Multi-dimensional analysis of dynamic human information interaction
Minsoo Park
Korea Institute of Science and Technology Information, Seoul, Korea
Introduction
Our adaptation occurs from our ability to process information and modify our information behaviour accordingly (Morgan 2002). Since the advent of the Web, humans have lived in dynamic, volatile digital information environments. Due to the dynamism and complexity of the Web information environments, people are getting more involved in multiple information task behaviours (Waller 1997). Multiple task performance is an important human behaviour that allows people to manage complex situations by handling more than one task in an effective, coordinated way (Burgess 2000; Carlson and Sohn 2000; Lee and Taatgen 2002).
The Compact Oxford English Dictionary (2005) defines multiple task performance as the ability to perform concurrent tasks by interleaving. In cognitive science, the concept of multiple task performance is more complicated than interleaving tasks in a multiple task sense. The complex situations people face often demand further mental activities, such as prioritisations and planning (Burgess 2000).
Multiple task performance has been important in the research of cognitive science, engineering psychology, human computer interaction, and human factors (e.g., Damos 1991; Treisman 1960; Wickens 1989). Early studies in the literature of multiple task performance in cognitive science focused mainly on dichotic listening skills (e.g., Broadbent 1958; Treisman 1960), during which people were asked to listen to two simultaneous messages and shadow one. Later research has focused on both cognitive and motor task performance (e.g., Wickens 1989). According to Wickens researchers in multiple task performance include both task characteristics and coordination processes.
While some tasks can be easily performed concurrently, others compete for cognitive capacities (Wickens 2002; Wickens et al. 2003) and as a consequence, people need to efficiently prioritise and coordinate their tasks with appropriate efforts to accomplish each task successfully. The way in which task prioritisations, task characteristics, and mental effort (cognitive resources) are connected has not yet been explored in information seeking and retrieval contexts. Research on multiple information task interaction in humans in such contexts is necessary for a greater understanding of human information behaviour in dynamic and complex information environments.
Recent studies show that people often perform multiple information tasks while using Web information retrieval (IR) technologies and looking for information through more than one information task over multiple search episodes (Spink 2004; Spink et al. 2002). In an exploratory study of human multiple information task behaviour, Spink et al. (2006) found that information task prioritising processes were influenced by the following factors: level of interest, level of knowledge, perceived level of information available on the Web, level of difficulty, level of importance, and information seeking from general information problems to specific ones.
Multiple task performance has been an important area of study in cognitive science, engineering psychology, human computer interaction, and human factors, but human multiple information task interaction during information seeking and retrieval processes in the Web environment is under-explored.
The overall goal of this study is to explore the relationships of task demand, mental effort, affective state, temporal demand, and performance in the processes of prioritising and coordinating multiple information tasks in the contexts of seeking and retrieving information on the Web.
Literature review
Different theoretical approaches to attention suggest that psychologists are far from agreeing on how to explain the attentional phenomena. Just et al. (2001) defined attention as limited cognitive capacity that can be distributed over tasks, such as in divided attention tasks. Kahneman (1973) viewed attention as a set of cognitive processes for categorizing and recognizing stimuli; the more complex the stimulus, the harder the processing, and therefore the more resources are engaged. Despite the different theoretical approaches, attention has been shown to a flexible aspect of cognition (Kahneman 1973). We see that attention, rather than being rigidly and mechanically limited, is instead a more flexible system, affected by factors such as the complexities of tasks and the person’s intention.
In Treisman’s (1960, 1964) attenuation theory, irrelevant messages (or less important messages) are turned-down through the multi-stage processing (physical/sensory processing, linguistic processing, and semantic/meaning processing), leaving more attention for important information. The turned-down messages are still partially accessible and monitored occasionally. Some messages have low thresholds and are easily processed, e.g., names, danger signals. Unattended information is still available, just less accessible. It requires little constraint on what gets through.
Work such as that by Johnston and Heinz (1978) has led many to use new metaphors when explaining attention. For instance, some compare attention to a spotlight that highlights whatever information the system is currently focused on (Johnson and Dark 1986). Accordingly, psychologists are now interested less in determining what information cannot be processed than exploring what kinds of information people choose to focus on.
In multimode theory (Johnston and Heinz 1978), attention is assumed to be flexible in that attended and non-attended information can be differentiated at different depths of perceptual analysis. Multimode theory assumes that the more processing needed, the greater the capacity and mental effort required, and the later the selection, the harder the task. Free attentional capacity decreases the later the selection occurs.
Kahneman (1973) presented a slightly different model to explain attention. He viewed attention as a set of cognitive processes for categorising and recognising stimuli. The more complex the stimulus, the harder the processing, and therefore the more resources are engaged. However, according to Kahneman, people have some control over where they direct their mental resources: They can often choose what to focus on and devote their mental effort to. Kahneman’s (1973) model of attention indicates how attention can be considered as a flexible system affected by several factors but has a limitation in explaining how an individual’s enduring dispositions and momentary intentions affect the processes of resource allocation. Essentially, this model suggests that we pay more attention to things we are interested in or have judged important.
Everyday observations tell us that the more one concentrates, the better one performs. Many researchers have employed the notion of mental effort (cognitive resources) as a hypothetical construct to explain performance differences (Bandura 1982; Salomon 1981, 1983; Salomon and Leigh 1984).
Bobrow and Collins (1975: 145) pointed out:
Data which were either deemed as important or could not easily be accounted for would receive sufficient processing effort and as a result, they would probably be remembered later. Moreover, we suspect that they would receive conscious attention at the time of their arrival and processing. Thus, data which are expected or otherwise, readily accounted for will be remembered.
To explain the essence of what is meant by constructs such as depth of processing and cognitive capacity, Salomon (1981, 1983) used the construct of amount of invested mental effort, which is defined as 'the number of non-automatic mental elaborations applied to a unit of material'. (Salomon 1984: 648) This concept is based on Kahneman’s (1973) attention theory, which assumes that one has a pool of available mental effort (cognitive capacity) that can be allocated to tasks and conscious information processes demand mental effort and therefore tap the pool of cognitive resources.
According to Salomon, the amount of invested mental effort indicates cognitive and motivational attributes:
It is cognitive in the sense that it pertains to mental elaborations of information material. But as these elaborations are controlled, rather than automatic, their employment implies a measure of choice, as all controlled activities do (Steiner, 1979). The exercise of choice, the preference of one alternative course of action over another, implies in turn the existence of motivation (Brigham, 1979). Non-automatic effort demanding elaborations are at one’s disposal; their actual employment is a matter of choice and motivation. (Salomon 1983: 44)
Motivation, curiosity, anxiety, or arousal may all be necessary factors in producing greater invested mental effort (Salomon 1981). But according to Salomon (1981) the invested mental effort ultimately produces learning. High motivation, even when accompanied by comprehensive knowledge or skill, is not sufficient to produce high performance unless one actually invests mental effort in processing information (Clark 1980).
Langer, Blank, and Chanowitz (1978) showed that when information or stimuli are considered as highly familiar, people tend to respond to them mindlessly, thereby resulting in low performance. The perception toward task difficulty is also thought to influence the amount of effort expended (Weiner 1985). Weiner found that individuals seem to perform best at tasks of intermediate difficulty. When faced with tasks of intermediate difficulty, people invest more effort since these individuals believe that the best performance strategy for high achievement in such situations is to try harder. These studies suggest that invested mental effort may depend on the perceived demand characteristics of the stimulus, the task, or the context.
More demanding, difficult or novel stimuli are generally expected to evoke more effort investment than simple stimuli (Salomon 1983). But according to Salomon, the nature of stimuli, their complexity, novelty, and the like, in interaction with learners’ abilities, affect performance or learning outcomes only to some extent. Perceptions, in the sense of predispositions, preconceptions, attitudes, or attributions, also play an important role in the way one processes information.
In a series of studies (Salomon 1983, 1984; Salomon and Leigh 1984), Salomon addressed the question of how individuals’ perceptions of information categories relate to the amount of invested mental effort and performance. The findings of these studies indicate that differential perceptions of tasks are related to the amount of invested mental effort, which in turn is related to performance and learning. The results suggest that individuals’ performance may depend on what they perceive the tasks to be.
Taken as a whole, this line of research suggests that individuals’ perceived demand characteristics of tasks or contexts affect AIME. The more demanding PDC is, the more AIME would be expended. When people face a task they perceive to be easy relative to their abilities, they may invest less mental effort in its processing or performance. For example, a skilled driver or Web surfer may perceive the respective task of driving or Web surfing to be easy and thus rely more on automatic processes. This is due to knowing that no additional effort investments are needed to perform the task that is perceived to be well mastered (Salomon 1984).
Research in human information behaviour, shows that people often face multiple task situations in information contexts. When they have multiple information tasks or problems, they tend to batch those problems or tasks and try to solve them at once, often using information retrieval systems (Spink 2004). Studies indicate that users’ searches may have multiple goals or topics in the contexts of human information interaction (Miwa 2001; Spink 2004).
The concept of human information coordinating behaviour has been introduced in the studies of human multitasking information behaviour (Spink et al. 2006; Spink et al. 2002; Spink et al. 2006). The general meaning of coordinating is bringing the different elements of a complex activity into a relationship that will ensure efficiency (Compact Oxford English Dictionary 2005). Spink, Park, and Cole emphasise that:
the concept of HICB [human information coordinating behaviour] is an important linking and sustaining process for a science of information that binds together the many HIB [human information behaviour] processes. The development of HIB necessitates a theoretical and empirical explication of the important nature and role of HIB’s, including HICB. In HICB, humans coordinate a number of elements, including their cognitive state, level of domain knowledge, and their understanding of their information problem, into a coherent series of activities that may include seeking, searching, interactive browsing, retrieving, and constructing information. A key process for HICB is to sustain these activities toward completion of some information goal or object. (Spink et al.2006: 150)
In the context of information access and use, task attributes have been investigated in numerous studies (e.g., Byström and Järvelin 1995; Culnan 1983; Hart and Rice 1991; Tiamiyu 1992). These studies show that task characteristics (e.g., task complexity) influence the way people seek and use information. Task complexity is multidimensional (Campbell 1988) and there are systematic interplays among task complexity, information types, information / communication channels, and sources (Bystrom and Jarvelin 1995).
Theoretical model
From the analysis of the studies reviewed, a theoretical model of human prioritising and coordinating information behaviour in the contexts of information seeking and retrieval emerged.
Figure 1 depicts the process of prioritising and coordinating multiple information tasks in the contexts of information seeking and retrieval. The model is fundamentally based on the global single-channel hypothesis, which is all of the mechanisms between stimulus input and response output (stimulus perception, response selection, movement initiation) together constitute a single-channel and can be used by only one task at a time (Craik 1948).
In this model, differential perceptions of tasks initiate the process by influencing the dimensions of task prioritisations, cognitive effort, affective state, and temporal demand. All these components are in turn related to the level of task performance. Performance is then followed by evaluation. Interplay plays an important role in the processes of human prioritising and coordinating behaviour over time in the context of multiple information tasks.
In this study, the notion of multiple task performance is coming from an interdisciplinary perspective of human information interaction. The conceptual foundation of multiple task performance has been built on the studies of cognitive psychology, human factors, and educational psychology and it has been extended in the contexts of information seeking and retrieval in human information interaction. This exploratory study seeks a multi-dimensional understanding of human prioritising and coordinating information behaviour by investigating, in particular, the relationships of task demands, cognitive effort, affective state, temporal demand, and performance.
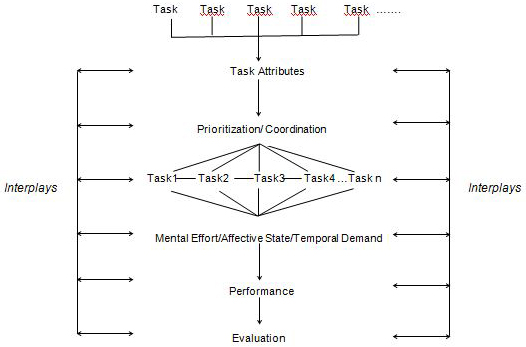
Figure 1. Theoretical model of human prioritizing and coordinating information behaviour
The next section details the methods used in this study.
Research design
This study aims to understand the relationships of perception, effort, emotion, time, and performance when people manage multiple information tasks using Web information technologies.
Data collection instrument
Data were collected from questionnaires completed before and after the task completion exercise. They were used to obtain general background information and examine the research problem. The pre-task questionnaire contained closed and open-ended questions. The post-task questionnaire was mainly made up of rating scales, applied to collect data on the levels of each of the four dimensions associated with assessment of the following variables: task demand (task dimension), mental effort (cognitive dimension), performance (behavioural dimension), temporal demand (temporal dimension) and psychological or affective level (psychological/affective dimension). Each dimension was defined as follows:
- Task demand: the perceived levels of difficulty, importance, interest, familiarity or complexity of each task.
- Mental effort: the experienced levels of cognitive demand required to accomplish each task.
- Affective state: the experienced levels of emotion or feeling during the task.
- Temporal demand: the experienced levels of time pressure because of the rate at which the tasks occurred.
- Performance: the experienced levels of success or satisfaction
The post-task questionnaire was designed based on the subjective workload assessment technique (Reid and Nygren 1988) with substantial modifications for the purpose of this study. Each scale in the pre and post questionnaires was presented as a 12-cm line with bipolar descriptors at each end (e.g., high or low, excellent or poor). Numerical values were not displayed on the rating scales. A questionnaire was chosen because it has the potential to collect cognitive data quickly and easily. Another advantage of a questionnaire is that the data may be both qualitative and quantitative, allowing them to play a part in both quantitative and qualitative studies (Su 1991).
Subject sample
The first step in choosing a sample is to decide who should be included in the population of interest (Krathwohl 2004). For this study, students and faculty members were the sampled population. The reason for choosing this population was that it was assumed that they regularly interacted with the Web for information or knowledge. Web information technologies have played an important role in electronic learning environments. Students and faculty regularly interact with information and information retrieval systems (e.g., the Web, databases, digital libraries) to solve their information problems and/or broaden their knowledge horizons in electronic learning environments.
Twenty volunteers with diverse academic backgrounds, including telecommunications, library and information science, environmental studies, health and community systems, sociology, nursing, and health information management, participated in this study. All students and faculty members at a university were asked to take part in the study. A notice of recruitment was distributed through emailing lists and notice boards. No demographic and disciplinary limits were set in order to minimise bias in the sampling process.
Data collection
The tasks
After giving general instructions, the subjects were asked to create one information problem for the non-assigned (additional) task. They were then given general descriptions of three different information tasks in random order. It was the participants who decided on the entire process of their searching sessions, e.g., which task they were going to begin with. They had a maximum of one hour to finish their sessions regardless of the state of completion of all tasks. The subjects conducted one task at a time. The three assigned tasks were related to medicine, travel, and research. The descriptions of the assigned tasks were as follows:
- Medicine: One of your family members has just been diagnosed with skin cancer, and you want to learn about the disease and the medical treatments available (e.g., currently existing and newly developed). You are also interested in how to protect yourself from the disease.
- Travel: You and your best friend are planning to travel to a place, where you can enjoy one of your favorite sports. You are trying to figure out how to prepare for this adventure and what kinds of information you need.
- Research: You are currently working on a term project. You still have enough time to finish it but you want to work hard on this one and get a good grade because you are really interested in the topic. Now, you are trying to find some good materials, which can provide some background information of the topic area you chose for the term project.
A task in this study was not simply a topic or an information search task. Each task entailed several activities such as problem solving and planning to pursue certain goals.
Research setting
The researcher conducted the study in a controlled environment. The research took place in a laboratory, which was equipped with Dell 3.06GHz Pentium PCs with 512 MB RAM and a 80 GB hard disk running on Windows XP. They also had Microsoft Office 2003 packages and several different kinds of Web browsers including the latest versions of Safari, Firefox, Netscape, and Internet Explorer.
Data analysis
The analysed data included pre- and post-task questionnaires. The questionnaires were tabulated, compiled and analysed with computer program SPSS. Cross-tabulated tables provide 'information on the variation of responses with various demographic and other independent variables that can throw considerable light on the respondents’ underlying characteristics, value structures and thinking processes' (Krathwohl 2004: 372).
Results
Demographic information
Category | Sub-category | Frequency | Percentage (%) |
---|---|---|---|
Age | 18-21 | 3 | 15% |
22-29 | 9 | 45% | |
30-39 | 6 | 30% | |
40+ | 2 | 10% | |
Total | 20 | 100% | |
Sex | Female | 15 | 75% |
Male | 5 | 25% | |
Total | 20 | 100% | |
Academic Status | Undergraduate | 3 | 15% |
Master's | 13 | 65% | |
Doctoral | 2 | 10% | |
Professor | 2 | 10% | |
Total | 20 | 100% | |
Academic Discipline | Social Sciences | 12 | 60% |
Engineering | 3 | 15% | |
Health Sciences | 4 | 20% | |
Natural Sciences | 1 | 5% | |
Total | 20 | 100% |
The subjects in this study had varying backgrounds (see Table 1), including different sexes, academic status (e.g., faculty, doctoral students, master’s students, and undergraduate students), ages, and academic discipline areas (e.g., engineering, social sciences, natural sciences, and health sciences). All participants were regular and experienced Web users.
Multidimensional measures on the tasks
This study was designed to gain a multi-dimensional understanding of the multiple information task behaviour in humans by analysing the dynamic interplays of perceived task attributes, mental efforts, affective states, temporal demands, and performances.
Table 2 describes the average scores of the multiple aspects associated with the multiple information task behaviour in humans in the context of information seeking and retrieval.
Measured variable |
Medicine | Travel | Research | Additional | ||||
---|---|---|---|---|---|---|---|---|
Mean | SD | Mean | SD | Mean | SD | Mean | SD | |
Difficulty | 3.870 | 2.1258 | 4.465 | 1.9680 | 5.690 | 2.3657 | 3.870 | 1.8388 |
Importance | 8.585 | 2.4692 | 5.365 | 2.6039 | 8.550 | 2.0075 | 5.965 | 3.3137 |
Interest | 7.540 | 3.2047 | 6.585 | 3.3611 | 6.150 | 3.1081 | 8.580 | 2.6333 |
Knowledge or familiarity | 4.815 | 3.1179 | 5.800 | 2.5381 | 6.455 | 2.6518 | 7.090 | 2.0619 |
Complexity | 5.830 | 2.6474 | 5.315 | 2.5646 | 7.775 | 2.2148 | 4.950 | 3.0626 |
Mental effort | 4.685 | 2.5236 | 4.715 | 2.2115 | 5.940 | 3.2419 | 4.090 | 2.8011 |
Affective state | 3.025 | 2.6248 | 3.765 | 2.3647 | 4.925 | 3.2973 | 3.275 | 2.8988 |
Temporal demand | 2.780 | 1.8171 | 4.185 | 2.7130 | 4.560 | 2.8840 | 3.205 | 2.3141 |
Performance (success) | 9.010 | 2.0047 | 7.265 | 3.1174 | 7.900 | 2.7719 | 8.950 | 2.3332 |
Performance (satisfaction) | 8.740 | 2.5124 | 7.275 | 3.4127 | 8.030 | 2.8603 | 8.700 | 2.6921 |
Duration | 572.20 | 339.995 | 632.35 | 436.576 | 833.05 | 532.620 | 519.25 | 318.951 |
The results in Table 2 show that the medicine task was considered the least difficult and familiar with the mean scores with 3.8 and 4.8, respectively, and most important with a mean of 8.5. The subjects felt the least emotional and temporal demands while working on the medicine task resulting in the highest levels of success and satisfaction regarding their performances.
The travel task was considered the least important (X=5.3) with the lowest levels of overall success (X=7.2) and satisfaction (X=7.2). The additional task was evaluated as the least difficult (X=3.8) and complex (X=4.9). The subjects also thought that the additional task was most interesting (X=8.5) and familiar (X=7.0), requiring the least level (X=4.0) of cognitive processing to perform the task. The subjects spent the least amount of time in conducting the additional task with a mean duration of 519 seconds.
The research task was considered most difficult (X=5.6) and complex (X=7.7), and the least interesting (X=6.1). It seemed that the subjects invested a high level of mental effort (X=5.9). It was noticed that the levels of emotional state and temporal demand were highly evaluated while the subjects were working on the research task. They also seemed to spend the longest amount of time in finishing the research task (833 sec.).
The additional task was considered most interesting (X=8.5) and familiar (X=7.0) and the least difficult (X=3.8) and complex (X=4.9). It was noticed that the subjects invested less time (519 sec.) and mental effort (X=4.0) on the additional task than the other tasks.
Correlation measures on the tasks
These data were analysed further to see if there were any statistically significant correlations among the variables. The results of this analysis are discussed in the following sections.
Correlation measures: medicine task
Difficulty | Importance | Interest |
Knowledge or Familiarity |
Complexity |
Mental effort |
Affective state |
Temporal demand |
Performance (success) |
Performance (satisfaction) |
Duration | ||
---|---|---|---|---|---|---|---|---|---|---|---|---|
Difficulty | Pearson 'r' | 1 | -0.267 | -0.078 | -0.446* | 0.400 | 0.517* | 0.456* | 0.211 | -0.300 | -0.230 | -0.059 |
Sig. (2-tailed) | 0.255 | 0.743 | 0.049 | 0.081 | 0.020 | 0.043 | 0.371 | 0.199 | 0.329 | 0.806 | ||
Importance | Pearson 'r' | 1 | 0.876** | 0.280 | 0.236 | -0.265 | -0.394 | -0.226 | 0.529* | 0.334 | 0.097 | |
Sig. (2-tailed) | .000 | .231 | .317 | .259 | .086 | .337 | .017 | .150 | .683 | |||
Interest | Pearson 'r' | 1 | .336 | .300 | -.095 | -.088 | .118 | .524* | .365 | .231 | ||
Sig. (2-tailed) | .147 | .198 | .689 | .711 | .621 | .018 | .114 | .326 | ||||
Knowledge / Familiarity | Pearson 'r' | 1 | .157 | -.412 | -.113 | -.060 | .642** | .558* | .112 | |||
Sig. (2-tailed) | .509 | .071 | .635 | .803 | .002 | .011 | .637 | |||||
Complexity | Pearson 'r' | 1 | 0.149 | 0.046 | -0.054 | 0.070 | 0.063 | -0.043 | ||||
Sig. (2-tailed) | 0.531 | 0.848 | 0.821 | 0.771 | 0.792 | 0.858 | ||||||
Mental effort | Pearson 'r' | 1 | 0.639** | 0.449* | -0.446* | -0.532* | 0.360 | |||||
Sig. (2-tailed) | 0.002 | 0.047 | 0.049 | 0.016 | 0.119 | |||||||
Affective State | Pearson 'r' | 1 | 0.532* | -0.472* | -0.473* | 0.331 | ||||||
Sig. (2-tailed) | 0.016 | 0.035 | 0.035 | 0.154 | ||||||||
Temporal demand | Pearson 'r' | 1 | -0.119 | -0.070 | 0.315 | |||||||
Sig. (2-tailed) | 0.617 | 0.769 | 0.176 | |||||||||
Performance (success) | Pearson 'r' | 1 | 0.925** | 0.020 | ||||||||
Sig. (2-tailed) | 0.000 | 0.935 | ||||||||||
Performance (satisfaction) | Pearson 'r' | 1 | -0.147 | |||||||||
Sig. (2-tailed) | 0.536 | |||||||||||
Duration | Pearson 'r' | 1 | ||||||||||
Sig. (2-tailed) |
Table 3 presents the results regarding the correlations of the variables on the medicine task. It was found that among the task attributes, difficulty was negatively associated with knowledge or familiarity (r = -0.446) and importance was positively correlated to interest (r=0.876). Task difficulty had a positive correlation with the degree of mental effort (r=0.517) and affective state (r=0.456). In terms of the behavioural dimension, the degree of success was positively associated with several task attributes including task importance (r=0.529), interest (r=0.524), and knowledge or familiarity (r=0.642). The level of satisfaction was correlated with one task attribute, knowledge or familiarity (r=0.558). In the cognitive dimension, it was noticed that several meaningful relationships existed between mental effort and other variables, including affective state (r=0.639) and temporal demand (r=0.449). Interestingly, the degrees of success (r=-0.446) and satisfaction (r=-0.532) were negatively associated with the level of cognitive processing. It was found that a positive relationship existed between affective state and temporal demand (r=0.532). The emotional degree was negatively associated with the levels of success (r=-0.472) and satisfaction (r=-0.473). A very strong relationship (r=0.925) existed between success and satisfaction.
Correlation measures: travel task
Difficulty | Importance | Interest |
Knowledge or Familiarity |
Complexity |
Mental effort |
Affective state |
Temporal demand |
Performance (success) |
Performance (satisfaction) |
Duration | ||
---|---|---|---|---|---|---|---|---|---|---|---|---|
Difficulty | Pearson 'r'† | 1 | 0.151 | -0.021 | 0.089 | 0.261 | -0.026 | 0.233 | -0.116 | -0.160 | -0.177 | 0.290 |
Sig. (2-tailed) | 0.525 | 0.930 | 0.710 | 0.266 | 0.912 | 0.323 | 0.626 | 0.501 | 0.455 | 0.215 | ||
Importance | Pearson 'r' | 1 | 0.612** | 0.444* | 0.310 | -0.037 | -0.246 | -0.162 | 0.337 | 0.324 | -0.027 | |
Sig. (2-tailed) | 0.004 | 0.050 | 0.183 | 0.875 | 0.295 | 0.496 | 0.146 | 0.164 | 0.909 | |||
Interest | Pearson 'r' | 1 | 0.627** | 0.394 | 0.216 | -0.157 | -0.168 | 0.148 | 0.231 | -0.131 | ||
Sig. (2-tailed) | 0.003 | 0.086 | 0.361 | 0.509 | 0.480 | 0.532 | 0.326 | 0.581 | ||||
Knowledge / Familiarity | Pearson 'r' | 1 | 0.121 | 0.162 | -0.258 | -0.315 | 0.185 | 0.277 | -0.222 | |||
Sig. (2-tailed) | 0.611 | 0.495 | 0.272 | 0.177 | 0.434 | 0.236 | 0.347 | |||||
Complexity | Pearson 'r' | 1 | -0.393 | -0.281 | -0.238 | 0.161 | 0.174 | 0.232 | ||||
Sig. (2-tailed) | 0.087 | 0.230 | 0.312 | 0.498 | 0.464 | 0.324 | ||||||
Mental effort | Pearson 'r' | 1 | 0.641** | 0.458* | -0.501* | -0.347 | 0.115 | |||||
Sig. (2-tailed) | 0.002 | 0.042 | 0.024 | 0.134 | 0.631 | |||||||
Affective state | Pearson 'r' | 1 | 0.604** | -0.752** | -0.719** | 0.292 | ||||||
Sig. (2-tailed) | 0.005 | 0.000 | 0.000 | 0.211 | ||||||||
Temporal demand | Pearson 'r' | 1 | -0.718** | -0.700** | 0.122 | |||||||
Sig. (2-tailed) | 0.000 | 0.001 | 0.609 | |||||||||
Performance (success) | Pearson 'r' | 1 | 0.967** | -0.397 | ||||||||
Sig. (2-tailed) | 0.000 | 0.083 | ||||||||||
Performance (satisfaction) | Pearson 'r' | 1 | -0.371 | |||||||||
Sig. (2-tailed) | 0.107 | |||||||||||
Duration | Pearson 'r' | 1 | ||||||||||
Sig. (2-tailed) | ||||||||||||
* Correlation is significant at the 0.05 level (2-tailed) ** Correlation is significant at the 0.01 level (2-tailed). |
The results in Table 4 indicate that among the task attributes, task importance was positively associated with interest (r=0.612) and knowledge or familiarity (r=0.444). A positive relationship (r=0.627) was found between interest and knowledge or familiarity. It was noticed that mental effort was related to affective state (r=0.641), temporal demand (r=0.458), both positively, and success negatively (r=-0.501). It was found that there was a relationship (r=0.604) between affective state and temporal demand. The degree of emotional state was also negatively associated with overall performance, including success (r=-0.752) and satisfaction (r=-0.719). There was a strong relationship between temporal demand and performance: success (r=-0.718) and satisfaction (r=-0.700). In the travel task, it was also found that the level of success was strongly related to the level of satisfaction.
Correlation measures: research task
Difficulty | Importance | Interest |
Knowledge or familiarity |
Complexity |
Mental effort |
Affective state |
Temporal demand |
Performance (success) |
Performance (satisfaction) |
Duration | ||
---|---|---|---|---|---|---|---|---|---|---|---|---|
Difficulty | Pearson 'r' | 1 | -0.039 | -0.284 | -0.268 | 0.432 | 0.437 | 0.372 | 0.230 | -0.414 | -0.416 | 0.062 |
Sig. (2-tailed) | 0.872 | 0.225 | 0.253 | 0.057 | 0.054 | 0.107 | 0.329 | 0.069 | 0.068 | 0.797 | ||
Importance | Pearson 'r' | 1 | 0.280 | 0.300 | 0.449* | 0.005 | -0.048 | 0.087 | 0.089 | 0.092 | 0.568** | |
Sig. (2-tailed) | 0.231 | 0.199 | 0.047 | 0.982 | 0.840 | 0.715 | 0.709 | 0.700 | 0.009 | |||
Interest | Pearson 'r' | 1 | 0.374 | -0.112 | -0.160 | -0.273 | -0.259 | 0.533* | 0.595** | -0.078 | ||
Sig. (2-tailed) | 0.104 | 0.638 | 0.500 | 0.245 | 0.270 | 0.015 | 0.006 | 0.743 | ||||
Knowledge or familiarity | Pearson 'r' | 1 | 0.023 | 0.046 | -0.050 | -0.294 | 0.238 | 0.284 | -0.163 | |||
Sig. (2-tailed) | 0.922 | 0.847 | 0.835 | 0.208 | 0.312 | 0.225 | 0.492 | |||||
Complexity | Pearson 'r' | 1 | 0.181 | 0.206 | 0.315 | -0.294 | -0.284 | 0.428 | ||||
Sig. (2-tailed) | 0.446 | 0.383 | 0.176 | 0.208 | 0.225 | 0.060 | ||||||
Mental effort | Pearson 'r' | 1 | 0.680** | 0.669** | -0.638** | -0.615** | 0.197 | |||||
Sig. (2-tailed) | 0.001 | 0.001 | 0.002 | 0.004 | 0.405 | |||||||
Affective state | Pearson 'r' | 1 | 0.509* | -0.751** | -0.682** | 0.259 | ||||||
Sig. (2-tailed) | 0.022 | 0.000 | 0.001 | 0.270 | ||||||||
Temporal demand | Pearson 'r' | 1 | -0.563** | -0.596** | 0.483* | |||||||
Sig. (2-tailed) | 0.010 | 0.006 | 0.031 | |||||||||
Performance (success) | Pearson 'r' | 1 | 0.941** | -0.233 | ||||||||
Sig. (2-tailed) | 0.000 | 0.323 | ||||||||||
Performance (satisfaction) | Pearson 'r' | 1 | -0.279 | |||||||||
Sig. (2-tailed) | 0.233 | |||||||||||
Duration | Pearson 'r' | 1 | ||||||||||
Sig. (2-tailed) | ||||||||||||
* Correlation is significant at the 0.05 level (2-tailed). ** Correlation is significant at the 0.01 level (2-tailed) |
Table 5 shows that among the task attributes, importance was correlated to complexity (r=0.449) and duration (r=0.568). There was a relationship between interest and performance: success (r=0.533) and satisfaction (r=0.595). It was noticed that mental effort was associated with several variables including affective state (r=0.680), temporal demand (r=0.669), success (r=-0.638), and satisfaction (r=-0.615). Affective state was related to temporal demand positively (r=0.509) and performance negatively (success, r=-0.751; satisfaction, r=-0.682). The degree of temporal demand was positively associated with task duration (r=0.483) and negatively related to both success (r=-0.563) and satisfaction (r=-0.596). A noticeable relationship (r=0.941) existed between success and satisfaction.
Correlation measures: additional task
Difficulty | Importance | Interest |
Knowledge or familiarity |
Complexity |
Mental effort |
Affective state |
Temporal demand |
Performance (success) |
Performance (satisfaction) |
Duration | ||
---|---|---|---|---|---|---|---|---|---|---|---|---|
Difficulty | Pearson 'r' | 1 | 0.441 | 0.257 | 0.111 | 0.562** | 0.332 | 0.288 | 0.398 | -0.061 | 0.095 | 0.318 |
Sig. (2-tailed) | 0.051 | 0.275 | 0.641 | 0.010 | 0.152 | 0.218 | 0.082 | 0.798 | 0.691 | 0.171 | ||
Importance | Pearson 'r' | 1 | 0.465* | 0.399 | 0.241 | 0.241 | 0.267 | 0.412 | -0.020 | 0.116 | 0.306 | |
Sig. (2-tailed) | 0.039 | 0.082 | 0.306 | 0.305 | 0.256 | 0.071 | 0.934 | 0.626 | 0.190 | |||
Interest | Pearson 'r' | 1 | 0.012 | 0.473* | 0.263 | 0.279 | 0.152 | -0.146 | -0.108 | 0.482* | ||
Sig. (2-tailed) | 0.960 | 0.035 | 0.262 | 0.233 | 0.523 | 0.539 | 0.650 | 0.031 | ||||
Knowledge or familiarity | Pearson 'r' | 1 | 0.052 | 0.025 | 0.124 | 0.139 | 0.279 | 0.363 | 0.086 | |||
Sig. (2-tailed) | 0.828 | 0.918 | 0.602 | 0.559 | 0.234 | 0.116 | 0.720 | |||||
Complexity | Pearson 'r' | 1 | 0.014 | -0.075 | 0.234 | 0.123 | 0.260 | 0.116 | ||||
Sig. (2-tailed) | 0.952 | 0.753 | 0.321 | 0.605 | 0.268 | 0.625 | ||||||
Mental effort | Pearson 'r' | 1 | 0.737** | 0.388 | -0.548* | -0.448* | 0.580** | |||||
Sig. (2-tailed) | 0.000 | 0.091 | 0.012 | 0.048 | 0.007 | |||||||
Affective state | Pearson 'r' | 1 | 0.153 | -0.611** | -0.530* | 0.240 | ||||||
Sig. (2-tailed) | 0.521 | 0.004 | 0.016 | 0.309 | ||||||||
Temporal demand | Pearson 'r' | 1 | 0.041 | 0.142 | 0.464* | |||||||
Sig. (2-tailed) | 0.864 | 0.552 | 0.039 | |||||||||
Performance (success) | Pearson 'r' | 1 | 0.943** | -0.070 | ||||||||
Sig. (2-tailed) | 0.000 | 0.770 | ||||||||||
Performance (satisfaction) | Pearson 'r' | 1 | 0.058 | |||||||||
Sig. (2-tailed) | 0.809 | |||||||||||
Duration | Pearson 'r' | 1 | ||||||||||
Sig. (2-tailed) | ||||||||||||
* Correlation is significant at the 0.05 level (2-tailed). ** Correlation is significant at the 0.01 level (2-tailed) |
In Table 6, for the additional task, task complexity was associated with both difficulty (r=0.562) and interest (r=0.473). It was found that perceived task interest was related to importance (r=0.465) and time spent (r=0.482). It was noticed that the degree of mental effort was associated with several variables including affective state (r=0.737), performance (success, r=-0.548; satisfaction, r=-0.448), and duration (r=0.580). Affective state was also negatively related to performance (success, r=-0.611; satisfaction, r=-0.530). A relationship existed between the level of temporal demand and the time spent on the task (r=0.464). In the additional task, the level of success was also strongly related to the level of satisfaction (r=0.943).
Discussion and conclusion
Multiple information task behaviour in humans consists of multiple components. The success of such activities depends on how people bring those different elements into a relationship that will ensure efficiency and harmony.
The correlations between the measures were examined at the significant levels of .01 and .05. Table 7 shows the dynamic interplays of the components of human multiple information task interaction on the Web, employing a matrix to represent/visualise the numeric data in a meaningful way.
Statistically significant relationships exist among the perceived attributes of the information tasks. Interest is related with importance (r=0.88, medicine task; r=0.61, travel task; r=0.47, additional task). knowledge or familiarity is associated with both importance (r=0.44, travel task) and interest (r=0.63, travel task). At the same time, knowledge or familiarity is negatively correlated with difficulty (r=-0.45, medicine task). Complexity is associated with multiple task attributes, including difficulty (r=0.56, additional task), importance (r=0.45, research task), and interest (r=0.47, additional task). Interesting information tasks are considered important. When people determine that they have some experience or are familiar with a certain information task, they think the task is more important and interesting and less challenging. A complex information task is perceived as more difficult, important, and interesting.
Both mental effort (r=0.52, medicine task) and affective state (r=0.46, medicine task) are correlated with the perceived degree of task difficulty. Negative perceptions toward an information task (e.g., higher task difficulty) led people to try harder but this feeling counteracts and increases stress and frustration. This might be caused by low self-confidence in performing the task.
In terms of performance, task importance (r=0.53, medicine task), interest (r=0.52, medicine task; r=0.53, research task), and knowledge or familiarity (r=0.64, medicine task) are all significantly correlated with success. Among them, only task interest (r=0.60, research task) and knowledge or familiarity (r=0.56, medicine task) are significantly related to satisfaction. There is a significant correlation between duration and task importance (r=0.57, research task). Duration is also correlated with task interest (r=0.48, additional task). It seems that people perform better with higher satisfaction when faced with more important, interesting, and familiar information tasks. In addition, they tend to spend more time on interesting and important tasks to complete them.
It should be noticed that there are dynamic correlations among other measures, including mental effort, affective state, temporal demand, performance, and duration, for most of the information tasks.
Mental effort is positively associated with multiple measures, such as affective state (r=0.64, medicine task; r=0.64, travel task; r=0.68, research task; r=0.74, additional task), temporal demand (r=0.45, medicine task; r=0.46, travel task; r=0.67, research task), and duration (r=0.58, additional task). At the same time, the cognitive measure is negatively correlated with performance in terms of success (r=-0.45, medicine task; r=-0.50, travel task; r=-0.64, research task; r=-0.55, additional task) and satisfaction (r=-0.53, medicine task; r=-0.62, research task; r=-0.45, additional task).
Another measure, which is related to multiple dimensions, is affective state. It was found that a statistically significant relationship exists between affective state and temporal demand (r=0.53, medicine task; r=0.60, travel task; r=0.51, research task). Affective state is also negatively correlated with performance, with respect to success (r=-0.47, medicine task; r=-0.75, travel task; r=-0.75, research task; r=-0.61, additional task) and satisfaction (r=-0.47, medicine task; r=-0.72, travel task; r=-0.68, research task; r=-0.53, additional task).
A negative correlation exists between temporal demand and performance in terms of success (r=-0.72, travel task; r=-0.56, research task) and satisfaction (r=-0.70, travel task; r=-0.60, research task). In addition, temporal demand is positively correlated with duration (r=0.48, research task; r=0.46, additional task). There is a highly significant correlation between success and satisfaction (r=0.93, medicine task; r=0.97, travel task; r=0.94, research task; r=0.94, additional task).
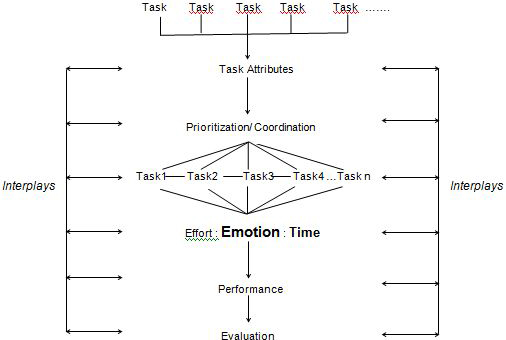
Figure 2: Model revisited
With the resulting data, the theoretical model of human prioritising and coordinating information behaviour was revisited. Figure 2 indicates those with higher cognitive effort experienced more stress and frustration and higher temporal demand, resulting in lower levels of performance, even though they spent more time finishing the information task. It seems that cognitive, emotional, temporal, and behavioural aspects are closely related to each other across the tasks. When people consider a task to be demanding (e.g., higher difficulty or complexity), they often become frustrated. In this case, even though they try harder to solve the problem for a longer time period, their negative emotional state prevents them from performing the task successfully. In other words, high degrees of temporal demand and a negative emotional state led people to perform poorly even though they invest more mental effort on the task. Our feelings and emotions play a key role in our ability to deal with a situation where multiple information tasks need to be done within time limits.
Humans have inhabited dynamic, volatile information environments since the advent of the Web. As Web information environments become more complex and dynamic, people often find themselves faced with multiple information tasks or goals while interacting with Web information technologies. Our adaptation occurs from our ability to process information effectively and adapt our information behaviour accordingly. Despite the values, current studies of human-information interaction have limitations in explaining our adaptive information behaviour in dynamic and complex Web information environments.
Interest | Knowledge or familiarity |
Complexity | Mental effort |
Affective state |
Temporal demand |
Performance (success) |
Performance (satisfaction) |
Duration | |
---|---|---|---|---|---|---|---|---|---|
Difficulty | -.446*[MT] | .562*[AT] | .517*[MT] | .456*[MT] | |||||
Importance |
.876***[MT] .612**[TT] .465*[AT] |
.444*[TT] | .449*[RT] | .529*[MT] | .568*[RT] | ||||
Interest | .627**[TT] | .473*[AT] |
.524*[MT] .533*[RT] |
.595*[RT] | .482*[AT] | ||||
Knowledge or familiarity | .642**[MT] | .558*[MT] | |||||||
Mental effort |
.639**[MT] .641**[TT] .680**[RT] .737**[AT] |
.449*[MT] .458*[TT] .669**[RT] |
-.446*[MT] -.501*[TT] -.638**[RT] -.548*[AT] |
-.532*[MT] -.615**[RT] -.448*[AT] |
.580*[AT] | ||||
Affective state |
.532*[MT] .604**[TT] .509*[RT] |
-.472*[MT] -.752**[TT] -.751**[RT] -.611**[AT] |
-.473*[MT] -.719**[TT] -.682**[RT] -.530*[AT] |
||||||
Temporal demand |
-.718**[TT] -.563*[RT] |
-.700**[TT] -.596*[RT] |
.483*[RT] .464*[AT] |
||||||
Performance (success) |
.925***[MT] .967***[TT] .941***[RT] .943***[AT] |
||||||||
Note 1: * means moderate correlation; ** strong correlation; *** very strong correlation Note 2: MT stands for Medicine task; TT, Travel task; RT, Research task; AT, Additional task |
The understanding of human multiple information task behaviour can be utilized as a conceptual base to design information systems which support efficient and effective human-information and human-computer interactions in complex and dynamic information seeking and retrieval environments.
From the system design point of view, it would be advantageous for systems to support a user’s individuality to close the gaps in performance differences. Adaptive (also called personalised) information systems are designed to deal with the fact that users are individuals, taking into account individual features such as goals/tasks, knowledge, background, Web experience, preferences and interests (Brusilovsky, 2001). To build such systems to be human-friendly, system designers first need to understand the dynamic interplays of humans, information, tasks, and systems.
Researchers in the field of human-information interaction and human-computer interaction can incorporate the concept of human multiple task performance to design adaptive user interfaces. Creating effective user interfaces requires knowledge of how people actually deal with multiple information task situations physically, cognitively, and emotionally under dynamic and complex circumstances. The results of this study can be employed as a theoretical foundation for designing human-friendly, adaptive user interfaces, which function as intelligent and affective central mechanisms and help users prioritise, monitor and coordinate their needs/tasks/goals effectively and efficiently.
This study identifies the significance of the emotional state of the information seeker as a factor in dynamic information seeking and retrieval contexts and enlightens the existing areas of human information interaction. This exploratory study will contribute to the growth of knowledge in information science.
About the author
Minsoo Park is a senior researcher of Korea Institute of Science and Technology Information, Seoul, Korea. Her main areas of research interest are human-information interaction and human-computer interaction. She can be contacted at mspark7@gmail.com.