Assessing user needs of Web portals: a measurement model
Misook Heo
Duquesne University, 600 Forbes Avenue, Pittsburgh, PA 15282, USA
Introduction
With the increase of data mining on the Internet, Web portals quickly became one of the most promising Web services. Web portals not only provided users with integrated access to dynamic content from various information sources, but also made it possible for users to personally tailor their information retrieval (Tojib et al. 2008).
As more Web portals became available, studies on the factors that influence user attraction and retention started to receive more attention (Lee et al. 2012; Liao et al. 2011; Lin et al. 2005; Presley and Presley 2009; Tojib et al. 2008). Until recently, most user studies about Web portals have been in the field of business. This may be because business Web portals were the forerunners of Web portal services, and because the more users visit a Web portal, the more revenue the business makes from advertisements (Ha 2003). As more institutions adopt Web portals, however, studies are expanding into diverse domains such as education, government and libraries. With the high cost of portal implementation and management (Bannan 2002), user studies have become more critical for portal success (Liao et al. 2011; Liu et al. 2009).
While there are numerous research studies regarding business portal users, there is limited user-centred literature about domain-specific, non-commerce Web portals, especially cultural heritage portals. When a cultural heritage portal cannot meet potential users’ needs, the portal’s usage will decline and its educational impact will deteriorate.
The goal for this paper is to examine the user needs of cultural heritage portals. This line of study will provide researchers and practitioners with a research-based user study framework, which will help them better identify the underlying construct of user demands that will eventually build and maintain successful Web portals.
Web portal
While there are numerous definitions for Web portals, a general consensus seems to be that Web portals are a personalised, Internet-based, single access point to varying, divergent sources (Al-Mudimigh et al. 2011; Liu et al. 2009; Presley and Presley 2009; Telang & Mukhopadhyay 2005; Yang et al. 2005; Watson and Fenner 2000). As the Web began to emerge as a platform that harnessed collective intelligence and distributed it with lightweight programming models (Anderson 2007), Web portals became constant update services that allowed users to consume, remix and multiply data and services, emphasising collaborative information sharing.
Web portals also function as an authenticated access point with a single user interface. With this personalisation, each user becomes a unique audience of their own distinct content (Gilmore and Pine 2000; Kobsa et al. 2001). Individuals perform the gatekeeping function by customising their information preferences (Sundar and Nass 2001). This personalisation feature may promote a greater sense of ownership of portal content (Kalyanaraman and Sundar 2006).
Cultural Heritage Portals
Cultural heritage portals offer digital duplications of artefacts. As with other portals, cultural heritage portals often provide users with distributed artefacts by multi-institution collaboration (Cha and Kim 2010; Concordia et al. 2010; Gibson et al. 2007; Tanackoviæ and Badurina 2008), the ability to personalise their experiences (Giaccardi 2006; Yoshida et al. 2010), and to contribute their knowledge or add content (Cox 2007; Giaccardi 2006; Farber and Radensky 2008; Moirez 2012; Pruulmann-Vengerfeldt and Aljas 2009; Timcke 2008; Yakel 2006). With the realisation that users of archives are also changing from researchers to non-researchers (Adams 2007; Huvila 2008), discussions on strategies to engage users are rising (Cox 2007; Durbin 2008; Farber and Radensky 2008; Huvila 2008; Pruulmann-Vengerfeldt and Aljas 2009), and understanding user demands and their motivations for using cultural heritage materials is recognised as a critical factor of cultural heritage portals (Pruulmann-Vengerfeldt and Aljas 2009).
Europeana, one of the best-known cultural heritage portals, exhibits the aforementioned cultural heritage portal trends. With the goal of maximizing public access to the widest range of heritage materials from trusted providers throughout Europe,it functions as a gateway to distributed European cultural heritage resources, and aggregates over fifteen million digital items from 1500 museums, archives, libraries, and other similar institutions in Europe. Users can access the portal through one of twenty-eight official languages of the European Union and personalise their experience with ‘My Europeana’. At the time of designing, Europeana used techniques such as user profiles, log-file analysis, surveys, and focus groups to meet user needs (Cousins 2009). In addition, user engagement is a goal of the current strategic plan which will allow users to contribute to the content of the portal in various ways such as uploading simple media objects along with their descriptions, annotating existing objects, or enriching existing descriptions (Aloia et al. 2011). Through increased user engagement, it encourages user interest and loyalty (Aloia et al. 2011). Currently, users can submit opinions, and user-oriented service has been stressed through the EuropeannaConnect project. The Europeana use patterns from September 2010 to August 2011 show around three million unique users, and over half of them (one per cent of heavy users and fifty six per cent of normal users) have used Europeana with a measurable level of engagement (Clark et al. 2011).
Trove: Australia in Pictures and Moving Here also exhibit several of the cultural heritage portal trends. In June 2012, Trove: Australia in Pictures integrated Picture Australia, a portal launched a decade ago with the goal to serve as a single access point to discover Australian heritage as documented in pictures. Trove: Australia in Pictures currently aggregates about 1.8 million digitised images relating to Australia from over seventy cultural agencies in Australia. In response to changing expectations of users, it provides data engagement features that allow users to personalise their experiences, and social engagement features that allow users to contribute their knowledge and add contents (Holley 2010). While group-specific statistics have not been published, Trove: Australia in Pictures has documented over 4.8 million activities each month consistently since the complete integration of Picture Australia with Trove.
With the aim of encouraging minority ethnic groups’ involvement in recording and documenting their own history of migration, Moving Here connects to over 200,000 digitised resources regarding the last 200 years of UK migration history from a consortium of thirty archives, libraries and museums and houses over five hundred digital stories (Flinn 2010). Although Moving Here does not provide individual users with the ability to personalise their experience, users can contribute personally owned images or digital stories. In fact, about half of the testimonies of migrants were reported to be from voluntary contributions (Alain and Foggett 2007).
Theoretical background
There seem to be few studies that examined user needs of cultural heritage portals, partly because of their contemporary nature. Studies examining the factors influencing user attraction and retention for other Web portals, however, often utilise a few common theoretical frameworks, such as data quality, service quality and technology acceptance models. This section reviews these frameworks to understand their characteristics.
Data quality model
Data quality explains the extent to which data meet user requirements in a given context (Cappiello et al. 2004; Knight and Burn 2005; Strong et al. 1997). Data quality is therefore relative; acceptable data quality varies by the context through which it is being measured (Tayi and Ballou, 1998) and implies that dimensions of data quality need to be adjusted to support specific user needs in the context of the individual’s interests.
Ever since Wang and Strong (1996) proposed a multi-dimensional framework of data quality measurement, many researchers have made efforts to define a basic set of data quality dimensions. While the definitions of dimensions and measures have long been debated (Batini et al. 2009; Cappiello et al. 2004), possibly in part because of the contextual nature of data quality, the dimensions that are often defined include: intrinsic, contextual, representational, and accessibility (e.g., Caro et al. 2008; Haug et al. 2009; Wang and Strong, 1996). Recently, the International Organization for Standardization (ISO) and the International Electrotechnical Commission (IEC) jointly provided a data quality model, ISO/IEC 25012. The model defines fifteen characteristics of general data quality from two different perspectives: inherent and system dependent (ISO/IEC 2008).
The study of data quality is multidisciplinary (Madnick et al. 2009). While data quality studies originated in the context of information systems, they have been pursued by various disciplines (e.g., Lee et al. 2002). Table 1 lists a selection of data quality studies and their recommended data quality dimensions.
Study | Research focus | Suggested dimensions and sub-dimensions |
---|---|---|
Caro et al. (2008) | Proposal for a generic Web portal data quality model |
Intrinsic: accuracy, objectivity, believability, reputation, currency, expiration, traceability, duplicates (the extent to which delivered data contains duplicates) Operational: accessibility, security, interactivity, availability, customer support, ease of operation, response time Contextual: applicability, completeness, flexibility, novelty, reliability, relevancy, specialisation, timeliness, validity, value-added Representational: interpretability, understandability, concise representation, consistent representation, amount of data, attractiveness, documentation, organisation |
Haug et al. (2009) | Creation of a classification model for the evaluation of enterprise resource planning system data |
Intrinsic: completeness, unambiguousness, meaningfulness, correctness Accessibility: access rights, storage in enterprise resource planning system, representation barriers Data usefulness: relevance, value-adding |
ISO/IEC (2008) | Standards for software product quality requirements and evaluation |
Inherent: accuracy, completeness, consistency, credibility, currentness System dependent: availability, portability, recoverability Inherent and system dependent: accessibility, compliance, confidentiality, efficiency, precision, traceability, understandability |
Katerattanakul and Siau (2008) | Development of an instrument measuring factors affecting data quality of personal Web portfolios |
Presentation quality: use and organisation of visual settings and typographical features, consistent presentation, attractiveness Contextual quality: provision of author’s information Accessibility quality: navigational efficiency, workable and relevant hyperlinks |
Wang and Strong (1996) and Strong et al. (1997) | Recommendations for information systems professionals to improve data quality from the data consumers’ perspective |
Intrinsic: accuracy, objectivity, believability, reputation Accessibility: accessibility, access security Contextual: relevancy, value-added, timeliness, completeness, amount of data Representational: interpretability, ease of understanding, concise representation, consistent representation |
Service quality model
The conceptualisation of service quality has been debated over the past three decades (Brady and Cronin 2001; Ekinci 2002; Martínez and Martínez 2010; Parasuraman et al. 1985, 1988, 1991). These divergent views led to many service quality models including Grönroos (1984) and Parasuraman et al. (1985, 1988, 1991) proposing their conceptualisation models (for reviews, see Prakash and Mohanty 2013; Seth and Deshmukh 2005). In reviewing the service quality literature, several points became apparent: 1) there is no consensus on a conceptual definition 2) there is no agreement on an operational definition to measure service quality, 3) Parasuraman’s conceptualisation model dominates in the literature.
Parasuraman et al. (1985) assert that the service is inherently intangible and heterogeneous, and separation of production from consumption is not possible; service quality needs to be assessed on the basis of customer perceptions. Their conceptualisation claims that service quality is a function of the gap between expectation and perceived performance along the various quality dimensions (Gap analysis). They subsequently proposed the SERVQUAL, a multidimensional instrument consisting of five universal constructs: tangibles, reliability, responsiveness, assurance and empathy (Parasuraman et al. 1988). Since then, the SERVQUAL has received much attention from researchers and has been widely applied by practicing managers. One of the service quality models that is often used in comparison with SERVQUAL is the importance-performance analysis model. Originally developed by Martilla and James (1977), the importance-performance analysis’ conceptualisation of quality is determined as a function of the gap between customer perceptions of performance and the importance of the service attributes. Many importance-performance analysis researchers, however, have used the concepts of importance and expectation interchangeably, possibly because of the similarity between importance-performance analysis and SERVQUAL (Oh 2001).
Though popular, the SERVQUAL model has received a number of criticisms from theoretical and operational perspectives (for reviews, see Buttle 1996; Ladhari 2009). Among the criticisms are its adoption of the expectancy disconfirmation framework (satisfaction is a function between expectations and the disconfirmation from the expectations) and the applicability as a universal construct. Cronin and Taylor (1992) questioned the expectancy disconfirmation framework of SERVQUAL as the primary measure of service quality. They argued that the disconfirmation of the expectations paradigm explains levels of satisfaction, not service quality, and SERVQUAL does not distinguish service quality from satisfaction well. While customer satisfaction and perceived service quality are conceptually different constructs, researchers have acknowledged that the two are strongly related (Lin et al. 2005; Telang and Mukhopadhyay 2005) and often treated similarly in practice (Zeithaml 2000). Though Parasuraman (1988) suggests that the five constructs of SERVQUAL are universal, critics also argued that those service quality constructs, including the number of dimensions and items within each factor, are contextualised; therefore, the weight and number of dimensions may actually be unique to each type of service industry (Buttle, 1996; Cronin and Taylor, 1992; Gounaris and Dimitriadis 2003; Ladhari 2009; Seth and Deshmukh 2005). The appropriateness of its premise and stability of dimensions needs to be assured when adapting SERVQUAL (Landrum et al. 2009), so that it can better measure service quality within a particular service industry.
Even with criticisms, SERVQUAL has been used most widely in assessing service quality; has been extended to the context of electronic businesses as E-S-QUAL (core e-business service quality scale) and E-RecS-QUAL (the e-recovery service quality scale) (Parasuraman et al. 2005); and has been widely tested in various online services, such as e-commerce, e-government, e-learning, and library systems (Table 2).
Study | Research focus | Suggested dimensions |
---|---|---|
Moraga et al. (2004) | Proposal for a generic Web portal service quality model |
Tangibles (i.e. software/hardware requirements) Reliability Responsiveness Assurance Empathy Data quality |
Liu et al. (2009) | Identification of an instrument to measure the service quality of general portals |
Usability Privacy and security Adequacy of information Appearance |
Gounaris & Dimitiadis (2003) | Identification of quality dimensions from the users' perspective of business to consumer portals |
Customer care and risk reduction benefit Information benefit Interaction facilitation benefit |
Kuo et al. (2005) | Identification of user-perceived e-commerce portal quality dimensions |
Empathy Ease-of-use Information quality Accessibility |
April & Pather (2008) | Investigation of the information systems version of the SERVQUAL instrument in the Small and Medium Enterprises e-Commerce sector |
Credibility Availability Expertise Supportiveness Tangibles |
Devaraj et al. (2002) | Measurement of consumer satisfaction and preference with the e-commerce channel |
Empathy Reliability |
Siadat et al. (2008) | Ranking the dimensions of service quality affecting the customers’ expectation in online purchasing in Iran |
Tangibles Assurance Reliability Responsiveness Empathy |
Lee et al. (2011) | Identification of factors influencing the willingness to adopt e-Government services |
Timeliness Responsiveness |
Tan et al. (2010) | Development and testing of an e-Government service quality model |
Tangibles Assurance Reliability Responsiveness Empathy |
Udo et al. (2011) | Measurement of student perceived e-learning quality |
Tangibles Assurance Responsiveness Empathy |
Wang et al. (2010) | Validation of an Information Systems adapted SERVQUAL model in the context of an e-learning system use |
Tangibles Assurance Reliability Responsiveness Empathy |
Landrum & Prybutok (2004) | Proposed model of library success |
Tangibles Reliability Responsiveness, assurance, and empathy |
The technology acceptance model
The technology acceptance model is a framework designed to explain the factors of technology acceptance in a theoretically justified way and to predict the adoption of a new technology by users (Davis et al. 1989; Laio et al. 2011; Turner et al. 2010). This model assumes that a user’s internal beliefs, attitudes and intentions are critical factors of technology acceptance and future technology use (Davis et al., 1989). The original model indicates four internal variables: perceived usefulness, perceived ease-of-use, attitude toward use and behavioural intention to use (Davis et al., 1989; Gournaris and Dimitiadis 2003). Among them, perceived usefulness and perceived ease-of-use were recognised as the two primary determinants of behavioural intention and usage (Davis, 1989; Davis et al., 1989).
Technology acceptance model has proven to have a good predictive validity in its initial adoption and in its continued use of information technology, and is one of the most influential models for explaining user’s technology acceptance (Karahanna et al. 2006; Presley and Presley 2009). Some researchers believed, however, that technology acceptance model’s focus was only on functional or extrinsic motivational drivers, such as usefulness and ease-of-use (Lee et al. 2005). This suggests that to be able to better predict the adoption of a new technology, technology acceptance model needs to be adapted to include intrinsic motivational factors, such as perceived enjoyment.
Technology acceptance model has been expanded by researchers (Gounaris and Dimitriadis 2003), and has resulted in the addition of dimensions such as perceived enjoyment (or playfulness) and actual use. These expanded versions of technology acceptance model can predict adoption of diverse online systems such as e-commerce, e-learning or Web-based information systems and Web portals. Table 3 lists a few example studies in these fields.
Study | Research focus | Suggested dimensions |
---|---|---|
Liao et al. (2011) | Identification of factors influencing the intended use of general Web portals |
Habit --> Behavioural intention to use; Habit --> Perceived usefulness Perceived enjoyment/playfulness -->Behavioural intention to use Perceived usefulness -->Behavioural intention to use Perceived attractiveness --> Perceived usefulness; Perceived attractiveness --> Perceived enjoyment/playfulness; Perceived attractiveness --> (negatively) Psychic cost perceptions Psychic cost perceptions --> (negatively) Behavioural intention to use |
Presley & Presley (2009) | Identification of factors influencing students’ acceptance and use of university intranet portals |
Perceived ease-of-use --> Perceived usefulness; Perceived ease-of-use --> Attitude towards using Perceived usefulness --> Behavioural intention to use; Perceived usefulness --> Attitude towards using Attitude towards using --> Behavioural intention to use Perceived enjoyment/playfulness --> Attitude towards using Behavioural intention to use --> Use (actual) |
van der Heijden (2003) | Identification of a person’s usage behaviour on generic Web portals |
Perceived attractiveness --> Perceived ease-of-use; Perceived attractiveness --> Perceived enjoyment/playfulness; Perceived attractiveness --> Perceived usefulness Perceived usefulness --> Attitude towards using; Perceived usefulness --> Behavioural intention to use Perceived ease-of-use --> Perceived enjoyment/playfulness; Perceived ease-of-use --> Perceived usefulness; Perceived ease-of-use --> Attitude towards using; Perceived ease-of-use --> Behavioural intention to use Perceived enjoyment/playfulness --> Attitude towards using Attitude towards using --> Behavioural intention to use Behavioural intention to use --> Use (actual) |
Klopping & McKinney (2004) | Identification of factors influencing actual use of online shopping activities |
Perceived usefulness --> Behavioural intention to use Perceived ease-of-use --> Behavioural intention to use Behavioural intention to use --> Use (actual) |
Cheung & Sachs (2006) | Identification of factors to predict actual Blackboard usage |
Learning goal orientation --> Perceived enjoyment/playfulness Perceived enjoyment/playfulness --> Application specific self-efficacy; Perceived enjoyment/playfulness --> Perceived ease-of-use; Perceived enjoyment/playfulness --> Perceived usefulness Application specific self-efficacy --> Perceived ease-of-use; Application specific self-efficacy --> U Perceived ease-of-use --> Behavioural intention to use Perceived usefulness --> Behavioural intention to use |
Lee et al. (2005) | Identification of motivators for explaining students’ intention to use an Internet-based learning medium |
Perceived usefulness --> Behavioural intention to use; Perceived usefulness --> Attitude towards using Perceived ease-of-use --> Perceived usefulness; Perceived ease-of-use --> Attitude towards using; Perceived ease-of-use --> Perceived enjoyment/playfulness Attitude towards using --> Behavioural intention to use Perceived enjoyment/playfulness --> Attitude towards using; Perceived enjoyment/playfulness --> Behavioural intention to use |
Yi & Hwang (2003) | Identification of factors to predict the use of Web-based class management systems, e.g., Blackboard |
Learning goal orientation --> Application specific self-efficacy Perceived enjoyment/playfulness --> Perceived usefulness; Perceived enjoyment/playfulness --> Perceived ease-of-use; Perceived enjoyment/playfulness --> Application specific self-efficacy Perceived usefulness --> Behavioural intention to use Perceived ease-of-use --> Behavioural intention to use Application specific self-efficacy --> Perceived ease-of-use; Application specific self-efficacy --> Use (actual) Behavioural intention to use --> Use (actual) |
Moon & Kim (2001) | Extending and empirically validating technology acceptance model for the WWW context |
Perceived ease-of-use --> Perceived playfulness; Perceived ease-of-use --> Attitude towards using; Perceived ease-of-use --> Perceived usefulness Perceived playfulness --> Attitude towards using; Perceived playfulness --> Behavioural intention to use Perceived usefulness --> A; Perceived usefulness --> Behavioural intention to use Attitude towards using --> Behavioural intention to use Behavioural intention to use --> Use (actual) |
Overall, while data quality and service quality frameworks work for quality assessment, technology acceptance model does not directly measure quality. Users’ technology readiness, however, influences their expectations of Websites, and their acceptance may influence the process used in judging electronic service quality (Parasuraman et al. 2005). These three frameworks clearly complement each other and a combination of the three is proposed to assess the user needs of cultural heritage portals.
Research methods
Instrument
Portals are comprehensive information systems, and the cultural heritage portal is not an exception. Considering the volume of the data, the wide spectrum of services that the portal offers, and the fact that the cultural heritage portal is relatively new to its users, it was reasoned that one framework alone might not be sufficient to measure user needs of cultural heritage portals. With this reasoning, three widely used theoretical frameworks in the field of portal research were adapted in framing the measurement model; data quality and service quality models were selected to provide measure of quality, and technology acceptance model was chosen to provide measure of user adoption.
Researchers recommend that dimensions of data quality models need to be modified to support user needs in the context in which the user’s particular interests resides. Since the majority of the available scales were for business information systems, the current research borrowed Caro et al.’s (2008) scale, which was designed to measure the data quality of generic Web portals. Researchers of the service quality model also believe that the scale needs to be adapted to fit the study’s context. This study adapted Moraga et al.’s (2004) scale, which was developed to measure Web portal service quality. For technology acceptance model, researchers recommend intrinsic motivational factors such as user enjoyment. Two scales that contain a user enjoyment dimension were combined and adapted for this study: van der Heijden’s (2003) scale for generic portals and Moon and Kim’s (2001) scale for the Web.
To strengthen its face validity and content validity, the instrument was reviewed by five independent technology and content experts. During this process, some of the dimensions and questions were recognised as redundant. The resulting instrument consisted of eleven dimensions, measured by seventy-nine, six-point Likert scale questions (i.e. data quality - four dimensions of intrinsic, contextual, representational and operational measured by twenty-nine questions; service quality - four dimensions of reliability, responsiveness, assurance and empathy measured by thirty-two questions; and technology acceptance model – three dimensions of perceived ease-of-use, perceived usefulness and perceived enjoyment measured by eighteen questions).
In finalising the research instrument, the statement 'I agree to provide an honest answer to each question that follows' was added to promote honest responses. While cheating occurs to a certain degree, a simple honour code statement has been proved to eliminate almost all cheating (Ariely 2008).
Participants
Efforts were made to recruit typical users of cultural heritage portals, including general users, academic users, expert researchers and professional users (e.g., librarians, archivists, curators) (Purday 2009). Over 400 U.S. organisations were asked to help distribute the research information.
To obtain a 95% confidence level with an approximated population size larger than 20,000, a minimum sample size of 377 was expected. The online survey was closed when a total of 393 complete responses were collected. The descriptive characteristics of the participants are listed in Table 4.
Count | Percentage | |
---|---|---|
Number of respondents | 393 | 100.00 |
Sex | ||
Female | 318 | 80.92 |
Male | 75 | 19.18 |
Age | ||
Less than 25 (youngest reported = 19) | 50 | 12.72 |
26 to 29 | 67 | 17.05 |
30 to 34 | 56 | 14.25 |
35 to 39 | 49 | 12.47 |
40 to 44 | 44 | 11.20 |
45 to 49 | 27 | 6.87 |
50 to 54 | 81 | 20.61 |
55 or older | 19 | 4.83 |
Role | ||
Academic user (Faculty, or teacher or instructor, and student) | 105 | 26.72 |
Expert researcher | 6 | 1.53 |
Professional user (Librarian, archivist, curator, etc.) | 246 | 62.60 |
General user | 36 | 9.16 |
Highest degree earned | ||
High school diploma or equivalent | 10 | 2.54 |
Some college, no degree | 17 | 4.33 |
Associate’s degree | 3 | 0.76 |
Bachelor’s degree | 63 | 16.03 |
Master’s degree | 285 | 72.52 |
Specialist degree | 2 | 0.51 |
Doctoral degree | 13 | 3.31 |
Cultural heritage portal experience | ||
Have never used | 211 | 53.69 |
Have used but abandoned | 20 | 5.09 |
Irregular user | 116 | 29.52 |
Regular user (including users with at least one portal membership or content uploading experience) |
36 | 9.16 |
Habitual user | 10 | 2.54 |
Cultural heritage portal participation | ||
Member of at least one portal | 15 | 3.82 |
Have customised (or tailored) Web portal to fit your preferences and interests | 13 | 3.31 |
Have uploaded content, which was created or edited by you, to at least one Web portal | 25 | 6.36 |
Data analysis
Data analysis strategy
Confirmatory factor analysis was performed with SPSS AMOS 18.0. The maximum likelihood estimation methods with bootstrapping (on 2000 samples using the maximum likelihood estimator and the 90% bias-corrected confidence level interval) were conducted to assess the stability of parameter estimates and to provide robust standard errors. Model fit was ascertained using multiple fit indices such as the comparative fit index (CFI), Tucker-Lewis index (TLI) and root mean square error of approximation (RMSEA). While the comparative fit index and Tucker-Lewis index measure the relative improvement in the fit of the researcher’s instrument model over that of a baseline model (goodness of fit measure) where a value of one indicates the best fit, the root mean square error of approximation reflects the covariance residuals adjusted for degrees of freedom (lack of fit measure) where a value of zero indicates the best fit (Kline 2010). The root mean square error of approximation has an advantage of known sampling distribution; therefore, confidence limits can be calculated. Comparative fit index, Tucker-Lewis index and root mean square error of approximation are less sensitive to sample sizes and suited for confirmatory large sample analyses (Bentler, 1990; Rigdon, 1996). Although Hu and Bentler’s (1999) approximate fit indices recommended a combination rule that requires an incremental close-fit index cut-off value close to .95 and an root mean square error of approximation value near .06 as a way to minimise Type I and Type II errors, this study utilised these recommended cut-off values as reference points but not definitive decision points because of the following two reasons: first, having a fixed cut-off value for each index generalised across different sample size and varying conditions is cautioned by researchers (Marsh et al. 2004), and second, the validity of the two-index strategy is in question because the group of indices including Tucker-Lewis index, comparative fit index, and root mean square error of approximation are less sensitive to unspecified factor loadings (Fan and Sivo 2005). In testing exact fit between the model and observed covariance, the chi-squared difference test was conducted.
Results
Preliminary analysis
The assumptions of multivariate normality were tested for the sample data by kurtosis and skewness using SPSS 20.0.0. Minimal multivariate non-normal distribution was assessed. The multivariate outliers were also tested using Mahalanobis distance (d2) statistic through SPSS 20.0.0. Given the wide gap in the Mahalanobis d2 values between the first six cases and the remaining cases within the sorted d2 list, the first six cases were considered outliers and they were deleted. The final sample size was 387, and there were no missing data.
The validity of the indicator variables of each adapted model (data quality, service quality, and technology acceptance model) was also tested by confirmatory factor analysis. This test was used to make sure that each construct was psychometrically sound before the constructs were tested for validity of their relationships. The fit indices of the three models were not within the acceptable ranges indicating that the specified models were not supported by the sample data. Table 5 shows the values of models’ fit indices. Post hoc model fittings were pursued to detect parameters that did not fit, and the models were re-specified (as recommended in Schumaker and Lomax 2004). The indicator variables were eliminated by using multiple statistical criteria: below 0.4 standardised regression weight to reduce measurement errors (Ford et al. 1986), or above 2.58 in absolute value of standardised residual covariance to avoid prediction error and high modification indices. Dimensions with high covariates were closely examined for better determinants of the constructs (e.g., the operational dimension showed much in common with the intrinsic dimension, and intrinsic dimension was proved to be a better determinant of data quality; the reliability domain shared characteristics in common with both the responsive and empathy dimensions, and the latter two dimensions were proved to be better determinants of service quality). Conceptually sound improvements were made as suggested by the modification indices, and this resulted in better fitting, acceptable models. These models then were combined to create a measurement model. Table 5 indicates the values of the modified models’ fit indices.
Model | χ2 | df | p | CFI | TLI | RMSEA (90% CI) |
---|---|---|---|---|---|---|
Initial models | ||||||
Data quality model | 1893.921 | 373 | .000 | .811 | .794 | .103 (.098, .107) |
Service quality model | 2604.127 | 460 | .000 | .717 | .695 | .110 (.106, .114) |
Technology acceptance model | 801.606 | 132 | .000 | .883 | .865 | .115 (.107, .122) |
Re-specified models | ||||||
Data quality model | 42.382 | 24 | .012 | .990 | .985 | .045 (.021, .066) |
Service quality model | 37.797 | 24 | .036 | .989 | .983 | .039 (.010, .061) |
Technology acceptance model | 85.602 | 32 | .000 | .980 | .972 | .066 (.049, .083) |
Measurement model analysis
The measurement model, which consists of nine latent variables (responsiveness, empathy, assurance, representational, contextual, intrinsic, perceived enjoyment, perceived ease of use, perceived usefulness) measured by twenty-eight indicator variables, was tested by confirmatory factor analysis. The result indicated that the overall fit indices were within acceptable ranges (Table 6). The AMOS modification indices, however, indicated that adding a path from the service quality model to the perceived enjoyment variable would improve the measurement model fit to the data (modificiation index = 23.239). The modification index also indicated that adding a path from the service quality model to the intrinsic variable would improve the measurement model fit to the data (modification index = 15.827). The fit indices of the measurement model, before and after the model re-specification with these two added paths, are provided in Table 6.
Model | χ2 | df | p | CFI | TLI | RMSEA (90% CI) |
---|---|---|---|---|---|---|
Initial models | 814.809 | 338 | .000 | .934 | .926 | .060 (.055, .066) |
Re-specified models | 769.580 | 336 | .000 | .940 | .932 | .058 (.052, .063) |
The internal consistencies of most dimensions within the final measurement model were at a satisfactory level (above .70) although the internal consistencies of responsiveness and assurance were only moderately so (.673 and .679, respectively) showing lower Cronbach’s alpha values than other dimensions (see Table 7). Table 7 also shows that the inter-correlations among the dimensions were positive and statistically significant, supporting the measurement model’s construct validity. Figure 1 shows the re-specified measurement model with standardised regression coefficients (β), unstandardised regression coefficients (B), and squared multiple correlations (R2). Table 8 lists the mean and standard deviations of measurement model items.
1 | 2 | 3 | 4 | 5 | 6 | 7 | 8 | α | Mean | SD | |
---|---|---|---|---|---|---|---|---|---|---|---|
1. Responsiveness | .673 | 4.58 | 0.93 | ||||||||
2. Empathy | .678** | .721 | 4.67 | 0.94 | |||||||
3. Assurance | .709** | .673** | .679 | 4.31 | 0.94 | ||||||
4. Representational | .644** | .661** | .557** | .823 | 5.09 | 0.87 | |||||
5. Contextual | .611** | .589** | .528** | .803** | .847 | 4.98 | 0.84 | ||||
6. Intrinsic | .614** | .648** | .586** | .737** | .682** | .701 | 4.70 | 0.95 | |||
7. Perceived enjoyment | .586** | .634** | .553** | .582** | .569** | .520** | .859 | 4.54 | 0.96 | ||
8. Perceived ease-of-use | .684** | .697** | .587** | .765** | .741** | .661** | .595** | .893 | 5.23 | 0.84 | |
9. Perceived usefulness | .624** | .655** | .561** | .802** | .812** | .730** | 564** | .791** | .881 | 5.27 | 0.85 |
Note: ** Correlation is significant at the 0.01 level (2-tailed). |
Item Label | Mean | SD | |
---|---|---|---|
Responsiveness 1 | I can upload content at an acceptable speed. | 4.40 | 1.35 |
Responsiveness 2 | The cultural heritage portal's response e-mails are applicable to my original inquiries. | 4.86 | 1.15 |
Responsiveness 3 | The cultural heritage portal provides easy access to multiple Websites. | 4.50 | 1.06 |
Empathy 1 | The design of the cultural heritage portal minimizes distractions. | 4.56 | 1.17 |
Empathy 2 | The cultural heritage portal is continuously updated in response to user feedback. | 4.61 | 1.18 |
Empathy 3 | All hyperlinks within the cultural heritage portal are relevant. | 4.84 | 1.16 |
Assurance 1 | I am able to customize the cultural heritage portal. | 3.61 | 1.28 |
Assurance 2 | The cultural heritage portal clearly states the services it provides. | 4.96 | 1.04 |
Assurance 3 | The cultural heritage portal’s privacy policy is clearly stated on the portal. | 4.35 | 1.30 |
Representational 1 | The cultural heritage portal's data is easy to understand. | 5.06 | 0.98 |
Representational 2 | The quantity of data delivered by the cultural heritage portal is appropriate. | 4.89 | 1.09 |
Representational 3 | The cultural heritage portal gives information about the source of all data. | 5.31 | 0.98 |
Contextual 1 | The cultural heritage portal content is relevant to my needs. | 5.18 | 0.94 |
Contextual 2 | The cultural heritage portal content is detailed. | 4.74 | 0.98 |
Contextual 3 | The cultural heritage portal gives me the data I need within a time period that suits my needs. | 5.01 | 0.97 |
Intrinsic 1 | The cultural heritage portal content is impartial. | 4.83 | 1.22 |
Intrinsic 2 | The cultural heritage portal does not require me to access different systems to find the content I need. | 5.08 | 1.10 |
Intrinsic 3 | The cultural heritage portal does not contain duplicate data. | 4.18 | 1.29 |
Perceived enjoyment 1 | I enjoy using the cultural heritage portal. | 4.60 | 1.08 |
Perceived enjoyment 2 | Using the cultural heritage portal stimulates my curiosity. | 4.72 | 1.05 |
Perceived enjoyment 3 | Using the cultural heritage portal arouses my imagination. | 4.30 | 1.12 |
Perceived ease-of-use 1 | I can quickly find the services I need. | 5.32 | 0.99 |
Perceived ease-of-use 2 | The cultural heritage portal's navigation system is intuitive. | 5.23 | 0.95 |
Perceived ease-of-use 3 | The cultural heritage portal is user-friendly. | 5.35 | 0.94 |
Perceived ease-of-use 4 | The cultural heritage portal enables me to accomplish tasks more quickly. | 5.03 | 1.00 |
Perceived usefulness 1 | The cultural heritage portal gives me access to accurate content. | 5.39 | 0.92 |
Perceived usefulness 2 | The cultural heritage portal gives me access to the most current content. | 5.07 | 1.00 |
Perceived usefulness 3 | The cultural heritage portal gives me access to high quality content. | 5.37 | 0.92 |
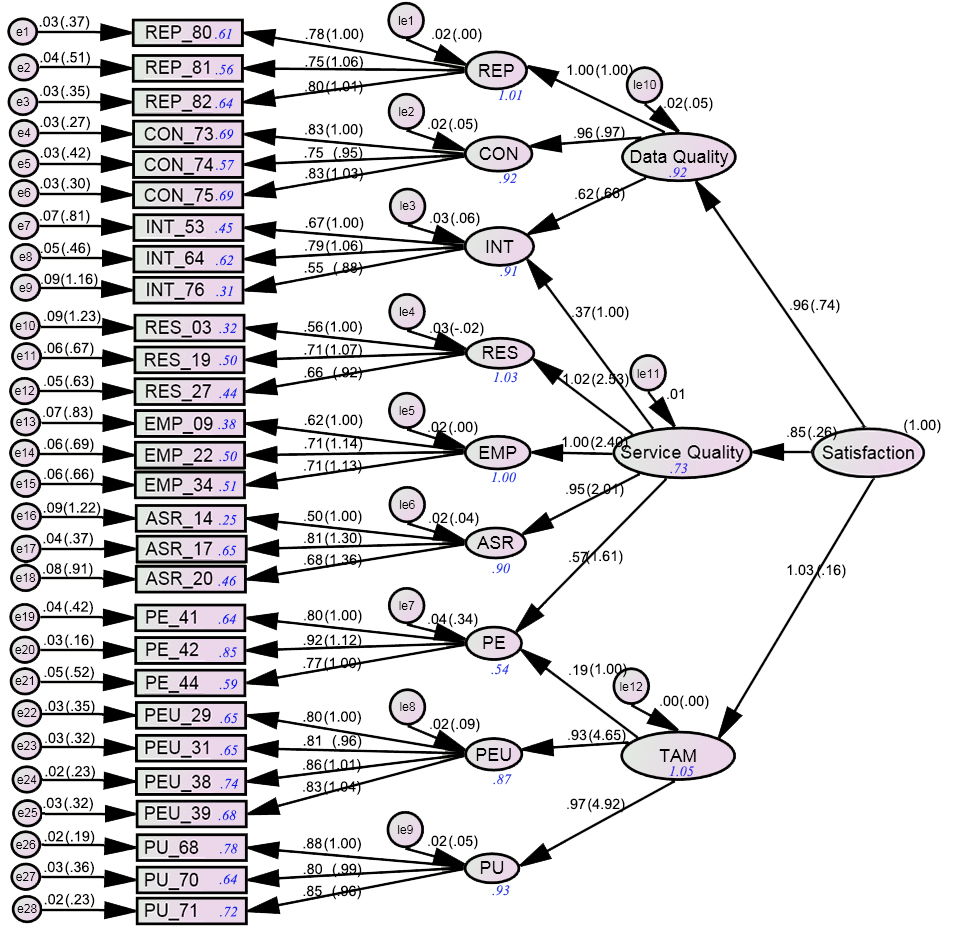
Discussion and implications
This study examined the potential of an instrument model, which was adapted from three existing theoretical frameworks of data quality, service quality and technology acceptance model, for measuring user needs of cultural heritage portals. Researchers of the data quality model debated whether dimensions and measures of data quality were contextual (Batini et al. 2009; Cappiello et al. 2004). In accordance with the contextual perspective, the adapted data quality model was not supported by the sample data; an adjustment of the data quality model specific for the context of cultural heritage portals was needed. Within the measurement model, it was also found that the intrinsic dimension had a high covariance with the adapted service quality model, implying that the intrinsic dimension was not a distinct dimension unique to data quality. Representational and contextual dimensions, however, were proven to be distinct dimensions of the data quality model.
Researchers of the service quality model have cautioned that the number of service quality dimensions is contextual (Carman, 1990; Gounaris & Dimitriadis 2003; Ladhari 2009; Li et al. 2002) and recommend ensuring the stability of dimensions by adapting the model. When adapted for the current study, the adapted service quality model was not supported by the sample data. In testing the measurement model, however, it was discovered that with the sample data, the responsive, empathy and assurance dimensions were distinct dimensions of the adapted service quality model.
While the technology acceptance model has been highly studied in the field of information technology (see Chuttur 2009 for an overview), the preliminary study found the adapted technology acceptance model to be unsupported by the sample data. Further, although many researchers recommend perceived enjoyment as a determinant of behavioural intention and usage of a technology, it was found that the perceived enjoyment dimension had a significant covariance with the adapted service quality model when the adapted technology acceptance model was merged into the measurement model, indicating that the perceived enjoyment dimension was not unique to the adapted technology acceptance model. Perceived ease-of-use and perceived usefulness dimensions, however, proved to be distinct dimensions of the adapted technology acceptance model.
This study provides a theoretical contribution to the field of cultural heritage portal research. Since the cultural heritage portal is a comprehensive information system with large volumes of data and variety of services and is still relatively new to its users, it was reasoned that measuring its user needs using one framework alone might not be sufficient. The study tested three widely used theoretical frameworks (data quality and service quality models for measure of quality, and technology acceptance model for measure of user acceptance) within the cultural heritage portals domain. Through the measurement model, this study examined the potential of integrated dimensions formed from these frameworks to measure cultural heritage portal users’ needs. During this process, this study demonstrated that the three frameworks measure both unique dimensions of user needs and also share some dimensions. This finding provides portal researchers with a word of caution: using one framework alone is not sufficient for assessing the user needs of the comprehensive, cultural heritage portals.
While this study does make an important contribution to the field, several limitations need to be considered. First, the study went through multiple model re-specification processes to achieve a better model fit. The results of the study therefore need to be considered as exploratory rather than confirmatory. Given the exploratory nature of the model re-specification, the recommendation is to replicate the proposed measurement model with independent samples to assess the stability of model fit. Until then, the generalisability and plausibility of the measurement model will not be approved. Second, although the fit indices of the re-specified measurement model were within acceptable range, several standardised coefficients were at or slightly larger than one, suggesting that the there might have been multicolinearity in the sample data. While the study provides some guidance to cultural heritage portal researchers regarding user needs, caution should be practiced to conclude on the predictive power of the underlying factors of user needs regarding cultural heritage portals. Third, the data quality scale adapted for the current study does not reflect the ISO/IEC 25012 standard. A scale that reflects the standard (e.g., Moraga et al. 2009) may need to be considered in future studies.
Acknowledgements
This work was supported by the National Research Foundation of Korea and is grant funded by the Korean Government (NRF-2010-330-H00006).
About the author
Misook Heo is an Associate Professor in the School of Education, Duquesne University, USA. She received her Master of Science in Information Science and Ph.D. in Information Science from University of Pittsburgh, USA. She can be contacted at: heom@duq.edu