An empirical analysis of mobile Internet acceptance in Chile
Patricio Esteban Ramírez-Correa
School of Business Sciences, Catholic University of the North, Coquimbo, Chile
Francisco Javier Rondán-Cataluña, and Jorge Arenas-Gaitán
Department of Business Management and Marketing, University of Seville, Seville, Spain
Introduction
The tempo and direction of the information systems discipline are set by the advanced economies of the world (Avgerou, 2008). Nonetheless, the need to understand whether theories developed in technologically advanced settings are applicable to technologically developing countries is an important issue for the discipline (Nasco, Toledo and Mykytyn, 2008).
Although Chile is a technological leader in Latin America (Everis..., 2012), it should not be considered as a technologically advanced country. It is still far from world technological leaders such as the Republic of Korea or Sweden (International..., 2013). Mobile phone penetration in Chile reached 95.7% of households in 2012. This is well above the Latin American average of 79.1%, but far from markets such as Hong Kong, which has a mobile phone penetration of 229% (Euromonitor International, 2013). Moreover, as a result of investments in infrastructure and the large number of smartphones operating in the country, Internet usage in Chile between 2007 and 2012 grew 63.8%, taking the population of Chileans using the Internet to 56.1%. This is fifteen percentage points above the average for Latin America, but still twenty percentage points below technologically advanced countries (Euromonitor International, 2013). Forecasts indicate that Chile will continue to advance technologically. In particular, mobile Internet has had a remarkable growth in recent years and the Chilean Government estimates that the demand for mobile Internet services will grow to eighteen times its current size in the next four years (Chile, 2012). Furthermore, cross-cultural studies including Chilean samples suggest that cultural distance is a moderator of information technologies’ acceptance (Alshare, Mesak, Grandon and Badri, 2011; Arenas-Gaitan, Ramirez-Correa and Rondan-Cataluna, 2011).
In recent years there has been a dramatic expansion of Internet users which is expected to continue over coming years. According to the International Telecommunication Union (2013) estimates, there were 6.8 billion mobile-cellular subscriptions by the end of 2013. Mobile Internet devices such as tablets and smartphones are one of the drivers of this spread of Internet access. In fact, the development of mobile technologies is changing the way users connect to the Internet and communicate interactively with others. Mobile Internet devices are used in various activities, including surfing the Internet, visiting social networking sites, using email, online gaming, reading reviews, geographic positioning and online shopping. Mobile Internet is much more common in advanced economies than in developing countries, with seventy-five per cent penetration in the former compared with twenty per cent in the latter. A major difference between developed and developing countries is that in developed countries mobile Internet is often a complement to, rather than a substitute for, fixed-broadband access. In developing countries, mobile broadband took off in 2010, and penetration rates will have increased from 4.4%to almost 20 % by the end of 2013. Understanding how mobile Internet is adopted in emerging markets will help accelerate the reduction of the digital divide.
The individual-level adoption of mobile Internet has been a focus of research in recent years (Cheong and Park, 2005; Choi, Seol, Lee, Cho and Park 2008; de Reuver, Ongena, and Bouwman, 2013; Gerpott, Thomas and Weichert, 2013; Hong, Thong and Tam, 2006; Hsu, Lu and Hsu, 2007; Kim, Chan and Gupta, 2007; Koenigstorfer and Groeppel-Klein, 2012; Lee, 2009; Liu and Li, 2010; Pedersen, 2005; Thong, Hong and Tam, 2011; Venkatesh, Thong and Xu, 2012; West and Mace, 2010). For the most part, these studies extended or adjusted models of information technology acceptance that had been developed originally for organizational settings. However, in many cases, these models by themselves are not satisfactory to explain the phenomenon of technology acceptance from the consumers’ perspective (Kim, et al., 2007). Recently, Venkatesh, et al. (2012) proposed the unified theory of acceptance and use of technology, version two (hereafter, ‘the theory’ or ‘the unified theory’) to explain the acceptance of mobile Internet. The theory was built based on past extensions of the unified theory of acceptance and use of technology (Venkatesh, Morris, Davis and Davis, 2003) in order to place particular emphasis on the context of consumer use. Because of the specific context of the orientation of the theory, it is sensitive to the characteristics of the consumer market. Therefore, the theory will be useful in consumer markets in technologically advanced countries, but is this approach appropriate to explain the phenomenon in less technologically advanced countries? (Venkatesh et al., 2012). Consequently, the need to understand whether the theory applies to populations in technologically developing countries is pertinent.
Therefore, given its pioneering experience in consumer technologies and the manifest digital divide with regards to technologically advanced countries, we believe that Chile is a good place to study the applicability of the unified theory. In addition, given the proximity between the Chilean culture and the cultures of other Latin America countries, we consider that the results of this analysis can help to understand the phenomenon of acceptance of mobile Internet in this increasingly important region of the world. In this context, the main objective of this paper is to examine the applicability of the unified theory of acceptance and use of mobile Internet in Chile.
There are two main contributions of this study. First, it provides an empirical validation of a recently proposed model which explains the acceptance of technologies in the consumer context, examining a sample of users more varied than used by Venkatesh et al. (2012), in relation to the proportionality of age and gender. Secondly, but equally important, it provides a unique analysis of the phenomenon of mobile Internet consumption in a technologically developing country and in the Latin American cultural context. The remainder of the paper is structured as follows. First, we present a literature review on the adoption of mobile Internet and of Chilean online consumer behaviour. Secondly, a research model is proposed and hypotheses are developed. Thirdly, the research method is described and study results are exhibited. Finally, we deliver a discussion, implications and conclusions.
Literature review
Adoption of mobile Internet
In the literature there are various approaches to research on the acceptance and use of mobile Internet. Some studies have applied the theory of diffusion of innovation (Rogers, 2003), which understands innovation diffusion as the process by which an innovation is communicated through certain channels over time among members of a social system. In addition, the theory explains that in this social system there are several categories of adopters: innovators, early adopters, early majority, late majority and laggards. Uses of diffusion of innovation theory in this context are diverse: (i) as a basis for developing hypotheses or models that explain the use of mobile Internet (de Reuver et al., 2013; Hsu, Lu and Hsu, 2008; Kim and Yang, 2012; Yamakami, 2006), (ii) as a theoretical element to discuss research findings (Gerpott, 2011; West and Mace, 2010), or (iii) to classify mobile Internet users (Okazaki, 2006).
Other studies have used models framed in the study of human behaviour such as the theory of reasoned action and the theory of planned behaviour (Ajzen, 1991; Ajzen and Fishbein, 1980). According to these theories, an individual's behaviour is determined by the intention to perform that behaviour. This intention is a function of attitude and subjective norms, which are preceded by beliefs about the behaviour and normative beliefs, respectively. The attitude represents individual feelings, positive or negative, on the completion of a specific behaviour, while the intent describes the strength of purpose of performing a specific behaviour (Ajzen and Fishbein, 1980). Moreover, beliefs about behaviour refer to the positive or negative evaluation of realization of a particular conduct by an individual and normative beliefs represent individual perception to social pressures to perform or not perform comportments. The relative weights of these two types of beliefs can vary among individuals (Ajzen and Fishbein, 1980). In particular, in the context of mobile Internet usage, the theory of reasoned action and theory of planned behaviour have been used as a theoretical basis for research models (Cheon, Lee, Crooks and Song, 2012; Hsiao, 2013), to integrate with others models (Shin and Choo, 2012) and to compare between countries (Shin, 2012).
A significant number of studies have used a model of information technology acceptance originally developed for organizational settings and widely validated, called the technology acceptance model (Davis, 1989). The technology acceptance model is influenced by the conceptual framework established by the theory of reasoned action and the theory of planned behaviour, and its purpose is to explain the process of acceptance of information technology at an individual level. The technology acceptance model's primary focus is on two theoretical ideas, perceived usefulness and perceived ease of use, which are identified as key determinants to explain the user's behavioural intention to use a technological innovation, particularly, to accept or to reject information technology. The perceived ease of use describes the degree of ease associated with the use of technology, and the perceived usefulness indicates the degree to which a person believes that using the technology will help to achieve performance improvements. Specifically, the technology acceptance model indicates that individual intention to use information technology is a function of perceived ease of use and perceived usefulness. Furthermore, the model notes that perceived ease of use directly affects the perceived utility. Examples of the use of the technology acceptance model in the context of mobile Internet include using it directly as the research model (Baek, Park and Lee, 2011; Coursaris and Sung, 2012), making extensions to the model (Kim et al., 2007; Lee, 2009; Park and del Pobil, 2013) and integrating it with other models to develop a research model (Lee and Chang, 2013; Zhou, 2011b).
Additionally there is a group of studies that used a research approach based on versions two and three of the technology acceptance model. Version two (Venkatesh and Davis, 2000) is a theoretical extension of the technology acceptance model that explains perceived usefulness and intention to use in terms of social influence (subjective norm, voluntariness and image) and cognitive instrumental processes (job relevance, output quality, result demonstrability and perceived ease of use). Version three (Venkatesh and Bala, 2008) is an extension of version two and emerges from an extensive analysis of the use of the technology acceptance model. Version three describes a set of determinants of perceived usefulness. The use of these models in the context of mobile Internet adoption has usually been empirically validating some variables and relationships (Abbasi, Chandio, Soomro and Shah, 2011; Vlachos, Giaglis, Lee and Vrechopoulos 2011) or integrating them with other models to propose a research model for the study (Leong et al., 2013a).
Another approach used in the study of the acceptance of mobile Internet is the unified theory of acceptance and use of technology (Venkatesh et al., 2003). This is a model that attempts to comprehensively explain why individuals use information technology at their disposal. It was developed as a synthesis of research on the acceptance of technologies available. The unified theory of acceptance and use of technology proposes four constructs (performance expectancy, effort expectancy, social influence and facilitating conditions) that affect the intended or actual use of technology. In the context of mobile Internet, this model has been validated empirically (Thong, Venkatesh, Xu, Hong and Tam, 2011; Wang and Wang, 2010) and integrated with other models (Mardikyan, Beşiroğlu and Uzmaya, 2012; Park, Yang, and Lehto, 2007; Zhou, Lu and Wang, 2010).
The most recent research approach used in mobile Internet is version two of the theory (Venkatesh et al., 2012), which seeks to adapt the unified theory of use and acceptance of technology to a consumer context. Version two integrates three new variables to explain the intention to use technology: hedonic motivation, price/value and habit. The theory can be explained as follows. By increasing expectation of performance, effort expectancy, social influence, facilitating conditions, hedonic motivation, price/value or habit, intent to use will increase. Additionally, an increase in intent to use, facilitating conditions or habit will directly increase the use of technology. Because of the novelty of the theory, the current literature references are mostly associated with the variables that it shares with the unified theory of use and acceptance of technology (Alwahaishi and Snasel, 2013; Chu, 2013; Gogus, Nistor, and Lerche, 2012; Leong et al., 2013b; Lin, Zimmer and Lee, 2013; Mantymaki and Salo, 2013; Nistor, 2013; Nistor, Gogus and Lerche, 2013; Nistor, Schworm and Werner, 2012), and just a few studies use the new variables proposed (Chong, 2013; Escobar-Rodríguez and Carvajal-Trujillo, 2013; LaRose, De Maagd, Chew, Tsai, Steinfield, Wildman and Bauer, 2012).
Chilean online consumer behaviour
There are very few academic publications studying business in Latin America (Donoso and Crittenden, 2008). In particular, studies about consumers' Internet behaviour are very limited (Andrews and Bianchi, 2013). However, in the case of Chile it is possible to indicate some features. First, in 2012 the percentage of the population using the Internet reached 61.4%, mobile Internet usage is also common (International..., 2013). Studies suggest that while there is an inverse relationship between age and perception and use of the Internet, Chilean consumers attach value to information technology irrespective of age or sex (Maldifassi and Canessa, 2009). In addition, and in relation to buying on the Internet, the attitude towards online shopping relates positively to the intention to buy online (Andrews and Bianchi, 2013).
Secondly, Chile is a collectivist culture (Hofstede, 2001); therefore Chileans are more influenced by other consumers. A more collectivist culture could be the explanation for some research findings on Chilean online consumer behaviour: (i) result demonstrability influences consumer attitudes towards online purchasing (Andrews and Bianchi, 2013); and (ii) social identification is highly predictive of e-commerce adoption (Arenas-Gaitán et al., 2013; Grandón, Nasco, and Mykytyn, 2011; Nasco et al., 2008).
Thirdly, Chile is a culture with high uncertainty avoidance (Hofstede, 2001); therefore Chileans request certainty in their daily life. Being a culture with a higher uncertainty avoidance could be the explanation for other research findings on Chilean online consumer behaviour: (i) perceived online risk negatively influences consumer attitudes towards online purchasing (Bianchi and Andrews, 2012); (ii) compatibility with values, buying patterns and past experiences influence attitudes towards continuing online purchasing (Andrews and Bianchi, 2013); (iii) perceived behavioural control does not influence the intention to adopt e-commerce (Grandón et al., 2011; Nasco et al., 2008); and (iv) Chilean users’ effort expectancy has no significant effect on the behavioural intention to use, or on the behaviour of online information technologies usage (Al-Khateeb, 2007; Andrews and Bianchi, 2013; Ramírez-Correa et al., 2013).
Research model and hypotheses
Research model
Figure 1 shows the research model based on the unified theory and the hypotheses of this study.
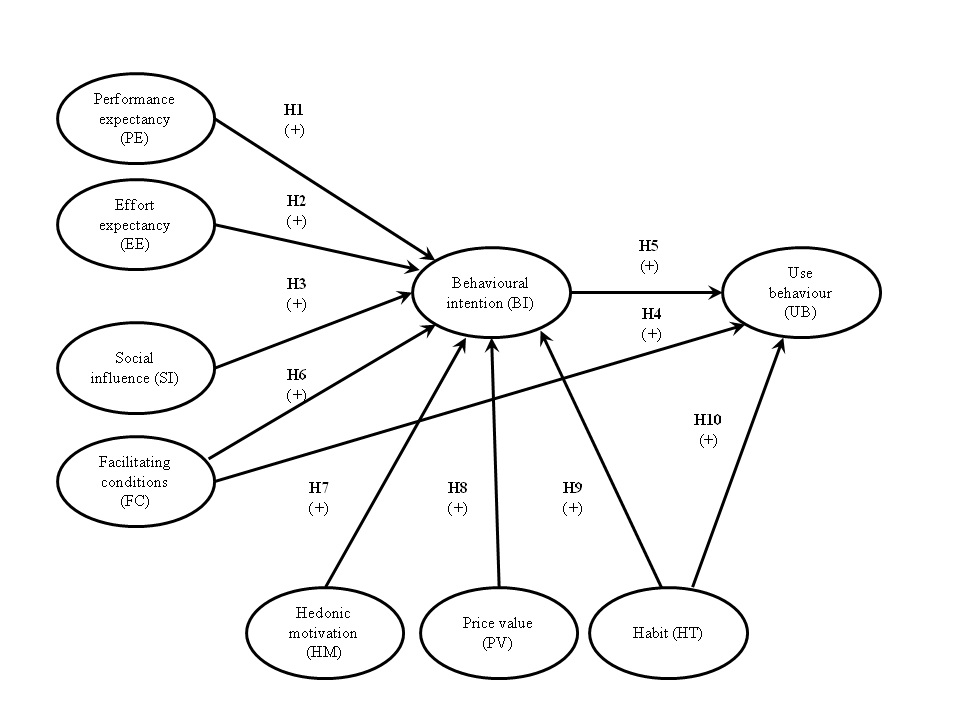
The research model aims to explain the acceptance of mobile Internet. In the model, use behaviour is explained, first, by the behavioural intention, as occurs in other contexts, and second, due to consumer context related to the phenomenon, by the facilitating conditions and habit. Moreover, behavioural intention is explained by two groups of antecedent variables. The first group is generally used in other contexts and it is composed of performance expectancy, effort expectancy and social influence. The second group is associated with the consumer context and is composed of hedonic motivation, price value , facilitating conditions and habit.
Hypotheses
The unified theory has been applied to explain the acceptance of mobile Internet (Mardikyan et al., 2012; Park and Kim, 2013; Shin and Choo, 2012; Thong, et al., 2011; Wang and Wang, 2010; Zhou, 2011a). Below we explain the hypotheses associated with this model.
Behavioural intention is the degree to which a person has formulated conscious plans to perform or not perform some specified future behaviour (Ajzen and Fishbein, 1980; Davis, 1989; Venkatesh, et al., 2003; Venkatesh, et al., 2012). Several studies specify variables that explain the increase in behavioural intention to use mobile Internet.
Some studies indicate that performance expectancy, defined as the degree to which using a technology will provide benefits to individuals in performing certain activities (Venkatesh, et al., 2003; Venkatesh, et al., 2012), affects behavioural intention in adopting mobile Internet (Mardikyan et al., 2012; Park and Kim, 2013; Thong, et al., 2011; Wang and Wang, 2010; Zhou, 2011a).
Also the literature suggests that effort expectancy, defined as the degree of ease associated with individuals’ use of technology (Venkatesh, et al., 2003; Venkatesh, et al., 2012), affects behavioural intention in adopting mobile Internet (Mardikyan et al., 2012; Thong, et al.. 2011; Wang and Wang, 2010).
Furthermore, various studies point out that social influence, defined as the extent to which an individual perceives that people who are important to him believe he should use a particular technology (Ajzen and Fishbein, 1980; Venkatesh, et al., 2003; Venkatesh, et al., 2012) - affects behavioural intention in adopting mobile Internet (Mardikyan et al., 2012; Shin and Choo, 2012; Thong, et al., 2011; Wang and Wang, 2010).
Finally, it is indicated that facilitating conditions, defined as the individual’s perceptions of the resources and support available to behave (Venkatesh, et al., 2003; Venkatesh, et al., 2012), affect use behaviour in adopting mobile Internet (Zhou, et al., 2010).
Considering the importance of a replica in a technologically developing country and with different cultural context, and based on these previous studies, the following hypotheses are proposed:
H1. Performance expectancy is positively related to behavioural intention in the adoption of mobile Internet in Chile.
H2. Effort expectancy is positively related to behavioural intention in the adoption of mobile Internet in Chile.
H3. Social influence is positively related to behavioural intention in the adoption of mobile Internet in Chile.
H4. Facilitating conditions are positively related to use behaviour in the adoption of mobile Internet in Chile.
The revised uniform theory has been applied to explain mobile Internet acceptance (Venkatesh, et al., 2012). Below we explain the hypotheses associated with this model.
Use behaviour is the frequency of information technology usage (Davis, 1989; Venkatesh and Bala, 2008; Venkatesh and Davis, 2000; Venkatesh, et al., 2003; Venkatesh, et al., 2012), and it has been used as the final consequence in all the models of information technology acceptance. The literature indicates that behavioural intention explains use behaviour in adopting mobile Internet (Venkatesh, et al., 2012).
Furthermore, the literature suggests that the facilitating conditions explain the increase in behavioural intention to use mobile Internet (Thong, et al., 2011; Venkatesh, et al., 2012). Several authors suggest that both behavioural intention and use behaviour in adopting mobile Internet are predicted by variables associated with the consumer context.
Some studies indicate that hedonic motivation, defined as the fun or pleasure derived from using a technology (Venkatesh, et al., 2012), affects behavioural intention towards adopting mobile Internet (Cheong and Park, 2005; Liu and Li, 2010; Thong, et al., 2006; Venkatesh, et al., 2012; Yang, Lu, Gupta and Cao 2012).
Additionally, various studies suggest that price value, defined as the individuals’ cognitive trade-off between the perceived benefits of the applications and the monetary cost for using them (Venkatesh, et al., 2012), affects behavioural intention towards adopting mobile Internet (Cheong and Park, 2005; Kim, et al., 2007; Mardikyan et al., 2012; Venkatesh, et al., 2012; Wang and Wang, 2010).
Finally, the literature indicates that habit - defined as the extent to which people tend to perform behaviours automatically because of learning (Venkatesh, et al., 2012) - affects both behavioural intention towards adopting mobile Internet (Venkatesh, et al., 2012) and use behaviour in the adoption of mobile Internet (Kim, et al., 2010).
Considering the importance of a replica in a technologically developing country, and based on these previous studies, the following hypotheses are proposed:
H5. Behavioural intention is positively related to use behaviour in the adoption of mobile Internet in Chile.
H6. Facilitating conditions are positively related to behavioural intention in the adoption of mobile Internet in Chile.
H7. Hedonic motivation is positively related to behavioural intention in the adoption of mobile Internet in Chile.
H8. Price value is positively related to behavioural intention in the adoption of mobile Internet in Chile.
H9. Habit is positively related to behavioural intention in the adoption of mobile Internet in Chile.
H10. Habit is positively related to use behaviour in the adoption of mobile Internet in Chile.
In addition, we will investigate the moderating effects proposed in the unified theory as we consider that there may be important differences with the results obtained in a developed market due to the cultural and technological gap. Specifically, we propose to explore the moderating effects of gender, age and experience in the adoption of mobile Internet in Chile.
Research method
Empirical research was based on a non-random sampling method. Quota sampling was used to select participants based on age range and gender. Quotas were selected according to the profile of Chilean Internet users (Ipsos, 2012). We chose this method because one advantage of quota sampling is that it provides sufficient statistical power to detect group differences. Data were collected in Chile in two major cities 900 kilometres apart through a face-to-face survey in November 2012. The exclusion of invalid interview schedules provided a final sample size of 501 mobile Internet users, 266 males and 235 females. 60% of the participants had more than one year of experience using mobile Internet. Table 1 shows the survey sample.
Age range | % all | % male | % female |
---|---|---|---|
13-14 | 5.6 | 2.4 | 3.2 |
15-19 | 15.4 | 8.8 | 6.6 |
20-24 | 16.0 | 8.0 | 8.0 |
25-34 | 25.3 | 13.4 | 12.0 |
35-44 | 18.6 | 10.0 | 8.6 |
45-59 | 15.2 | 8.0 | 7.2 |
60 and older | 4.0 | 2.6 | 1.4 |
Total | 100.0 | 53.1 | 46.9 |
The measurement scales used were similar to those proposed by Venkatesh, et al., (2012). All items were measured using a seven-point Likert scale. Experience was measured in months and age was measured in years. Sex was codified to 0 (Female) and 1 (Male). The interviews were conducted in Spanish. Before the use of the schedule, it was translated from English to Spanish and then back to English to assure equivalence in translation, then a pilot test was conducted with 130 mobile Internet users. The results indicated the reliability of the scales (see appendix with measurement instrument).
The partial least squares path model approach to structural equation modelling was applied to test the research model (Chin, 1998; Tenenhaus, Vinzi, Chatelin and Lauro, 2005). Specifically, SmartPLS 2.0 M3 software (Ringle, Wende and Will, 2005) was used for model analysis.
Results
A partial least squares path model is described by two models: (1) a measurement model relating the manifest variables to their own latent variables and (2) a structural model relating some endogenous latent variables to other latent variables.
Measurement model analysis
Previous to analysing the structural model, the reliability and validity of the measurement model were measured. For use behaviour the measurement method used is formative, for all other variables the measurement method used is reflective. For each reflective latent variable, the individual reliability was assessed examining the loads (λ) of the measures with their respective latent variable. The λ of all indicators should be 0.5 or above on their expected latent variable (Hair, Anderson and Tatham, 1986) and they should be significant at least at the 0.05 level (Fornell and Larcker, 1981). The λ of indicators for the research model were mostly above 0.7 (all above 0.5) and they were significant at the 0.001 level. For use behaviour the variance inflation factor was calculated in order to confirm that there was no collinearity between the different indicators. In all cases the values remained below the recommended maximum value of 3.3 (Cenfetelli and Bassellier, 2009). Table 2 shows the results from the cross-loading procedure.
Indicator | BI | PE | EE | SI | FC | HD | HT | PV | UB | p | VIF |
---|---|---|---|---|---|---|---|---|---|---|---|
Behavioural intention 1 | 0.89 | -0.02 | 0.06 | -0.02 | 0.07 | -0.04 | -0.25 | -0.01 | 0.03 | <0.001 | |
Behavioural intention 2 | 0.90 | -0.03 | -0.06 | 0.04 | -0.06 | 0.01 | 0.33 | 0.01 | -0.06 | <0.001 | |
Behavioural intention 3 | 0.94 | 0.04 | 0.00 | -0.02 | -0.02 | 0.03 | -0.08 | 0.00 | 0.02 | <0.001 | |
Performance expectancy 1 | 0.11 | 0.91 | 0.11 | -0.03 | -0.01 | 0.09 | -0.02 | -0.05 | 0.03 | <0.001 | |
Performance expectancy 2 | 0.03 | 0.91 | 0.00 | -0.05 | -0.02 | 0.09 | -0.09 | 0.01 | -0.01 | <0.001 | |
Performance expectancy 3 | -0.16 | 0.82 | -0.12 | 0.09 | 0.04 | -0.20 | 0.11 | 0.04 | -0.02 | <0.001 | |
Effort expectancy 1 | -0.09 | 0.06 | 0.93 | 0.02 | 0.05 | -0.02 | 0.01 | -0.03 | -0.01 | <0.001 | |
Effort expectancy 2 | 0.07 | 0.04 | 0.91 | -0.02 | -0.06 | 0.00 | -0.05 | 0.09 | 0.02 | <0.001 | |
Effort expectancy 3 | -0.04 | 0.03 | 0.92 | -0.03 | 0.00 | 0.07 | -0.04 | -0.03 | 0.05 | <0.001 | |
Effort expectancy 4 | 0.07 | -0.14 | 0.86 | 0.03 | 0.01 | -0.06 | 0.09 | -0.03 | -0.07 | <0.001 | |
Social influence 1 | -0.02 | 0.02 | -0.01 | 0.94 | 0.04 | -0.01 | -0.08 | 0.03 | 0.01 | <0.001 | |
Social influence 2 | -0.03 | -0.01 | 0.01 | 0.96 | -0.02 | -0.02 | 0.06 | -0.04 | -0.04 | <0.001 | |
Social influence 3 | 0.04 | -0.01 | -0.01 | 0.94 | -0.02 | 0.03 | 0.01 | 0.01 | 0.04 | <0.001 | |
Facilitating conditions 1 | -0.11 | 0.04 | 0.36 | -0.02 | 0.85 | -0.04 | -0.02 | -0.05 | -0.01 | <0.001 | |
Facilitating conditions 2 | -0.50 | -0.08 | -0.08 | 0.02 | 0.79 | -0.08 | 0.22 | 0.05 | 0.02 | <0.001 | |
Facilitating conditions 3 | 0.65 | 0.04 | -0.32 | 0.00 | 0.74 | 0.13 | -0.22 | 0.00 | -0.01 | <0.001 | |
Hedonic motivation 1 | 0.02 | -0.02 | 0.03 | 0.00 | -0.02 | 0.95 | 0.03 | -0.06 | -0.03 | <0.001 | |
Hedonic motivation 2 | 0.02 | 0.05 | -0.02 | 0.00 | 0.02 | 0.93 | -0.04 | 0.02 | 0.02 | <0.001 | |
Hedonic motivation 3 | -0.04 | -0.04 | -0.01 | 0.00 | 0.00 | 0.92 | 0.01 | 0.05 | 0.01 | <0.001 | |
Habit 1 | 0.15 | 0.07 | 0.04 | -0.10 | 0.07 | -0.01 | 0.88 | -0.06 | 0.00 | <0.001 | |
Habit 2 | -0.23 | -0.07 | 0.01 | -0.01 | -0.07 | 0.05 | 0.90 | -0.02 | 0.02 | <0.001 | |
Habit 3 | 0.09 | 0.01 | -0.03 | 0.11 | 0.00 | -0.04 | 0.86 | 0.08 | -0.03 | <0.001 | |
Price value 1 | -0.15 | -0.03 | 0.04 | -0.01 | -0.09 | -0.06 | 0.05 | 0.94 | 0.04 | <0.001 | |
Price value 2 | -0.27 | 0.01 | 0.06 | 0.04 | -0.10 | -0.04 | 0.11 | 0.93 | -0.02 | <0.001 | |
Price value 3 | 0.76 | 0.07 | -0.19 | -0.05 | .34 | 0.19 | -0.28 | 0.51 | -0.03 | <0.001 | |
Use behaviour 1 | 0.07 | -0.14 | -0.18 | 0.05 | 0.20 | 0.12 | -0.31 | -0.01 | 0.51 | <0.001 | 1.07 |
Use behaviour 2 | -0.23 | -0.37 | 0.01 | 0.13 | -0.05 | 0.47 | 0.21 | -0.11 | 0.54 | <0.001 | 1.10 |
Use behaviour 3 | 0.09 | 0.12 | -0.03 | -0.07 | 0.03 | -0.09 | 0.05 | 0.01 | 0.81 | <0.001 | 1.36 |
Use behaviour 4 | 0.03 | 0.27 | 0.16 | -0.06 | -0.14 | -0.36 | 0.01 | 0.09 | 0.67 | <0.001 | 1.26 |
Loadings (reflective items) and weights (formative items) are shown in bold; loadings and cross-loadings are oblique-rotated; p-values refer to loadings and were obtained through bootstrapping with 100 resample; VIF, variance inflation factor for indicator |
The reliability of the reflective latent variable indicates how rigorous observed variables are measuring the same reflective latent variable, Cronbach's α coefficient was used as the index of reflective latent variable reliability (CA ≥0.7 were accepted). In addition, composite reliability was calculated. The latent variable convergent validity was assessed by examining the average variance extracted (AVE > 0.5 were accepted) (Fornell and Larcker, 1981). To test for multi-collinearity, the variance inflation factor was calculated for each latent variable (all were less than 3.1). Table 3 shows the variance inflation factor, Cronbach's α coefficient, composite reliability and average variance extracted for each latent variable.
Latent variable | VIF | CR | CA | AVE |
---|---|---|---|---|
Behavioural intention | 3.09 | 0.93 | 0.89 | 0.82 |
Performance expectancy | 2.40 | .91 | .86 | .78 |
Effort expectancy | 2.54 | .95 | .93 | .82 |
Social influence | 1.30 | 0.96 | 0.94 | 0.89 |
Facilitating conditions | 3.08 | 0.84 | 0.70 | .63 |
Hedonic motivation | 2.25 | 0.95 | 0.92 | 0.87 |
Habit | 2.55 | 0.91 | 0.85 | 0.77 |
Price value | 1.44 | 0.85 | 0.72 | 0.67 |
Use behaviour | 1.44 | Formative | ||
CR, composite reliability coefficient for latent variable; CA, Cronbach’s a coefficient for latent variable; VIF, variance inflation factor for latent variable; AVE, average variances extracted for latent variable |
The latent variable discriminant validity was tested by analysing if the square root of the average variance extracted from each latent variable is greater than the correlations with the rest of the latent variable (see Table 4).
Variable | BI | PE | EE | SI | FC | HM | HT | PV | UB |
---|---|---|---|---|---|---|---|---|---|
Behavioural intention | 0.91 | ||||||||
Performance expectancy | 0.62 | 0.88 | |||||||
Effort expectancy | 0.51 | 0.63 | 0.91 | ||||||
Social influence | 0.40 | 0.36 | 0.18 | 0.94 | |||||
Facilitating conditions | 0.66 | 0.62 | 0.73 | 0.29 | 0.79 | ||||
Hedonic motivation | 0.60 | 0.59 | 0.61 | 0.29 | 0.67 | 0.93 | |||
Habit | 0.74 | 0.61 | 0.46 | 0.41 | 0.52 | 0.50 | 0.88 | ||
Price value | 0.47 | 0.42 | 0.31 | 0.33 | 0.41 | 0.46 | 0.44 | 0.82 | |
Use behaviour | 0.44 | 0.47 | 0.41 | 0.14 | 0.44 | 0.46 | 0.42 | 0.27 | NA |
Square roots of average variances extracted shown on diagonal. All correlations are significant at p < 0.001. |
Structural model analysis
Next, once validity and reliability of the measurement model were supported, relationships between constructs were tested. The hypotheses were assessed by examining path coefficients (β) and their significance levels. Bootstrapping with 100 subsamples was performed to test the statistical significance of each path coefficient using t-tests. The variance explained (R2) in the endogenous latent variable and the regression coefficients’ significance (F-test) serve as indicators of the explanatory power of the model. As shown in Table 5, the model fit indices were calculated to globally assess the model.
Index | Value | p |
---|---|---|
Average of path coefficient (APC) | 0.175 | <0.001 |
Average of square root (ARS) | 0.463 | <0.001 |
Average of variance inflation factor (AVIF) | 2.125 | |
Average of average variances extracted (AAVE) | 0.781 | |
Goodness of fit (GoF) | 0.601 |
The main results of partial least squares analyses to test the model are shown in Figure 2. All the results of partial least squares analyses for the model are shown in Table 6 and Table 7, including moderator effects of age, sex and experience.
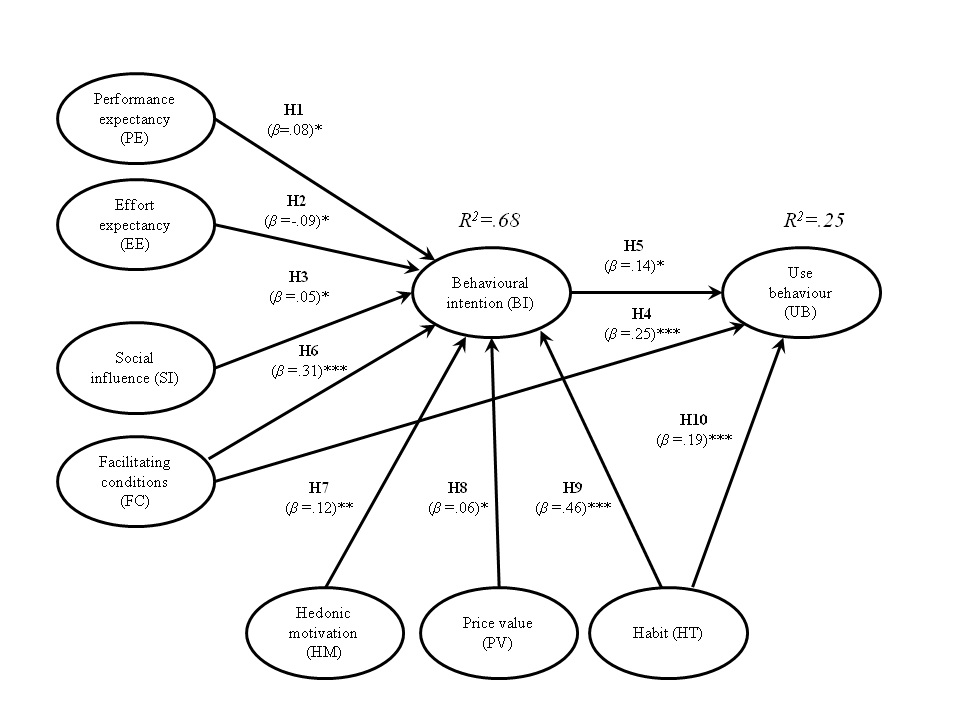
Dependent variable: use behaviour | D only | ES | D + I | ES |
---|---|---|---|---|
R2 | 0.25 | 0.29 | ||
Q2 | 0.25 | 0.28 | ||
Behavioural intention (BI) | 0.14 * | 0.06 | 0.19 ** | 0.08 |
Habit (HT) | 0.19 *** | 0.08 | 0.17 * | 0.07 |
Facilitating conditions (FC) | 0.25 *** | 0.11 | 0.17 ** | 0.07 |
Age (AGE) | -0.07 | 0.01 | ||
Sex (SEX) | -0.02 | 0.00 | ||
Experience (EXP) | -0.02 | 0.00 | ||
BI × EXP | -0.02 | 0.00 | ||
SEX x AGE | -0.06 | 0.01 | ||
AGE × EXP | 0.00 | 0.00 | ||
SEX × EXP | 0.11 + | 0.01 | ||
SEX × AGE × EXP | -0.08 | 0.00 | ||
HT × SEX | -0.02 | 0.00 | ||
FC × AGE | 0.07 | 0.02 | ||
FC × EXP | -0.02 | 0.00 | ||
HT × AGE | 0.03 | 0.01 | ||
HT × EXP | 0.07 | 0.01 | ||
HT × SEX × AGE | 0.02 | 0.00 | ||
HT × SEX × EXP | -0.08 | 0.01 | ||
FC × AGE × EXP | 0.01 | 0.00 | ||
HT × AGE × EXP | -0.01 | 0.00 | ||
HT × SEX × AGE × EXP | 0.02 | 0.00 | ||
D only: direct effects only; D+I: direct effects and interaction terms; ES: effect size; *** p < 0.001; ** p < 0.01; * p <0.05; + p <0.10 |
Dependent variable: behavioural intention | D only | ES | D + I | ES |
---|---|---|---|---|
R2 | 0.68 | 0.73 | ||
Q2 | 0.68 | 0.73 | ||
Social influence (SI) | 0.05 * | 0.02 | 0.05 + | 0.02 |
Habit (HT) | 0.46 *** | 0.34 | 0.44 *** | 0.33 |
Hedonic motivation (HM) | 0.12 ** | 0.07 | 0.16 *** | 0.09 |
Effort expectency (EE) | -0.09 * | 0.04 | -0.04 | 0.02 |
Performance expectency (PE) | 0.08 * | 0.05 | 0.06 | 0.04 |
Facilitating conditions (FC) | 0.31 *** | 0.21 | 0.33 *** | 0.22 |
Price value (PV) | 0.06 * | 0.03 | 0.02 | 0.01 |
Age (AGE) | 0.10 * | 0.00 | ||
Sex (SEX) | -0.02 | 0.00 | ||
Experience (EXP) | 0.13 * | 0.03 | ||
SEX x AGE | -0.05 | 0.00 | ||
AGE × EXP | -0.18 * | 0.01 | ||
SEX × EXP | -0.07 | 0.01 | ||
SEX × AGE × EXP | 0.17 * | 0.02 | ||
PE × SEX | 0.02 | 0.00 | ||
SI × SEX | 0.05 * | 0.00 | ||
FC × SEX | 0.01 | 0.00 | ||
HM × SEX | 0.00 | 0.00 | ||
PV × SEX | -0.03 | 0.00 | ||
HT × SEX | 0.01 | 0.00 | ||
PE × AGE | 0.10 | 0.02 | ||
EE × AGE | 0.08 | 0.02 | ||
SI × AGE | -0.03 | 0.00 | ||
FC × AGE | 0.10 | 0.01 | ||
HM × AGE | -0.06 | 0.01 | ||
PV × AGE | 0.00 | 0.00 | ||
HT × AGE | 0.03 | 0.00 | ||
EE × EXP | 0.07 | 0.01 | ||
SI × EXP | -0.12 * | 0.01 | ||
FC × EXP | 0.03 | 0.00 | ||
HM × EXP | -0.02 | 0.00 | ||
HT × EXP | -0.08 | 0.01 | ||
PE × SEX × AGE | 0.10 | 0.01 | ||
EE × SEX × AGE | 0.08 | 0.01 | ||
SI × SEX × AGE | 0.06 | 0.00 | ||
FC × SEX × AGE | -0.16 + | 0.01 | ||
HM × SEX × AGE | 0.05 | 0.00 | ||
PV × SEX × AGE | -0.02 | 0.00 | ||
HT × SEX × AGE | -0.02 | 0.00 | ||
EE × SEX × EXP | -0.12 | 0.02 | ||
SI × SEX × EXP | 0.05 | 0.00 | ||
FC × SEX × EXP | -0.05 | 0.01 | ||
HM × SEX × EXP | 0.09 | 0.01 | ||
HT × SEX × EXP | 0.05 | 0.00 | ||
EE × AGE × EXP | -0.14 | 0.03 | ||
SI × AGE × EXP | -0.04 | 0.00 | ||
FC × AGE × EXP | 0.26 + | 0.03 | ||
HM × AGE × EXP | -0.09 | 0.02 | ||
HT × AGE × EXP | 0.14 | 0.01 | ||
EE × SEX × AGE × EXP | 0.15 | 0.02 | ||
SI × SEX × AGE × EXP | -0.05 | 0.00 | ||
FC × SEX × AGE × EXP | -0.17 | 0.01 | ||
HM × SEX × AGE × EXP | 0.02 | 0.00 | ||
HT × SEX × AGE × EXP | -0.03 | 0.00 | ||
D only: direct effects only; D+I: direct effects and interaction terms; ES: effect size; *** p < 0.001; ** p < 0.01; * p <0.05; + p <0.10 |
The model explains 73% of the variation in behaviour intention and 29% of the variation in behaviour of mobile Internet usage. In addition, effect sizes of all relationships are above the threshold minimum, highlighting the effect size of the variable habit.
The results support hypotheses 1, 3, 4, 5, 6, 7, 8, 9 and 10. However, hypothesis 2 is not supported. Table 8 summarizes the findings.
Hypothesis | Dependent variables | Independent variables | Moderators |
---|---|---|---|
H1 | Performance expectancy | Behavioural intention | |
H3 | Social influence | Behavioural intention | Sex, experience |
H4 | Facilitating conditions | Use behaviour | |
H5 | Behavioural intention | Use behaviour | |
H6 | Facilitating conditions | Behavioural intention | Sex, experience, age |
H7 | Hedonic motivation | Behavioural intention | |
H8 | Price value | Behavioural intention | |
H9 | Habit | Behavioural intention | |
H10 | Habit | Use behaviour |
Discussion
Overall, the study results indicate that the unified theory model is valid in Chile. The exception to this statement is the relationship between effort expectancy and behavioural intention (H2), a slightly negative relationship, contrary to the proposal made in the research model. This indicates that when mobile Internet is perceived easier to use, intention to use mobile Internet decreases. One explanation for this result may be associated with the cultural characteristics of Chile; the literature suggests that a high index of uncertainty avoidance is correlated with a low or non-significant relationship between effort expectancy and behavioural intention (Cardon and Marshall, 2008). Consistent with this explanation, other empirical studies of acceptance of online technologies in samples of Chilean users have delivered results where this relationship was non-significant (Al-Khateeb, 2007 ; Andrews and Bianchi, 2013; Ramírez-Correa, et al., 2013). Moreover, a recent study that applied the theory in a context of online consumer behaviour in Spain (Escobar-Rodríguez and Carvajal-Trujillo, 2013), a country with an index of uncertainty avoidance similar to Chile, indicated a negative relationship between these two variables, although this was non-significant. Considering these facts, we believe that this relationship should be closely studied in the future.
Research findings indicate that the variables that increase behavioural intention towards mobile Internet usage are, in order of importance: habit; facilitating conditions; hedonic motivation; performance expectancy; price value; and social influence. Among these variables the first two are of huge relevance. Therefore, the automatic and continuing use of mobile Internet generates greater intention towards mobile Internet usage, and the user’s perception of the resources and support available to use mobile Internet is essential to increasing behavioural intention towards mobile Internet usage. Moreover, research findings indicate that the variables which increase mobile Internet usage are, in order of importance: habit; behavioural intention; and facilitating conditions. Again, habit appears as a relevant precedent to explain the acceptance of mobile Internet in Chile. Given the recent proposal of this variable as an explanation of both the intention to use mobile Internet and mobile Internet usage, we believe that this result is an important finding that may help explain how people adopt such technologies in Latin American countries.
We would like to highlight the role of habit in the model. According to Venkatesh et al. (2012), habit has been defined as the degree to which individuals tend to perform behaviour mechanically because of learning (Limayem, Hirt and Cheung, 2007), while Kim and Mallhostra (2005) associate habit with automaticity. This includes the idea that former use is an important predictor of future behaviour (Kim and Mallhostra, 2005). From this point of view, these results are very consistent, since in the case of mobile Internet consumers already had the habit of using both Internet and mobile phones. The new technology, mobile Internet, only combines two habits that consumers already maintained.
Finally, we highlight the results in relation to the moderations that have been discovered in the analysis of the model. According to these results the relationship between social influence and behavioural intention is moderated, firstly by gender and secondly by experience. The effect of social influence on behavioural intention increases slightly in men and decreases with the experience of using mobile Internet. For this relationship, moderation of gender and experience together has been described in the literature (Thong, et al., 2011; Venkatesh, et al., 2012), but not independently.
Similarly, the relationship between facilitating conditions and behavioural intention is moderated, first, by age and experience, and second, by gender and age. The effect of facilitating conditions on behavioural intention increases in older people with more experience of using mobile Internet, and also increases in the case of older women, regardless of their experience in the use of mobile Internet. This latter moderation is consistent with the original study outlining the unified theory (Venkatesh, et al., 2012).
Overall, the result of the exploration of the potential moderations of the variables sex, age and experience in the acceptance of mobile Internet in Chile indicates a quite homogeneous consumer market.
Implications
From a theoretical point of view, this research validates a novel model of factors influencing the adoption of technology in a consumer context in Chile. In this sense, the main theoretical contribution of this research is to dismiss the hypothesis that, in a consumer setting of a country with high uncertainty avoidance index, when effort expectation decreases, intention to use technology increases. Another major contribution is the importance of habit as a predictor of individual behaviour in consumer technology acceptance; this result strengthens research findings in the context of technologically advanced countries (Limayem and Hirt, 2003).
Beyond the aforementioned implications associated to the replica, this study has other implications. Accepting the positivism paradigm which compels us to search for more thorough explanations of the phenomenon of mobile Internet acceptance, and given that the around 70% of the variance in behaviour intention is explained in the model, it is imperative to both elicit new variables and seek out new relationships between variables in order to increase this percentage. In regard to the former, study findings suggest that these new variables can be associated with cultural dimensions; one particular path to find these variables is to test the research model from this study in countries culturally similar to Chile, such as Argentina, Mexico and Venezuela (Schwartz, 2006). In regards to the latter, it is important to conceptually develop and assess non-linear relationships between behaviour intention and the variables which explain it.
This research further provides practical implications for the marketing of mobile Internet in Chile and other culturally similar countries. Firstly, the market for mobile Internet in Chile is relatively homogeneous, therefore consumers should respond similarly to advertising campaigns incentivising the adoption of this technology. An important exception to this rule is the group of older consumers with experience in the use of technologies, as they will be much more responsive to improvements associated with the resources and support available for using mobile Internet.
Secondly, habit is a fundamental variable in predicting intention to use mobile Internet and mobile Internet usage. As we know that this habit is achieved through learning, developing strategies to improve early and ongoing access to this technology may be a cornerstone for increasing its use. An illustration of a good strategy to create the habit could be the development of applications that require continuous use of mobile Internet and that have a high value for customers. Thirdly, effort expectancy and performance expectation, common variables in explaining the use of technology in organizational settings are not important predictors of mobile Internet use at a consumer level. Therefore, companies should observe other variables when trying to encourage mobile Internet usage, for instance, the hedonic motivations associated with its use. Finally, both social influence and price/value, which could be of great impact on the intention towards mobile Internet usage in a collectivist culture and an emerging economic market, appear in our results in a third level of relevance. In fact, social influence has a significant impact on the start of mobile Internet usage. However, this impact disappears with increasing experience in its use. Hence, companies can attract new users through social influence, but this influence does not ensure its permanence.
Conclusions
This research was devised to test the applicability of an information technology acceptance model in a technologically developing country. The revised unified theory was applied in a proportional sample of mobile Internet users in Chile.
In summary, we can affirm that Chilean acceptance behaviour of mobile Internet technology matches global observations according to the theory. Specifically, on one hand, the measurement model was validated successfully, and on the other hand, the structural model supported nine of the ten hypotheses proposed, achieving a 73% explanation of the intention to use mobile Internet. Therefore, the increase in mobile Internet usage is explained by the variables habit, facilitating conditions, hedonic motivation, performance expectancy, price value and social influence. The exploration of the potential moderations of the variables sex, age and experience in the acceptance of mobile Internet in Chile indicates a quite homogeneous consumer market.
The study findings highlighted habit as the major determinant in the acceptance of mobile Internet. We believe this is an interesting topic for future research, in particular the study of variables that moderate the effect of habit in the acceptance of information technologies. Additionally, another finding of this study is the unusual effect of effort expectancy in mobile Internet acceptance. This is an issue that should be analysed in future research.
Finally, we point out two limitations of this study. First, the use of a non-random sampling method limits the generalization of findings. Secondly, the study is cross sectional, a longitudinal study would be advisable to compare the different stages of the adoption of mobile Internet.
Acknowledgements
We would like to thank anonymous reviewers and the Associate Editor Dr. Charles Cole for their insightful comments. The authors would like to extend their gratitude to Professor Dr. Felix Tan, Head of the Department of Business Information Systems, Auckland University of Technology, New Zealand, for his support on this study during post-doctoral stay of the first author. This research is partially supported by the Catholic University of the North, Chile.
About the authors
Patricio Esteban Ramírez-Correa is Associate Professor at the School of Business Sciences of Catholic University of the North in Coquimbo, Chile. He received his BS degree in Computer from Pontifical University Catholic of Valparaiso, Chile and his PhD degree in Business Administration from University of Seville, Spain. His research interests include enterprise systems and information technology acceptance. He can be reached at patricio.ramirez@ucn.cl
Francisco Javier Rondán-Cataluña is Associate Professor at the Department of Business Management and Marketing at the University of Seville, Spain. He received his BA and PhD degrees in Business Administration from University of Seville, Spain. His research interests include franchise, price management and consumer behaviour. He can be reached at rondan@us.es
Jorge Arenas-Gaitán is Assistant Professor at the Department of Business Management and Marketing at the University of Seville, Spain. He received his BA and PhD degrees in Business Administration from University of Seville, Spain. His research interests include behaviour of firms in global markets, cross-cultural studies and information technology acceptance. He can be reached at jarenas@us.es