases resources and news regarding COVID-19. | Did you know Congress and their staff were getting daily briefings starting in January from @HHSGov @CDCgov @NIH and the @WhiteHouse about #COVID19? |
13 | AMA | 78 | AMA @amermedicalassn | American Medical Association. The association provides COVID-19 resource center for physicians. | We called on @HHSGov to ensure that physicians were included in the first round of CARES Act disbursements. |
14 | W | 77 | Walgreens @walgreens | The second-largest pharmacy store chain in the US. No-cost COVID-19 testing is available at select Walgreens locations. | Promises made...promises not kept. We will remember how @GOP & Trump Lied #COVID19 @Walgreens |
15 | HRSA | 77 | HRSA @hrsagov | Health Resources and Services Administration. It is the primary federal agency for improving access to health care services for COVID-19. | #StayHomeSaveLives |
16 | EJ | 74 | Eric Johnson @johnson4dallas | Mayor of Dallas. Eric Johnson encourages people to wear a mask and provides important news for COVID-19. | Good news: On April 10, I sent a letter to @HHSGov & @Walgreens urging them to select Dallas (particularly southern Dallas) for a new 15-minute #COVID19 testing site. |
17 | UB | 62 | Uché Blackstock @uche_blackstock | American physician. During the COVID-19 pandemic Blackstock used social media to share her experiences and concerns as a physician working on the front lines and on racial health disparities and inequities exposed by the pandemic. | Thank you to the Dutch news station @Nieuwsuur for providing a platform for me to amplify the #COVID19 racial disparities message and to continue the call for @HHSGov to release racial and ethnic demographic data on #COVID19 testing, cases and mortality. |
18 | WHO | 62 | WHO @WHO | World Health Organization. WHO plays a leading role in COVID-19 response. | How complicit is the #WHO in #China's #coronavirus cover-up? |
19 | SM | 60 | Steven Mnuchin @stevenmnuchin1 | United States Secretary of the Treasury. Mnuchin testifies on federal response to COVID-19 and its impact on the economy. | @atrupar This is my idea—Put @realDonaldTrump's signature on the death certificate of every US victim of #COVID19. |
20 | CMS | 59 | CMSGov @cmsgov | The Centers for Medicare & Medicaid Services. CMSGov releases valuable information and updates about COVID-19. | Why are none of our medical/ gov organizations keeping track of #HCW #COVID19 exposure in a systematic way? |