Conceptualizing outcome and impact measures for intelligence services
Rhiannon Gainor and France Bouthillier
School of Information Studies, McGill University, Montreal, Canada
Introduction
Literature of various intelligence fields, such as competitive intelligence and business intelligence, contains criticisms regarding measurement practices and activities to determine the value of intelligence. These criticisms include concerns about the lack of conceptual consistency, the multiplicity of measurement approaches and lack of consensus regarding best practices, and the lack of research evidence to support measurement activities, both conceptually and methodologically (e.g., Hughes, 2005; Lönnqvist and Pirttimäki, 2006; Marin and Poulter, 2004; Wright and Calof, 2006).
As part of a larger research project, the authors examined the literature of intelligence measurement in a variety of intelligence fields, specifically measurement to determine organizational outcomes and impact of intelligence. The authors found, however, that the literature contained some conceptual ambiguities specifically related to outcomes, impact, and measurement. Note that for the purposes of this article, which deals with measurement of intangibles, the following definition is provided: Measurement is the activity of identifying and then quantifying phenomena on an accepted scale of quantity or value.
Just as authors such as Savolainen (2009) and Bates (2005) have recognized the existence of ambiguous concepts in library and information studies and worked to clarify those concepts for the field, the authors determined that there was a need to go beyond what is explicit and more critically examine the underlying theory and philosophy of measurement being propounded in the intelligence measurement literature. Believing that consensus in terminology, conceptualization, and measurement practice is necessary in order to improve measurement and formulate best practices, the authors developed this exploratory and qualitative study. The study design consists of interviewing a small sample of intelligence experts working, publishing and presenting on intelligence measurement in various countries. Participants were asked: to define some key terms for outcome measurement; how they conceptualize intelligence measurement; if those conceptualizations address the challenges of outcomes and impacts in measurement, and if so, how; and the characteristics of an effective intelligence outcome measurement model. The interview guide can be found in Appendix A.
The purposes of this study were: to obtain information about expert opinions and conceptualizations in order to clarify ambiguous concepts in intelligence specifically related to intelligence outcomes, impact, and measurement; and to initiate comparative discussion about intelligence measurement, which has been to date elusive in the literature.
This article reviews intelligence measurement literature to identify conceptual challenges and the literature which informed the study design. A summary of the research design is then provided, along with the study findings. The discussion compares study participants' conceptual frameworks, providing an opportunity to make more explicit to researchers the challenges and potential future directions in conceptualizing measurement of intelligence outcomes to prove value or effectiveness, an important step toward consensus and the formulation of best practices.
The conceptual problems of measurement for intelligence
Competitive intelligence, which can be defined as the intense production and analysis of information about the competitive environment resulting in intelligence products for use in organizational decision-making, is one of several intelligence fields. Competitive intelligence, business intelligence, market intelligence, covert intelligence, counter-intelligence, and military intelligence share common roots and many practices (Juhari and Stephens 2006). For all of them, the intelligence cycle involves the following steps: information needs are identified; data and information are sourced; data are analysed and become intelligence; and resulting intelligence products, such as reports and industry profiles, are distributed. Intelligence products are used to inform decision-making and thereby improve decision outcomes, which is the ultimate purpose of intelligence (Bouthillier and Shearer, 2003; Leslau, 2010). Intelligence is therefore a process, the intelligence cycle, and a product, the intelligence deliverables.
Lönnqvist and Pirttimäki (2006) state that from their survey of business and competitive intelligence literature two primary reasons for measurement are given by authors: to improve process and to prove value. From a practical perspective, the value of measuring competitive intelligence is that it fosters improvement by providing data on performance (e.g., Blenkhorn and Fleisher, 2007). Authors have argued that measurement gives reassurance to high level stakeholders that there will be a return on investment, while staff, knowing that measurement activities are taking place, have an incentive to improve processes and deliverables (e.g., Buchda, 2007). Surveys have indicated that while there is little use of competitive intelligence performance measures in organizations (e.g., Marin and Poulter, 2004; Prescott and Bharwaj, 1995), competitive intelligence practitioners are aware of the need of measurement to advance practices in their field (e.g., Qingjiu and Prescott, 2000).
There is a documented history of problematic measurement in all intelligence fields, and this may be due to a variety of factors. Competitive intelligence practices are a recent development, formalized with the publication of Michael Porter's book Competitive Strategy in 1980. The youth of the field, connected to not only limited measurement practices but also limited awareness of measurement needs, may partially account for its measurement challenges. For example, Herring's (1996) small survey of senior executives overseeing a competitive intelligence programme indicated that while none of the executives in his sample were evaluating their programme activities, they were open to doing so once the idea was introduced to them.
In the field of national security intelligence (encompassing covert, military, and counterintelligence), Dahl (2010) argues that performance measurement has historically been a simple success or failure binary that does not provide critical information for improving performance. Reasons given in the national security intelligence literature for this narrow view of measurement tend to revolve around methodological challenges related to the politicization of intelligence in national security settings (Hulnick, 2006) and problems with conceptualization of measurement (Hastedt, 1991; Turner, 1991).
Another complicating factor for all intelligence fields may be that measurement itself is inadequately understood and practiced. Authors in a variety of fields related to business and the social sciences have argued that measurement practice does not follow measurement theory strictures and tenets, resulting in data and instrumentation errors (Bontis, 2001; Flamholtz, 1980; Gorad, 2010; Pike and Roos. 2004).
Issues of practice, history, and politicization aside, significant conceptual challenges which are retarding development of effective and standardized measurement approaches and tools are identified within the literature. These conceptual challenges to outcome measurement can be summarized as follows:
Multiplicity of measurement models, approaches
There are many measurement models and recommendations in the literature (e.g., Davison, 2000; Herring, 1996; Moore, Krizan and Moore, 2005; Steventon, Jackson, Hepworth, Curtis and Everitt, 2012) but comparative discussion regarding the models and recommendations is elusive. Recommendations tend to be prescriptive rather than tested. As Blenkhorn and Fleischer (2007) have pointed out, much of the literature consists of anecdotal reports of practitioner experience, which does not provide the evidence necessary to formulate best practice. Of course, many authors have also argued that the unique situation of each industry and organization renders the standardization of measurement tools impossible (e.g., Kilmetz and Bridge, 1999; Rothberg and Erickson, 2005).
Inconsistent use of terminology
Notable among challenges for intelligence measurement is the significant conceptual inconsistency across authors and publications in describing measurement and value, as found, for example, by Wright and Calof (2006) in their small study examining published competitive intelligence research in three countries. Inconsistencies between organizations and agencies in terminology use also exist; the discrepancies in use of the terms business intelligence and competitive intelligence in various parts of the world are an example (Buchda, 2007).
Unproven outcomes
Lönnqvist and Pirttimäki (2006, p. 33) complain of output value conceptualizations, stating that the literature 'includes a lot of unverified assumptions' about the possible benefits of intelligence to organizations. A comparison of the many surveys which ask competitive intelligence practitioners and managers what benefits should result from competitive intelligence contains a great deal of overlap, but also significant discrepancies (see Hannula and Pirttimäki, 2003; Jaworski and Wee, 1992; Marin and Poulter, 2004; Qingjiu and Prescott, 2000). Such speculative responses are at least partially due to a lack of research providing causal evidence as to what outcomes, beneficial or otherwise, are related to intelligence practices.
Good enough measurement
In his textbook on security and military intelligence analysis, Clark (2010) calls for 'the right things' to be measured, pointing out that intelligence organizations in the US and Russia do not have established feedback loops to provide assessment of how valuable intelligence activities have been. Instead, the measurement focus tends to be on process. Yet the purpose of intelligence is not process. For all intelligence fields, the primary purpose of intelligence is to inform decision-making, with the intent to increase the likelihood of the most optimal outcomes for the organization (Bose, 2008; Leslau, 2007; Turner, 1991). This disconnect, rooted in the significant conceptual and methodological challenges of not only measuring intelligence outcomes but intangibles measurement itself, has led to the popularity of good enough measures that do not give a complete picture of value to the organization or performance of the intelligence unit. Measures in use may be the accuracy and timeliness of the intelligence deliverables, and numbers of reports delivered over time.
Such process measures, which treat intelligence as an output of a system rather than an input into decision-making, in combination with user satisfaction surveys, appear to be the foundation of good enough metrics in both national security and business-related intelligence fields (Ganesh, Miree and Prescott, 2004; Moore et al. 2005). Unfortunately such process measures do not address problematic questions of validity in performance or value measurement. Determinations of value or benefit are necessary components in conceptualizations of performance.
Performance can be defined as a set of criteria critically applied to purposeful activity within organizations. Carton and Hofer (2006: 3) in their handbook on measuring organizational performance called it a "contextual concept" and a "multi-dimensional construct" that "involves measurement of the effects of organizational actions". Performance varies by industry and sometimes organization, determining what results in relation to which situational values are good or bad. Situational value judgments aside, the definition provided points to the essential criterion for determining performance, an identification and quantification of effect, or outcome and impact, which process measures alone cannot provide.
Calls for improvement
Scholars who have recognized the conceptual and methodological problems inherent in current measurement practices have made calls for research. Those interested in determining the value of competitive intelligence have made calls for research so that measures of competitive intelligence outcomes might be developed and that the benefit and value of it to organizations might be determined (Hughes, 2005; Marin and Poulter, 2004; Wright and Calof, 2006). In the national security field, calls have also been made for better conceptualization and, in turn, communication of what intelligence is and what it can be expected to accomplish (Lowenthal, 2013; Turner, 1991).
In the light of the problems and discussions identified above, and recognizing that, as argued by Liebowitz and Suen (2000), conceptual disagreements and problems lead to measurement confusion and error, the authors developed a study to try and address some of the conceptual questions about intelligence measurement.
In reading the literature of intelligence measurement, it became apparent to the authors that some of the most interesting discussion about measurement was being made implicitly, in statements that only half-revealed discussions about measurement and intelligence and hinted at the authors' own conceptual lenses. These half-visible discussions raised significant questions. For example: why do some authors, many of whom are practitioners, make the statement that standardization of measurement tools is impossible for intelligence work? Tools themselves, whether they be Likert scales or sliding rulers, once accepted and understood as a scale and indicator of size or value, can be used regardless of context. Do these authors actually mean that a suite of tools with customizable interchanging parts, dependent on organizational context, was necessary? Do these statements reflect a belief that measurement would be essentially process-related and not outcome-based? Were these statements presupposing unique tangible outcomes and ignoring more generic intangibles under headings such as innovation, relationships, or policy?
Authors in the intelligence measurement literature may provide only partial explanations as to the rationale behind their recommended measurement models and approaches, and there is little discussion as to how one author's recommendations align with, complement, or refute those of another author. Such elliptical discussion in the literature is likely a contributing factor to the conceptual difficulties of intelligence measurement. There is also little discussion of measurement theory and best practices in measurement when making recommendations, and of what good measurement looks like.
Recognizing the common roots and practices (namely, the intelligence cycle) of all intelligence fields, the authors decided to interview subject experts, people who were presenting and publishing in intelligence measurement in various intelligence fields, in order to examine in closer detail conceptualizations of intelligence measurement that are informing research and practice and explore whether the elliptical references in the literature (referenced above) would merit further study. Sampling criteria were that at least three different intelligence fields were represented by participants; academics and practitioners would be included; and at least two countries would be represented in the sample. This sampling criteria was developed in order to mirror the broad range of intelligence measurement literature which prompted the research questions, and to provide opportunity for a range of divergent opinions, in the belief that where or if those opinions converged, it might provide insight into potential areas of consensus.
Because this study was developed to meet a need within a larger, doctoral study concerned with measuring organizational outcomes and impacts of intelligence, the research questions were formulated as follows:
- How do these experts conceptualize the outcomes and impact of intelligence?
- How do experts in intelligence conceptualize measurement for intelligence outcomes and organizational impacts?
- How do the measurement models used and/or developed by these experts compare to one another?
The purpose of this small exploratory study was to clarify discourse in the literature around intelligence measurement, specifically outcome-based intelligence measurement.
Research methods
The authors conducted a field study in 2012, interviewing a sample of five intelligence experts working in Finland, the United Kingdom, and the United States. Experts were defined for the purposes of this study as scholars and practitioners in any field of intelligence (competitive, market, military, covert, etc.) who have publicly presented or published on intelligence measurement. Participants came from the fields of business intelligence, competitive intelligence, and covert/counter intelligence. They included university faculty, former US Central Intelligence Agency employees, and a competitive intelligence practitioner working with the UK Competitive Intelligence Forum to develop performance measures for competitive intelligence.
Potential participants were identified through publications and conferences, and snowball sampling was used in an attempt to increase the number of participants. Interviews were face to face, semi-structured interviews, which took on average just under an hour. Prior to the interviews, participants were sent a copy of the interview questions along with informed consent forms. All participants were notified that they could refuse to be recorded, participate anonymously, refuse to answer a specific question, or withdraw at any time, including after the interview was concluded. All participants completed the interview and agreed to have their names published in this study, as authors and presenters of research and/or practice in the field of intelligence and measurement. The five participants were:
Professor Antti Lönnqvist, Department of Information Management and Logistics, Tampere University of Technology, Finland
Dr. Sheila Wright, Leicester Business School, De Montfort University, UK
Mr. Andrew Beurschgens, Head, Market and Competitive Intelligence at a large UK mobile telecommunications firm; Board Member for the UK Competitive Intelligence Forum (UKCIF)
Dr. Stephen Marrin, Centre for Intelligence and Security Studies, Department of Politics and History, Brunel University, UK
Dr. John Kringen, Researcher at the Institute of Defense Analyses; formerly of the US European Command and the US Central Intelligence Agency
Participants were asked to comment on their measurement conceptualizations and models in relation to intelligence and outcomes rather than processes. They provided their own definitions of the terms output, outcome, and impact; descriptions of their measurement tools and measurement methods; and critiques of current intelligence measurement research and practice. They also provided some description of the history of their conceptualizations and their measurement models, and some informed critique of each model's purposes, strengths, and weaknesses.
After the interviews, the researcher reviewed the interview notes and recordings to capture notable quotations and to partially transcribe responses of participants. Reponses were summarized and compressed to distil essential elements for comparison. These summarized notes were then sent in electronic, written form to study participants, who were given the opportunity to review and edit the researcher's notes and conclusions to ensure that the notes accurately reflected their thoughts, practices, and comments on intelligence measurement. This review served the additional purpose of preventing publication of any inaccurate statements participants might then wish to retract.
Once all participant edits were received, the data were analysed. Responses were compared and contrasted to one another in several categories, as shown in the next section, which reviews the findings.
Findings
The findings of the study are here grouped under three subheadings: definitions, descriptions of participants' current outcome measurement practices, and description of ideal outcome measurement.
Definitions
Participants were asked to provide definitions for three terms: output, outcome, and impact. Some definitions were closely aligned, while others varied widely.
All participants agreed that outputs are usually tangible and easily identified. Outputs may take the form of products such as reports, increased situational awareness or knowledge on the part of the intelligence recipient, subsequent actions, or events. Outputs were described as typically occurring soon after the intelligence has been delivered to an audience.
While all participants agreed that outcomes were distinct from outputs and typically intangible in nature, some significant discrepancies occurred in the definitions provided. Outcomes were described by four of the five participants as an effect or intangible change within the decision-maker, the decision itself, or the audience who received the intelligence. Such outcomes might be a decision-maker's perspective, the effect on the decision itself, or the changed information need or information reception due to intelligence provided after a decision is made.
Antti Lönnqvist and John Kringen described outcomes at an organizational level and as building on, and being related to, outputs. Sheila Wright and Stephen Marrin stated that outcomes and outputs are unrelated and that the provision of intelligence may result in outputs, outcomes, both outputs and outcomes, or neither. Andrew Beurschgens defined outcomes as the intangible effects upon the audience, asking, Is the audience stimulated, provoked, motivated? For him, this is the outcome of intelligence, and it is directly related to the salesmanship of the competitive intelligence practitioner; in other words, his/her ability to get an audience engaged in using intelligence.
The greatest discrepancies in definitions occurred around the concept of impact. All agreed that intelligence impact is not, and should not be defined as, related to organizational strategy, although Antti Lönnqvist and John Kringen stated that the decision-maker(s) may link intelligence to strategy. Andrew Beurschgen did offer a caveat that if a competitive intelligence programme is aligned with corporate strategy then it is expected to influence the outcomes of strategic reviews.
Impact was variously defined as reduction of risk in the decision-making process (Sheila Wright), its effect upon policy (Stephen Marrin and John Kringen), its effect upon the decision-maker in the context of a decision (John Kringen), an indication of success that is closely related to, perhaps synonymous with, outcomes (Antti Lönnqvist), and simply, the magnitude of a given outcome's influence (Andrew Beurschgens).
Current measurement practice
Participants were asked to describe how they currently measure intelligence outcomes and impact. Only three of the five participants reported that they have attempted to measure outcomes and impact.
Antti Lönnqvist described his outcome measurement approach as a generic model often found in business literature and used by other scholars. This generic model consists of direct, indirect, subjective, and objective measurement, and the need to take pre-measurement steps. The pre-measurement steps are questions which ask why there is a need for measurement, what is being measured, and identify success factors and standards in relation to the input(s) and the viewpoint(s) of the audience. While he sees conceptual and practical limitations in this model related to identifying success factors that address contextual variation, he sees its strength as its customizability and thus how customization forces users to consider the purpose of the measurement activity, thus increasing its validity.
Antti Lönnqvist, in common with other participants, indicated that in his measurement approach, process measures are necessary to inform and make possible outcome measures:
Anyone trying to measure the outcomes of intelligence needs to understand the intelligence process and what is on the manager's mind when requesting, using, and discussing intelligence. Then we can identify what new information is brought by the intelligence. For example, before we can measure impact we have to ask, is the information being accessed and then being used? So a process measure such as usage statistics is needed to be a part of outcome measurement.
Andrew Beurschgens and John Kringen described customer feedback as the chief mechanism through which outcomes and impact might be assessed, if not measured. John Kringen stated that general practice at the CIA is to use a combination of process and satisfaction measures in combination with debriefing sessions to obtain insight into how well their service is valued by their users. Although he acknowledges it to be imperfect, he also considers this practice to be useful and workable. As a part of these measures the CIA attempts to identify indicators (signposts) of success. However, such feedback is not quantified by any kind of formal metric, and is not always available, due to problematic access to users, particularly high-level decision-makers. Similarly, Andrew Beurschgens, describing practices in competitive intelligence, states that he uses a high level structured feedback approach, based on the work of Tim Powell (a competitive intelligence practitioner and author of how-to texts such as Analyzing Your Competition: Its Management, Products, Industry and Markets, published by Find/Svp Info Clearing House) and research being done with the UK Competitive Intelligence Forum. Such user feedback, in his view, should relate to questions about the timeliness and usefulness of the intelligence, whether the stakeholders were better-informed about relevant issues, and whether the decision-makers were better enabled to reach a consensus. He also described how technology tools, through tracking processes such as usage rates and new project development, might also identify outcomes and impacts such as the dollar value of business opportunities lost or gained.
Stephen Marrin and Shelia Wright do not have an outcome measurement tool or method that they use, although Stephen Marrin (2012) has conceptualized directions intelligence measurement could take, such as batting averages. Sheila Wright stated in her interview that if she were asked to measure the outcomes or impact of competitive intelligence, she would attempt to convince the requestor not to try the nearly impossible. She then continued with this statement, questioning the value of such measurement:
Rather than ask, what is the value of having CI, it is more useful to ask, what is the value of not having CI? Another question is, why do we need to prove the value of CI units? There are many business departments, like strategic planning, which are considered just "a cost of business", which are not required to prove their value.
All participants agreed that intelligence measurement as it is currently practiced, including their own measurement practice, is problematic and could be improved. Responses given as to why these problems exist were voluminous and diverse. Participants were unified in citing problems with managing feedback mechanisms, namely gaining access to intelligence users, and the subjectivity of user statements. Participants also cited problems with establishing causal relationships between action and effect in intangibles, and isolating effects for measurement.
Significant conceptual problems for current measurement practices were also discussed. One was the lack of consistency in approach to measurement, which is directly related to non-standardized measurement tools. Another is attitudinal: under-valuation of both intelligence and measurement resulting in non-cooperation between departments, and managerial resistance or disinclination to participate, in organizations.
Stephen Marrin argued that a conceptual framework is needed for security intelligence measurement, stating fundamental concepts that would inform intelligence measurement are not yet developed. As an example, at one point in his interview, he cited the fact that while in business financial measures can be used as a fundamental quantifier of value, there is no single currency of value in intelligence.
John Kringen pointed out, specifically in relation to security intelligence, that the weakness of current intelligence measurement is that there is no conceptual model of intelligence system dynamics that looks at both inputs and outputs, when outputs are policy outcomes either domestically or in recipient societies. Such conceptual models, he argues, are necessary in order to determine feasible outcome measurement. He discussed the need for ways to quantify intelligence challenges in the context of the intelligence problem. He noted that rating intelligence by its accuracy is not an adequate reflection of performance. Very simple research tasks might result in perfect accuracy, while highly complex and challenging research tasks involving multiple stakeholders might result in less accurate, but potentially far more valuable, intelligence products. He suggested that rating intelligence performance should be more like assigning scores to Olympic diving than generating batting averages in baseball, but acknowledges the conceptual challenge to such a shift in perspective and measurement tools is that right now there is no agreement on the judging scales to be used.
These conceptual criticisms were echoed by Andrew Beurschgens, who stated that not only his own measurement approach, but all current competitive intelligence measurement approaches, lack consistency and rigour. He attributes these problems to inadequate research into and literature on conceptual models upon which measurement might be based, commenting that 'there is not the same level of literature available on measurement models as there is now on the analysis part of the CI process'.
Future measurement
All participants acknowledged that their measurement practices and conceptualizations were imperfect, and stated that they considered their measurement approach to be dynamically changing as they encountered new research and ideas for practice. Participants were asked to comment on what outcome measurement should be in the future, specifically naming desirable characteristics of an ideal robust and useful outcome measure for intelligence. Four of the five participants supplied homogenous lists of necessary characteristics.
According to those responses, any robust and useful measure of intelligence outcomes would be:
- Reliable: not only meaning that the measurement tool be consistent, but that more than one measurement tool is used in a composite or multi-measurement method approach
- Valid: the audience and purpose of the measurement activity are addressed and made explicit by the choice of measurement tool(s) and approach(es)
- Causal: the measure relates intelligence to beneficial effects (traces causal relationships)
- Credible: results obtained are supported by evidence of value, either quantitative or qualitative, positive or negative. The data captured is non-politicized and objectively fair
- Usable: the measure is not only easy and simple to use but also to understand, fostering communication between measurer and audience
Other noteworthy but disagreed-upon characteristics and elements were suggested. Sheila Wright, who defines competitive intelligence as behavioural change, stated that an outcome measure should provide evidence of behavioural change. Andrew Beurschgens, who has worked with Sheila Wright in UK Competitive Intelligence Forum, included self-help as an element for his proposed outcome measures, defining it as those who will seek out intelligence in response to an information need. His concept of self-help as an outcome could potentially be an outcome measure for competitive intelligence, when identified as an acquired behavioural response. He also believes, however, that measures can and should provide evidence of value to the organization as a whole. Along with Antti Lönnqvist he suggested that a suite of measurement tools should also include a financial measure.
Antti Lönnqvists business management perspective and Sheila Wright's behavioural change perspective meant that their answers to the interview questions provided both intriguing contrasts and points of correlation. One such correlation occurred around the issue of precision and accuracy in measurement. Sheila Wright argued that it is impossible to measure intelligence value entirely and exactly. Instead, a measurement approach would have to accept that only elements can be captured, and these elements would provide a partial but adequate picture of value. In a discussion about the cost-effectiveness of measurement, Antti Lönnqvist pointed out that academic research looks for accuracy and can make extreme investments in highly complex measurement tools to ensure accuracy and to advance research. Business, in contrast, is often willing to compromise on the accuracy of measurement in order to keep costs down and to simplify measurement activities. He also stated that while accuracy is not possible with inaccurate phenomena such as intelligence outcomes, inaccurate measures can still be helpful, and so he does not see the inaccuracy of outcomes measurement as an obstacle.
In other comments, Stephen Marrin discussed the need for outcome measures which might relate to the role intelligence plays in decision-making and need for proxy measures that could indirectly capture intangibles. Andrew Beurschgens suggested that a measurement model would need to allow for anecdotal evidence and account for the quick depreciation of deliverables, since a competitive intelligence product is often a single-use item. Several participants also discussed the need for outcome measures to show organizational (inter-departmental) usage and reflect varying stakeholder perspectives.
These findings are discussed in the next section.
Discussion
The literature review identified certain criticisms and weaknesses of intelligence measurement that are supported by the findings of this study. Discussion of the findings is put into subsets, below, to facilitate comparison to the literature review.
A multiplicity of models
Some participants complained of the lack of consistency and research in intelligence measurement literature. Yet each described his or her own unique approach to or model of outcome measurement. This reflects what the authors have found in the literature, which is that while some approaches had elements in common, none were identical. Interestingly, while current practice was disparate, participants exhibited strong similarities in their conceptualizations of ideal measurement, discussed below.
Inconsistent use of terminology
When asked to define a sample of terms related to measurement, participants gave varied answers. Even when there was consistency amongst participants, a broader reading of the literature could give examples of other researchers who disagree with the definitions provided in this study.
For example, participants agreed that impact should not relate to organizational strategy and that intelligence is not related to strategy. Yet within the field of competitive intelligence and business intelligence, researchers have identified organizational strategy (Herring, 1996), strategy formulation (Hughes, 2005), and strategic decision-making (Bose, 2008) as being strongly related to competitive intelligence and discovering its value in the application of competitive intelligence deliverables.
The variations in terminology use found amongst study participants reflect larger inconsistencies within intelligence fields.
Unproven outcomes
Participants did not state a need for research to give evidence of intelligence outcomes. However, discussions of ideal outcome measures in the future described measurement tools that are able to make connections between the role of intelligence and improved outcomes, providing evidence of causality.
Good enough measurement
The literature review described a good enough existing measurement practice in intelligence; namely process measures combined with satisfaction measures. John Kringen stated that this measurement practice, although imperfect, is workable and sufficient to meet the most pressing needs in intelligence work at this time. However, all participants indicated that current measurement practices could be improved, and that they would welcome such improvements.
Sheila Wright and Anitti Lönnqvist indicated that partial and inaccurate measurement can suffice, if it provides a simple and inexpensive method to obtain adequate understanding of the phenomenon measured. This concept of acceptable inaccuracy may be worth exploring. Gorad (2010) states in his article on measurement in the social sciences that an inaccurate measurement tool is acceptable for use if the margin of error is known to the user and the audience. Determining an acceptable margin of error would be useful in formulating intangibles measurement tools, and has not yet been explored in the intelligence literature.
Calls for improvement
The literature review cited studies calling for improvements to existing measurement practice. Interestingly, although the study participants acknowledged methodological challenges to measurement, such as access to decision-makers and the lapse of time necessary for outcomes to manifest, their strongest criticisms of current practice were reserved for conceptual problems. For example, the issue of no single currency in intelligence noted by Stephen Marrin was one of many made by participants which highlighted their concerns that inadequate or nonexistent conceptual models are significant challenges for developing effective intelligence measurement.
Such discussion has been previously initiated in intelligence measurement literature, but to date it has been largely overshadowed by discussion of methodological challenges of measurement, in tandem with prescriptive conceptual models and frameworks. The emphasis placed by the participants on conceptual challenges as being the significant source of problematic measurement practice suggests that the time has come for the field to engage in the comparative conceptual discussions and formulation of consensus suggested by this paper.
Pointers toward best practices
Discussions in the literature of best practices in intelligence measurement are elusive. Yet when asked, the study participants were able for the most part to very clearly describe characteristics of ideal outcome measurement, even if they could not describe what the measurement tools themselves would look like. The checklist provided in the study findings will hopefully provide some fodder for a larger discussion about what intelligence measurement best practice should be, as the discrepancies in descriptions of best practices provoke a slew of additional questions not yet raised in the literature. For example, does valid outcome measurement require process measure components, as argued by Antti Lönnqvist? The checklist may also provide a useful starting point for subsequent research on best measurement practices.
A variety of conceptual lenses
In trying to understand the roots of so many different measurement models and approaches in the literature through discussions with study participants, it became clear that even those within the same field of intelligence did not agree on what intelligence is about, or what might be termed its about-ness.
Below is a figure that attempts to visually represent the interpretive lens through which each participant appeared to speak. Participants might well disagree with this, but it is offered here not to pigeonhole them, but rather to show some of the varying stances possible and represented by the participants in the answers they provided specifically for this study.
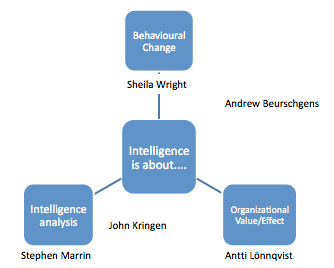
As stated in the introduction, intelligence is sometimes defined as a piece of information (the intelligence product) destined to inform a decision-making process. Intelligence is also defined as a function or process within organizations. Participants appeared to represent differing viewpoints as to the role of intelligence as an organizational service or function to be valuated, and this can be seen in Figure 1.
Sheila Wright stated that intelligence is about behavioural change: upon receipt of the intelligence, how is the audience affected? For example, how was the decision-maker influenced? Did his/her attitude toward a situation change? Stephen Marrin spoke of how intelligence is analysis: the analyst, how well the analyst performs, and the quality of the intelligence produced. Antti Lönnqvist took a management-level perspective, where the role of intelligence is a business function, intended to advance the goals of the larger organization. The other two participants occupy ground between two perspectives, with John Kringen leaning toward intelligence analysis, and Andrew Beurschgens leaning toward behavioural change (Figure 1).
Such differences in perspective may be a significant factor in determining why there is a lack of standardized measurement practices in intelligence, and partially explain why there continues to be prescriptive measurement methods and models presented in the literature. Further research is needed to determine if more conceptual stances regarding the role of the intelligence function in organizations exist than those represented here. Such varied stances, unreconciled, will mean that measures will need to account for audience perspectives on the role of intelligence within the organization, and for its related considerations as to what outcomes are valued.
Marrin (2012) has stated that there is no consensus about the purpose of intelligence. The authors of this study would expand on this and state that while the commonly accepted intelligence cycle suggests (and many studies have supported this) that the purpose of intelligence is to inform a decision, there is no consensus as to how the decision outcomes should be improved; i.e., to what end a decision is informed, which materially affects the would-be measurer's conceptualization of outcomes, impact, and their indicators.
The tensions between desired measurement approach and conceptualizations of intelligence about-ness, are significant and need to be addressed. Antti Lönnqvist discussed in his interview how outcome measurement, once developed, will need to provide for different audiences. He gave an example of business managers who want financial quantities assigned to represent value and who consider subjective measures highly suspect. This presents a problem because qualitative measures are often subjective but are needed to quantify intangibles and cognitive effects. Turner (1991, p. 276) in describing the challenges for national security intelligence measurement, stated that 'responsiveness to the consumer is of utmost importance in evaluating intelligence as a governmental function', adding that '[E]valuating...intelligence is a complex task that must take into account organizational goals, bureaucratic imperatives, availability of resources, and the uses to which policymakers put the intelligence product' (1991, p. 283). Such complexities are insurmountable if there cannot be basic conceptual agreement as to what outcomes of intelligence are expected, as a result of informing decision-making activities.
Conclusion
The study found that that while participants' definitions of outputs and outcomes aligned, definitions of impact were unique to each participant. Participants' descriptions of their own practices in, and reactions to, measuring outcomes and impacts were varied and paralleled discussions in the literature. These included philosophical questions about the need for measurement, necessary questions to determine the reliability and validity of the measurement, descriptions of methodological challenges related to identifying and quantifying intangibles, use of qualitative and quantitative tools, and process and satisfaction measures as a surrogate for outcome and impact measures.
Overall, participant responses paralleled comments and discussions that can be found in the intelligence measurement literature, and provided some additional insights into those discussions. Conceptualizations of intelligence outcomes and impact varied with the perspective of the participants as to the function of intelligence in the decision-making process.
Participants agreed that significant conceptual and methodological challenges exist for developing intelligence outcome and impact measures, and argued for research into addressing the conceptual challenges as a priority for the development of effective measurement. Although they were unsure what future measurement tools might be, and some questioned whether such measurement is possible, most gave detailed and sophisticated lists of criteria which would need to be met by a successful measure. Such a list is unique in the literature and in conjunction with the conceptual differences on the part of the respondents would merit further study.
The value of this study is shown in several ways. First, the insight it provides into the conceptual frameworks used by experts in developing, testing, and describing current intelligence measurement practices. Second, the comparison of these frameworks provides an opportunity to make more explicit to researchers the challenges and potential future directions of intangibles measurement, specifically as they relate to intelligence outcomes in proving value or effectiveness. Third, the study is a step towards opening discourse to determine what commonly used terms mean to various intelligence groups, so that conceptual challenges to measurement can be addressed. The authors hope the juxtaposition of participants' current measurement practices with their critiques of the field and criteria for future measurement best practices will provide a valuable starting point to begin this conceptual discussion, which study participants indicate is needed in order to move research and practice forward.
This study will be followed up with additional research to determine the feasibility of outcome-based measurement for competitive intelligence services within organizations, where competitive intelligence is used in organizational decision-making processes. Additional research may include a survey of intelligence practitioners in various fields to determine how closely the comments of the experts in this study reflect the conceptualizations and practices of the larger field.
Sheila Wright and Stephen Marrin stated in their interviews that the current state of intelligence measurement is so problematic that it is essentially untaught in their university courses. This holds implications for the practices of graduates. We cannot be surprised that other audiences, whether they be managers or taxpayers, undervalue what is done in any intelligence field, when we as researchers and educators are unable to cogently express its value through comprehensible measurement. It is the hope of the authors that in providing a starting point for conceptual comparisons, this study may prompt conversations between researchers, students, and practitioners in this field that may lead toward consensus of terminology and conceptualization, research to investigate what outcomes should be used to supply data for measurement of intelligence activities, and formulation of best practice through systematic testing of measurement models and recommendations in the literature.
Acknowledgements
The authors would like to thank Sheila Wright, John Kringen, Stephen Marrin, Andrew Beurschgens, and Antti Lönnqvist for their participation in this study and their generosity and patience in discussing their practice, research, and reflections in the area of intelligence measurement. The authors also thank the reviewers at the 2013 i3 conference in Aberdeen who provided useful feedback on the first version of the paper, and the anonymous reviewer at Information Research who provided a thoughtful and helpful critique, and the journal's editors Charles Cole and Amanda Cossham. Rhiannon Gainor would like to acknowledge that this research was made possible by a provincial grant from Québéc's Fonds de Recherche Société et Culturelle (FQRSC) and a research and travel grant from McGill University.
About the authors
Rhiannon Gainor is a PhD candidate at the School of Information Studies, researching competitive intelligence, metrics for information services, and decision-making. She holds an MLIS and an MA from the University of Alberta. She can be reached at: rhiannon.gainor@mail.mcgill.ca.
France Bouthillier is an Associate Professor and the Director of the School of Information Studies at McGill. Her research interests include competitive intelligence, the information needs of small businesses, and digital resource assessment in the medical sector. She can be reached at: france.bouthillier@mcgill.ca.
Note
This paper is based on a presentation at the Information: Interactions and Impact (i3) Conference. This biennial international conference is organised by the Department of Information Management and Research Institute for Management, Governance and Society (IMaGeS) at Robert Gordon University. i3 2013 was held at Robert Gordon University, Aberdeen, UK on 25-28 June 2013. Further details are available at http://www.i3conference.org.uk/