The interplay between knowledge gap and perceived risk in motivating risk information seeking
Shadi Shakeri, Nicholas Evangelopoulos and Oksana Zavalina
Introduction. This study proposes a theoretical framework for the complex interplay between knowledge gap and perceived risk as motivators of information seeking. Given the shortage of studies that use quantitative research methods to support theory-building in information behaviour research, the survey method is adopted and two models with alternative configurations are examined.
Method. Since the two proposed models involve relationships between latent constructs, survey items were developed to operationalise the constructs and statistical tests were performed to examine the associated hypotheses. Data were collected from a large public university through a scenario-based online survey. In order to avoid confounding effects, participants were recruited from a variety of educational levels, age groups, and socioeconomic backgrounds. Eight scenarios were randomly assigned across the participants. A total of 289 responses were used for analysis.
Analysis. The two models were tested using partial least squares structural equation modelling (PLS-SEM), a commonly used method for theory development in exploratory social sciences research.
Results. In model one, where perceived risk and knowledge gap are assumed to have direct effects on motivation to seek information, both direct effects are significant. In model two, where perceived risk is assumed to have a moderating effect on the relationship between knowledge gap and motivation to seek information, knowledge gap interacts with perceived risk to motivate information seeking. Given that model two has a better fit than model one, the results indicate that perceived risk and knowledge gap play a rather complex role in information-seeking behaviour. Model two proposes the risk-gap interaction hypothesis (RGIH), which states that risk perceptions interact with knowledge gap to motivate information seeking.
Conclusions. The findings suggest that, in the presence of the interaction between perceived risk and knowledge gap, as suggested by the risk-gap interaction hypothesis, the well-established direct effect between knowledge gap and motivation to seek information becomes insignificant. Therefore, the interaction between perceived risk and knowledge gap is of primary importance in motivating information seeking.
Introduction
One of the characters in Sir Arthur Conan Doyle’s The Hound of the Baskervilles, Dr. James Mortimer, describes the relationship between knowledge, predictability and perceived danger as follows: ‘that which is clearly known hath less terror than that which is but hinted at and guessed’ (Conan Doyle, 2009, p. 12). As humans, we try to adapt, survive and succeed in a world that is changing fast and is becoming less predictable at an accelerated pace. Examples of uncertainties that we face in everyday life include the occurrence of a bank crisis, the emergence of new fashions and the appearance of new technologies (Bentley, Earls and O’Brien, 2011, p. 123). As we navigate our knowledge gaps, uncertainties, fears, incoherence and other inadequacies, we are able to identify threats and opportunities, manage our perceived risks and fears, and enhance the quality of our judgments, decisions and behaviour (Fischhoff, Slovic, Lichtenstein, Read and Combs, 1978; Peters and Slovic, 2000; Slovic, 1987; Slovic, Finucane, Peters and Macgregor, 2004). The theories of uncertainty reduction and uncertainty management hold that humans naturally and intuitively attempt to reduce uncertainty to create coherence and meaning in their lives, and cope with and adapt to environmental stressors (Bradac, 2001). Successful uncertainty reduction may lead to increased predictability of the behaviour, and a subsequent decrease in information needs (Brashers, Neidig, Hass, Dobbs, Cardillo and Russell, 2000).
Information behaviour (IB) research is primarily concerned with describing the ways humans seek and utilise information (Bates, 2010). Julien, Pecoskie and Reed (2011) define the scope of information behaviour research to include information needs and information uses. According to Wilson (1999), information behaviour is the umbrella term that includes information-seeking and information-searching behaviour. The number of information behaviour research publications has grown exponentially, and it has gained its own identity as a sub-field of information science (IS) (Milojevic, Sugimoto, Yan and Ding, 2011). However, even though information behaviour research has drawn from theoretical work (González Teruel, González Alcaide, Barrios and Abad-Garcia, 2015), the theoretical landscape in information behaviour has suffered from a lack of in-depth analysis of models and theories, with many previously developed theoretical models not being properly tested and validated (Greifeneder, 2014; Vakkari, 2008). Classic models of information behaviour cover a range of different aspects, from what motivates individuals to begin a search process to how the obtained information is used for conducting everyday life or performing specific tasks. Research models that appear under the information behaviour umbrella are often complementary. For example, Ellis’s (1989) model is concerned with a searcher’s behaviour in actual search activities; while Kuhlthau’s (1991) model includes Ellis’s information search activities, as well as affective and cognitive stages of a search process. However, the classic models do not provide a complete picture. For instance, Wilson’s (1999) second model, which is an expansion of his 1981 model, suggests three overarching theories, namely, stress coping theory, risk-reward /theory, and social learning theory, as activating mechanisms of information behaviour. While one may understand the focus and application of these theories, Wilson does not sufficiently explain how these theories would function as antecedents of information behaviour. Niedzwiedzka’s (2003) study presents a critical description of Wilson’s second model, suggesting that the constructs of perceived risk, hope for reward and perceived level of self-efficacy should replace the names of the aforementioned theories. To our knowledge, these constructs have not been empirically investigated to the full extent.
A review of the information science literature reveals the importance of uncertainty as a motivating factor for information behavior. Atkin (1973) examines the role of extrinsic uncertainty (e.g., cognitive, behavioural, and defensive adaptations) as an extrinsic determinant of information seeking for utilitarian purposes (i.e., problem solving). Krikelas (1983) emphasises the importance of uncertainty in motivating individuals to seek information, explaining that when persons are in a need-creating environment where they become aware of the state of uncertainty, they attempt to reduce that uncertainty to an acceptable level by retrieving information from both their memory and nearby individuals. He describes how uncertainty may be associated with a specific event or an ongoing process associated with life, work, or both. Krikelas (1983, p.9) defines information seeking as ‘an attempt to continually construct a cognitive environmental ‘map’ to facilitate the need to cope with uncertainty’. Uncertainty is associated with negative feelings such as doubt, anxiety, and frustration (Kuhlthau, 1991; 2005). Kuhlthau (1993) proposes that in the initiation stage of any search process, which is also part of the pre-focus phase in Cole’s (2011; 2012, p.92) model, individuals experience feelings of uncertainty and apprehension when they become aware of a gap in knowledge. Uncertainty is also defined as ‘a lack of knowledge or as an aspect of knowledge that implies a degree of unknowability’ (Kouw, Van Den Heuvel, and Scharnhorst, 2013, p. 89). The present paper refers to this lack of knowledge as knowledge gap, a term also used in Case (2012, p. 113), and defines it as the realisation of a deficiency in an individual’s state of knowledge regarding a given situation or problem. This view of knowledge gap is consistent with Belkin’s notion of anomalous state of knowledge (ASK), which describes ‘inadequacies in a state of knowledge… such as gaps or lacks, uncertainty, or incoherence’ (Belkin, 1980, p. 137). It is also compatible with Wilson’s ‘recognition of some need, perceived by the user’ (Wilson, 2006, p. 659), and Bowler’s metacognitive knowledge, or ‘knowing that you do not know’ (Bowler 2011, p. 109).
The concept of knowledge gap as defined in this study is related to the notion of information insufficiency, used in the risk information seeking and processing model (RISP), (Griffin, Dunwoody and Neuwirth, 1999), and the planned risk information seeking model (PRISM) (Hovick, Kahlor and Liang 2014; Kahlor, 2010). Information insufficiency is defined as the difference between individuals’ current knowledge and the amount of information they perceive is needed, in order to deal with a given situation (i.e., information sufficiency). However, knowledge gap as defined here, refers to the realisation of such a difference. In the view of information insufficiency, a more cognitive approach to information gap is adopted, leaving the affective elements aside. The present study draws on the classic conceptualisation of knowledge gap as a cognitive-affective motivator for information seeking.
Uncertainty and risk are interconnected. Following Sitkin and Pablo (1992, p.10), risk is defined as ‘the extent to which there is uncertainty about whether potentially significant and/or disappointing outcomes of decisions will be realized’. Based on this definition, risk is related to the uncertainty associated with negative outcomes (Libby and Fishburn, 1977). Following Slovic (1987) and Kahneman (2011, p. 139), the present paper refers to intuitive judgements individuals make when they are asked to evaluate risks and benefits associated with a decision, as perceived risk. Individuals perceive risk in contemplating a particular decision or behaviour, but such a decision may involve choices with inherent uncertainty. Risk is also defined as a lack of knowledge about the distribution of potential outcomes (March, 1978), suggesting a link between knowledge gap and perceived risk. The perceived uncertainty motivates individuals to obtain information to be able to predict and evaluate the negative and positive consequences (losses and gains) of a decision. Humans normally use a value judgement to weigh up the consequences of risky prospects. The more value people attribute to an activity (due to a favourable affective impression), the lower risk they perceive (Alhakami and Slovic, 1994; Finucane, Alhakami, Slovic and Johnson, 2000). Kahneman and Tversky’s (2013) influential prospect theory suggests that in situations with high prospects of gains, individuals are more likely to protect the gains, becoming rigid and risk-averse; while in situations with high prospects of loss, risk-taking behaviour becomes more common. Some studies (e.g., Osborn and Jackson, 1988; Thaler and Johnson, 1990) have contradicted prospect theory, arguing that with a high probability of losing, individuals are more likely to become risk-averse, and conversely, a high probability of gains may encourage people to take risks. The contradictory findings imply that situational factors have an effect on the relationship between risk perception and risk behaviour. Hoskisson, Chirico, Zyung and Gambeta (2017) recognise the existence of multiple competing theories that attempt to explain risk-taking behaviour, and provide a framework that lists a variety of situational factors that moderate managerial risk-taking. In this study, knowledge gap plays the role of the situational factor that influences the relationship between risk perception and information seeking, seen here as the individual’s immediate reaction to risk.
Aim of the study
As much as uncertainty arising from a knowledge gap has been critical to information science research, perceived risk can play a role in explaining certain phenomena within the information behaviour context. While the information science literature emphasises the role of knowledge gap as a motivating factor for information seeking, little research has examined the role of perceived risk as a natural motivational driver in influencing information seeking. Additionally, the interaction between knowledge gap and perceived risk as motivators for information seeking, is an under studied phenomenon in the information science research. The objective of the present study is to investigate one of the most basic questions in information behaviour studies, that is, what motivates people to seek information when risk is involved. Risk will be delimited to denote an everyday life risk. This is different from the risk associated with using an information system, such as the risk of facing difficulties using the system, or an information resource, such as the risk of being exposed to unwanted information (Savolainen, 2016), or poor information quality (Wittek, Liu, Daranyi, Gedeon and Lim, 2016). These risks have been studied in the information science literature as affective barriers to information seeking. In contrast, everyday life risk, as examined in this study, is assumed to be a driver for information seeking, rather than a barrier, as information seeking is used as a coping reaction. Figure 1 adopts the elements of the universe of knowledge in Wilson’s (2006) context of information seeking to clarify the scope of risk in the present study as one that focuses on the domain of everyday life risk.
In search for some clarification in what motivates information seeking, the aim of this study is to investigate how perceived risk and knowledge gap interact with each other to influence humans’ everyday information seeking. More specifically, the current study attempts to examine and model the interaction between various constructs in motivating information behaviour. Therefore, the overarching research question guiding this study is:
RQ: How do knowledge gap and perceived risk interact as antecedents of information seeking in risk situations?
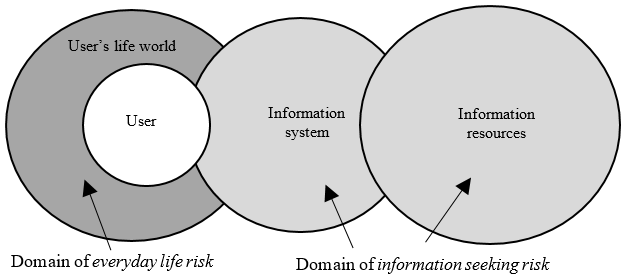
Figure 1: Domains of risk in information seeking
Literature review and hypotheses development
Knowledge gap and information seeking
An original version of knowledge gap emerged in the communication literature as the knowledge gap hypothesis, proposed by Tichenor, Donohue and Olien (1970). The hypothesis holds that knowledge grows at a faster rate among individuals of high socioeconomic status (SES) than among individuals of lower socioeconomic status, resulting in the appearance of a knowledge gap across the two groups. In this sense, a gap in knowledge is realised when ‘individuals encounter a discrepancy or lack of sense in their environment’ (Case, 2012, p. 113). As the notion of knowledge gap migrated to the information science literature, its locus moved from the societal to the individual level. As individuals regularly compare their current levels of knowledge to an ideal level they hope to achieve, they may realise a gap and react to the perceived uncertainty arising from that gap by seeking information. However, the recognition of a knowledge gap does not necessarily lead to actions. Actions occur when individuals consciously articulate their thoughts and identify potential solutions to their problems (Case, 2012, p. 80). At this point, knowledge gaps translate to information needs, as the primary cause of information seeking. Information needs are conscious realisations of recognised gaps in levels of knowledge. Atkin (1973, p. 206) defines an information need as:
a function of extrinsic uncertainty produced by a perceived discrepancy between the individual’s current level of certainty about critical environmental objects [people, things, events, ideas] and a criterion state that he seeks to achieve.
Moving to the information retrieval context, knowledge gap is the urge that drives a user to use an information system to address an information need. A knowledge gap originates from a ‘visceral need, […] a conscious or even unconscious need for information […] probably inexpressible in linguistic terms’ (Taylor, 2015, p. 254). Belkin (1980), employing Taylor’s concept of visceral need to propose his anomalous state of knowledge hypothesis, argues that, when individuals recognise anomalies (gaps) in their states of knowledge, they become motivated to consult information sources (Belkin, Oddy and Brooks, 1982). Anomalous state of knowledge can be an iterative process, in a sense that if the information seeker is not able to fill the perceived gap (i.e., satisfy the information need) completely, the gap may persist, or a new gap may emerge (Case, 2012, p. 83). Information seeking is not necessarily performed within information systems. Information can be also sought through interpersonal interactions (Berger, 2002; Berger and Calabrese, 1975; Bradac, 2001; Brashers, 2001; Liu, Bartz and Duke, 2016; Markon and Lemyre, 2013).
Even though the relationship between knowledge gap and information seeking has been generally established, a more detailed mechanism that links the two constructs has not been proposed. Lachlan, Spence and Nelson (2010) noted, information seeking is a cognitive reaction to perceived risk and fear caused by recognised knowledge gap and the resulting uncertainty. This study formalises the relationships between the three constructs, by considering knowledge gap, as defined earlier, as both a cause of perceived risk and a driver for information seeking. The decision sciences literature has considered uncertainty as a component of perceived risk (Hoskisson, Chirico, Zyung and Gambeta, 2017) and the information science literature has associated knowledge gap with feelings of uncertainty (e.g., Cole 2011; Kuhlthau 2005), but specific relationships have not been adequately examined. To address this gap, the present study introduces the following hypotheses.
H1: Knowledge gap has a positive effect on motivation to seek information.
H2: Knowledge gap has a positive effect on perceived risk.
Perceived risk and its interaction with risk propensity
In previous studies, perceived risk has been found to be related to risk propensity. Risk propensity is defined as ‘the tendency of a decision maker either to take or to avoid risks’ (Sitkin and Pablo, 1992, p. 12). Sitkin and Pablo (1992, p. 10) developed a model of determinants of risk behaviour, which simultaneously accounts for the effects of both dispositions and situations on risk propensity. This model conceptualises risk propensity as a confluence of personal dispositions, cognitive inputs, and past experiences. Based on this study, two major approaches to conceptualising risk propensity are identified in the literature. One approach is to view risk propensity as a domain-specific tendency, and another is to view it as a fixed personality trait. In the following paragraphs, the two approaches will be discussed in detail.
Prospect theory (Kahneman and Tversky, 2013), holds that risk taking is more common when individuals perceive high potential for loss, whereas, in situations where the prospect of gain is higher than loss, risk aversion is preferred. The theory explains that the semantic details (framing) of the situation can influence the evaluations of gains and losses, which are made with regards to a reference point. Tversky and Kahneman (1981) describe that when the outcomes of an action or behaviour are positively framed (phrased), subjects prefer to be risk averse, whereas when the consequences are negatively displayed, a tendency for risk taking is observed. Also, a study that investigates the effect of positive and negative framing on food behaviour suggests that framing can affect perceived risk, and, in turn, risk behaviour, and can have implications for communication at times of food crisis (Mitchell, Bakewell, Jackson and Heslin, 2015).
Other researchers (e.g., Fagley and Miller, 1997; Highhouse and Yüce, 1996; Hollenbeck, Ilgen, Phillips, and Hedlund, 1994) also suggest that risk seeking, in situations of perceived gain, and risk aversion, in situations of perceived loss, can occur. Weber and Milliman (1997) conducted an experimental study to explore the relationship between subjects’ risk propensity (risk seeking versus risk aversion) and perceived risk (more risky versus less risky). This study stresses the role of situational factors in determining how individuals evaluate gains and losses associated with a decision. The results of one experiment reveal that investors’ stock selection and the resulting perceived risk were different if they were presented with positive feedback about the outcomes of their decisions (e.g., they made money) than when negative feedback (e.g., they lost money) was provided (Weber and Milliman, 1997). The study proposes that risk propensity has a situational aspect, supported by fixed personality traits. Nicholson, Soane, Fenton O’Creevy and Willman, (2005) studied risk-seeking behaviour patterns of individuals across six domains. The study’s results demonstrate that some individuals can consistently take risks or be averse to risk, while others reveal domain-specific risk-taking behaviour patterns, suggesting risk seeking is influenced by several personal and situational factors. Similarly, Weber, Blais and Betz, (2002) investigated risk propensities across different domains (e.g., health, recreational, ethical, etc.) and found that risk seeking can be affected by domain-specific factors.
The concept of risk propensity as a personality trait has been investigated in the context of risk behaviour (e.g., Zuckerman, 1974; Zuckerman and Kuhlman, 2000; Breakwell, 2007, p. 44). People’s attitudes toward and perceptions of risk can be affected by their personality. The construct was proposed by Weber and Bottom (1989), who label this phenomenon as attitude toward perceived risk. Attitudes refer to general evaluations individuals make about other individuals, objects, issues, etc. (Petty, Cacioppo and Kasmer, 2015, p. 117). Nicholson, Soane, Fenton-O’Creevy and Willman (2005, p. 159) define attitude toward perceived risk as ‘the degree to which people find perceived risk attractive’. In a study developing a scale of attitude to risk, Weber, Blais and Betz (2002) emphasise the role of attitudes towards perceived risk in determining risk propensity. Similarly, Cooper, Woo and Dunkelberger (1988) argued that differences in attitudes towards perceived risk, which is rooted in differences in personalities, is the reason why entrepreneurs and managers show different risk-taking behaviours. They note that, compared to managers, entrepreneurs have a higher tendency towards risky behaviour.
Although risk propensity is a behaviour influenced by individuals’ personality traits and/or situational factors, this study only considers risk propensity as a general personality trait. This study supports the hypothesis that risk perception is influenced by individuals’ propensity for risk that are reflections of their fixed personality traits. Hence, this study hypothesises that:
H3: Risk propensity has a negative effect on perceived risk.
Perceived risk and information seeking
Researchers across many disciplines have studied risk, as judgments of risk have severe impacts on individuals’ success, survival and quality of life, as well as on environmental stability, the health of an entire society and the survival of the human species. Most cognitive psychology and neuroscience theories suggest that analytic and experiential systems can be used to analyse risk. These two distinct systems support cognitive functions that are dubbed in the cognitive and social psychology literature as the dual process, or type one-process and type two-process (Evans and Stanovich, 2013). The analytic system (type two) uses algorithms and normative rules for risk assessments. This system is relatively slow, effortful, and requires conscious control, whereas, the experiential system (type one) is intuitive, fast, mostly automatic, and not very accessible to conscious awareness (Slovic, Finucane, Peters and MacGregor, 2004).
In judging risk, individuals rely on their cognition as well as feelings to assess risks and benefits associated with events, decisions, behaviours, etc. (Kahneman, 2011, pp. 89-90; Sjӧberg, 2006; 2007). Perceived risk and perceived benefit are inversely correlated in individuals’ minds. This means higher perceived benefit results in lower perceived risk, and conversely, lower perceived benefit results in higher perceived risk (e.g., Finucane, Alhakami, Slovic and Johnson, 2000; Fischhoff, 1978; Slovic, Kraus, Lappe and Major, 1991; McDaniels, Axelrod, Cavanagh and Slovic, 1997). Alhakami and Slovic (1994) underscore the importance of emotional judgments in evaluating perceived risk and explain the relationship as follows: when individuals like an activity, they attribute more benefits and lower perceived risk to that activity, whereas when the activity is disliked, higher levels of perceived risk are associated with it. The reliance on emotional judgments increases with time constraints (De Dreu, 2003; De Dreu, Nijstad and Van Knippenberg, 2008).
Perceived risk is a consequence of risk judgments and a determinant of risk behaviour. Research into consumer behaviour shows that consumers use information seeking as a method to reduce their perceived risk and increase their confidence in their decisions (e.g., Locander and Hermann, 1979). Therefore, information seeking is motivated in the presence of high perceived risk. This relationship is depicted in the risk information seeking and processing model, proposed by Griffin, Dunwoody and Neuwirth (1999). This model considers the three constructs of information insufficiency, perceived information-gathering capacity (self-efficacy), and personal beliefs as the primary motivators of risk information seeking and processing. The model suggests that individuals’ risk perceptions (conceptualised as perceived hazard characteristics in risk information seeking and processing model) coupled with their affective responses will influence their perceptions of information insufficiency, and motivates information seeking and processing behaviour. In risk information seeking and processing model, information insufficiency is conceptualised according to Eagly and Chaiken’s (1993) notion of judgmental confidence, which refers to individuals’ confidence in their knowledge about a risk problem and their ability to handle the risk. The term characteristics in the construct perceived hazard characteristics implies multidimensionality of the risk. Multidimensionality of risk means that risk is beyond just the estimates of the likelihood of potential harms from exposure to risk (Griffin, Neuwirth, Dunwoody and Giese, 2004).
The risk perception attitude (RPA) framework suggests relationships between perceived risk, self-efficacy and information seeking intention (Rimal and Real, 2003). This framework is used for understanding and predicting the psychological underlying of humans’ self-protective behaviour. The framework suggests that perceived risk mediated by self-efficacy beliefs determine intention to seek information.
The planned risk information seeking model (Hovick, Kahlor and Liang, 2014; Kahlor, 2010) treats risk information seeking as a deliberate (planned) behaviour and maps the variables included in the theory of planned behaviour (TPB) and the risk information seeking and processing model to propose a new approach to modelling motivating factors of information seeking. In this model, risk perception is a motivator of information seeking, and its effect is mediated by affective risk response and perceived knowledge insufficiency. Therefore, similar to the risk information seeking and processing model, the planned risk information seeking model highlights the role of emotional responses, emerging from individuals' risk perception, in determining individual information seeking. Since all pairwise relationships among the aforementioned four constructs are positive, planned risk information seeking model suggests a positive relationship between risk perception and information seeking intention. The present study does not focus on affective risk response and proposes an alternative set of relationships, where the link between risk perception and information seeking intention is direct.
In view of the literature mentioned above, this study proposes two alternative relationships between perceived risk and motivation to seek information, one simpler and one more complex. These are formulated as follows:
H4: Perceived risk has a positive effect on motivation to seek information.
H5: Perceived risk has a modifying effect on the relationship between knowledge gap and motivation to seek information.
Proposed models
The current study examines the direct and indirect (interaction) effect of perceived risk on motivation to seek information. The constructs in this model are derived from the literature and include risk propensity, perceived risk, knowledge gap, and motivation to seek information. Table 1 provides sources and definitions of the constructs.
Construct | Definition | Source |
---|---|---|
Risk propensity | The tendency of a decision maker either to take or to avoid risks. This is typically considered as a personality trait that is relatively stable but can be influenced by environmental changes only slowly. | Sitkin and Pablo (1992); Zhao, Seibert and Hills (2005) |
Perceived risk | Intuitive judgements individuals make when they are asked to evaluate risks and benefits associated with a decision. | Slovic (1987); Kahneman (2011, p. 139) |
Knowledge gap | Realisation of a deficiency in an individual’s state of knowledge regarding a given situation or problem. | Belkin, Oddy, and Brooks (1982) |
Motivation to seek information | Individuals’ willingness to search and acquire information, in order to reduce a perceived knowledge gap associated with a certain event or decision. | Cox and Rich (1964) |
In order to hypothesise, test, and compare these effects, two models are proposed. These are shown as model 1 and model 2 in Figure 2. In model 1, perceived risk is directly connected to motivation to seek information, whereas, in model 2, perceived risk modifies the relationship between knowledge gap and motivation to seek information. Consequently, two groups of competing hypotheses are considered in the study (Table 2). Model 1 includes H1, H2, H3, and H4, where H4 assumes a direct connection between perceived risk and motivation to information seeking. In model 2, while the first three hypotheses remain the same, H4 is replaced with H5, which hypothesises an interaction construct (perceived risk × knowledge gap) as a predictor of intention to search for information. The interaction assumes that perceived risk modifies the relationship between knowledge gap and information seeking.
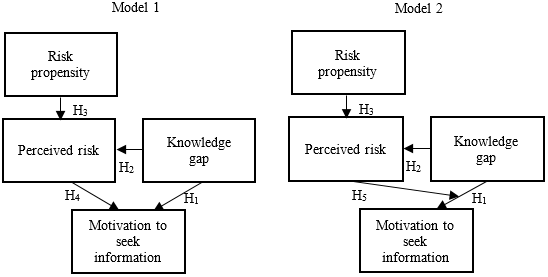
Figure 2: Two alternative models for motivation to seek information under risk
The relationship between perceived risk and motivation to seek information, hypothesised in H4, is well-supported in the literature: individuals seek information in order to cope with perceived risk. In situations of unusually high perceived risk, however, this relationship may be strengthened or weakened. In her theory of life in the round, Chatman (1999) proposes conditions for information seeking and information avoidance in a prisoner’s world of low precision and high uncertainty and offers conditions for crossing information-seeking boundaries. In other examples, cancer patients tend to avoid information about their conditions or different treatment choices when their health is at risk (Brashers, Neidig, Hass, Dobbs, Cardillo and Russell, 2000), and college teachers tend to avoid information from Internet sources such as Rate my professors when they are concerned about risk to their professional careers (Golman, Hagmann, and Loewenstein, 2017). In such high-risk situations, individuals may want to avoid information, since ‘acquiring information and resolving uncertainty would be akin to accepting the risk of possible disappointment’ (Golman, Hagmann and Loewenstein, 2017, p. 106). This argument implies that the direct effects hypothesised in H1 and H4 alone cannot fully explain the situation. One way to address this modelling issue would be to introduce an interaction effect. This is done by H5, which proposes perceived risk as a moderator of the relationship between knowledge gap and motivation to seek information. Since the introduction of H5 makes the model more complex, following a parsimony argument, the approach of proposing two competing models is considered: a simpler model, with the relationships in H1, H2, H3, and H4, and a more complex model, where H4 is replaced by H5. Testing two competing models is a common practice in psychology, sociology, education and business research (e.g., Vigoda‐Gadot, 2007; Vitaro, Tremblay, Kerr, Pagani and Bukowski, 1997).
Summary of hypotheses of the proposed models
Table 2 summarises the hypotheses that were used for examining the relationships between constructs in the two proposed models. Hypotheses were developed based on the following information science, communication and decision science literature.
Hypotheses | Model version | |
---|---|---|
H1 | Knowledge gap has a positive effect on motivation to seek information. | 1, 2 |
H2 | Knowledge gap has a positive effect on perceived risk. | 1, 2 |
H3 | Risk propensity has a negative effect on perceived risk. | 1, 2 |
H4 | Perceived risk has a positive effect on motivation to seek information. | 1 |
H5 | Perceived risk has a modifying effect on the relationship between knowledge gap and motivation to seek information. | 2 |
The H1 hypothesis is based on Belkin’s (1980) anomalous state of knowledge and holds that an anomaly in individuals’ states of knowledge, i.e., knowledge gap, has a motivating effect on their information-seeking behaviour. The H2 hypothesis is based on Slovic (1987) and Tversky and Kahneman’s (1974) studies on risk and describes how negative feelings, such as uncertainty arising from an identified gap in knowledge affect humans’ risk judgments. The H3 hypothesis is based on an established link between risk propensity as a personality trait and perceived risk (Carland, Hoy, Boulton and Carland, 1984; Zuckerman and Kuhlman, 2000). The H4 hypothesis is based on the risk perception attitude framework. The framework suggests that individuals with high levels of perceived risk tend to spend time on information seeking and knowledge acquisition (Rimal and Real, 2003). Previously, the effects of perceived risk and knowledge gap were assumed independently. However, to examine how the interplay between these two constructs influences information seeking, the H5 hypothesis theorises that perceived risk and knowledge gap motivate information seeking when interacting with each other.
Research methods
Since this research focuses on behavioural aspects of information seeking, it employs a scenario-based survey to establish relationships among psychological constructs that motivate individuals to seek information. The survey approach is commonly used in social sciences research for the purpose of obtaining empirical support for a theoretical model. Surveys ‘rely on the technique of self-report to obtain information about such variables as people’s attitudes, opinions, behaviours, and demographic characteristics’ (Kirk, 2013, p. 7). In her review of published information behaviour research, Greifeneder (2014) found thirty-four studies where the survey method was used. For example, Smith (2016) used the survey method to test relationships among privacy and trust attitudes, and subjects’ intent to volunteer for research studies that require installation of tracking software. Risk propensity, perceived risk, perceived knowledge gap and motivation to seek information, are all hypothetical constructs used in this study. These were manipulated by using hypothetical scenarios, and subjects were randomly assigned to one of the designed scenarios. However, the main purpose of the scenarios was not to provide experimental treatments that control the low and high levels of the constructs. Rather, their purpose was to increase variability in the constructs and provide a complete coverage of their measurement space.
Once the constructs were measured using the survey instrument, the hypotheses were tested using statistical tests within partial least squares structural equation modelling (PLS-SEM), also known as partial least squares path modelling (Fornell and Larcker, 1981). Partial least squares structural equation modelling is a commonly used method for theory development in exploratory research (Hair, Ringle and Sarstedt, 2011; Tenenhaus, 2008), and can produce reliable results with relatively small sample sizes (Hair, Hault, Ringle and Sarstedt, 2014, p. 15), increased model complexity, and constructs with fewer items, even just one or two items (Hair, Ringle and Sarstedt, 2011). Hair, Hault, Ringle and Sarstedt (2014, p. 14) argue that, in cases where theory is in the process of being developed, partial least squares structural equation modelling, which is a component-based approach to structural equation modelling, is preferred over the covariance-based structural equation modelling (CB-SEM). While partial least squares structural equation modelling is still met with some resistance from researchers who are simply more familiar with other methodological approaches, recent debates have concluded that partial least squares does offer advantages and should continue to be used as an important analysis tool in social science research (Henseler, et al., 2014). In contrast to covariance-based structural equation modelling, partial least squares cannot automatically produce a goodness-of-fit (GOF) index, however, the model validity is calculated by determining structural paths and R-square values. The partial least squares method is increasingly applied to research in marketing and other business disciplines (Henseler, Ringle and Sinkovics 2009). Recently, partial least squares is receiving more attention in information science publications (e.g., Akter, D’Ambra and Ray, 2011; Apostolou, Bélanger, and Schaupp, 2017; D’Ambra, Wilson and Akter, 2013).
The partial least squares analyses to obtain the values for the measurement and structural models were performed in R, an open source software environment for statistical analysis and graphical representation. Partial least squares analyses generate two types of models: the measurement model and the structural model (Anderson and Gerbing, 1988). The measurement model consists of measures of the relationships between constructs and their indicators and is used for assessing the psychometric properties of the scales. The structural model is used for interpreting the structural relation results.
Scenario design
As part of the research design, different hypothetical scenarios were composed to function as manipulations of important constructs to capture variation in the response variable. The study used hypothetical scenarios to introduce variability in the predictor variables. Rather than measuring variability in the study constructs that is due to the subjects’ individual differences alone, the study design also introduced variability from the experimental treatments, i.e., the scenarios. The purpose was to study individuals’ information seeking intention, prompted not just by their personal characteristics, such as their interests, past experiences, or mood at the moment when the study was conducted. Rather, the purpose was to capture differences in the subjects’ perceived risk and knowledge gap associated with a situation, or a context, represented in the study by the scenarios. This scenario-based approach, also known as scenario-based role-playing experiment, is commonly used in the social sciences literature (Rungtusanatham, Wallin, and Eckerd, 2011).
The study was designed to address a number of validity threats. It was conducted in a short period, to minimise history and maturation effects. Each participant was recorded only once, to minimise testing and regression effects. Participants were randomly assigned to one scenario, to reduce the risk of self-selection threat. Experimenter and participant effects were not reported (Gay, Mills, and Airasian, 2009, pp. 243-244).
An example of the scenarios is presented in Appendix A. These scenarios were designed so that they represent different levels of the two constructs, knowledge gap and perceived risk, within their contexts. To represent the different levels (low, high) of the two constructs, two different scenario groups were designed for each of the four construct level combinations, for a total of eight scenarios. All scenarios involve information seeking prior to making a travel decision. In the first scenario group, the decision-making context corresponds to a job interview. In the second scenario group, the decision-making context refers to visiting a friend. In both scenario groups, risk is represented by a disease outbreak. The scenario design components representing each level are shown in Table 3 and the corresponding scenarios are presented in Table 4. The inclusion of these components made the scenarios consistent and comparable. For example, all the scenarios designed to signify high risk include the phrase, ‘the disease is severe, spreads fast, and there is no vaccination plan’.
Level | Perceived risk | Knowledge gap |
---|---|---|
Low |
|
|
High |
|
|
Scenario | Knowledge gap | Perceived risk | |
---|---|---|---|
Decision-making context 1 (a job interview) | A | H | H |
B | H | L | |
C | L | H | |
D | L | L | |
Decision-making context 2 (visiting a friend) | E | H | H |
F | H | L | |
G | L | H | |
H | L | L |
Measurement of constructs
Items for each construct were developed to operationalise and measure the hypothetical constructs empirically and quantitatively. Except for the risk propensity construct, which is a formative construct measured by fifteen items, four items were developed for each one of the remaining constructs, including perceived knowledge gap, perceived risk, and motivation to seek information based on extensive review of the literature. Appendix B lists the survey items used to measure the three constructs. The items refer to the disease outbreak situation described in the scenarios. Survey responses were captured using a four-point Likert scale, ranging from strongly disagree to strongly agree. The fifteen items for measuring the risk propensity construct were a subset of the domain-specific risk taking (DOSPERT) questionnaire’s items (Blais and Weber, 2006). The survey responses associated with this construct were captured using a four-point Likert scale, ranging from not at all risky to extremely risky. In addition, an introduction section that presents one of the eight designed scenarios, and a section with demographic questions were incorporated into the online questionnaire created using the Qualtrics survey tool. The demographics included respondents’ travel habits (frequency), sex and age. Instrument development started with a pilot study. Data and analysis results from the pilot study were used to assess internal consistency and convergent and discriminant validity of the constructs and perform manipulation checks to validate and finalise the instrument. Since the study uses eight different scenarios, eight corresponding versions of the survey instrument were prepared. The Qualtrics randomiser feature was used to assign one of the eight scenarios to each respondent.
Data collection and respondents’ demographics
The general adult population of information seekers, eighteen years or older, with relatively advanced levels of information literacy and access to technology, was targeted. Subjects for this study were recruited based on their enrolment in undergraduate- or graduate-level courses at a large public university in the United States. Out of 700 invited students, 438 consented to participate. Responses with duration less than five minutes and more than one day were considered to be low quality data and were excluded from the analysis. The final data set included 289 responses. The final data set was checked against the original set of 438 responses, to verify that the distributions of scenarios, gender, age groups and traveling experience levels were comparable and the selection of the final data set did not bias the sample.
Table 5 summarises the descriptive statistics of the demographic variables, travel frequency, sex, and age. Regarding travel frequency, about half of the respondents (145) picked I travel with moderate frequency, 107 (37.02%) selected I travel very infrequently, and 30 (10.38%) and 7 (2.42%) identified themselves as I am a very frequent traveler and I never travel respectively. Of the sample of 289 respondents, there were 160 male participants (55.36%) and 129 female participants (44.46%). In terms of age, the survey participants are distributed in the following age group: 18–20 (n=87; 30.10%), 21–25 (n=125; 43.25%), 26–35 (n=52; 17.99%), 36–50 (n=23; 7.96%), and 50+ (n=2; 0.69%).
Characteristic | Categories | Frequency | % |
---|---|---|---|
Gender | Male | 160 | 55.36 |
Female | 129 | 44.64 | |
Age | 18-20 | 87 | 30.10 |
21-25 | 125 | 43.25 | |
26-35 | 52 | 17.99 | |
36-50 | 23 | 7.96 | |
50+ | 2 | 0.69 | |
Travel Frequency | I am a frequent traveller | 7 | 2.42 |
I travel with moderate frequency | 107 | 37.02 | |
I travel infrequently | 145 | 50.17 | |
I never travel | 30 | 10.38 |
Measurement model
The overall measurement model was assessed in terms of reliability, convergent validity, and discriminant validity. To evaluate the interaction construct, sixteen items were generated, as knowledge gap and perceived risk, each consisted of four analytical items (four multiplied by four).
Cronbach’s alpha was used to estimate the internal consistency of a set of items designed to measure the construct. The results reveal that the Cronbach’s alpha scores for constructs in model 1 (main effect model) and model 2 (interaction model) were all greater than 0.7, which is typically an acceptable threshold (Nunnally and Bernstein, 1994), suggesting high construct internal consistency. Next, the constructs’ convergent and discriminant validity were assessed using exploratory factor analysis with varimax rotation. The analysis of all items from the reflective constructs, i.e., knowledge gap (KGap), perceived risk (PerRisk), and motivation to seek information (InfoSeek), yielded three factors, as expected. Item cross-loadings were larger than 0.6 on one latent factor and smaller on all the other latent factors (see Appendix C). As recommended by Falk and Miller (1992), loadings above 0.55 are acceptable. Chin (1998, p. 325) notes, ‘loadings of 0.5 and 0.6 may still be acceptable if there exist other indicators in the block for comparison’. Therefore, the results indicate acceptable discriminant and convergent validity of the constructs. The convergent validity of the constructs was also assessed using the average variance extracted (AVE). Results presented in Table 6 show that all average variance extracted values are above the suggested threshold of 0.5, indicating that the measurement model has good convergent validity (Chin, 1998). Similarly, the discriminant validity of the instrument was also confirmed by looking at the square roots of average variance extracted as recommended by Fornell and Larcker (1981). Table 7 verifies that the square roots of the average variance extracted for each construct are greater than its highest correlation with any other construct. Overall, the results of the measurement models were considered satisfactory and the two models examined in this study were employed for testing the research hypotheses.
Measure | Items | AVE | Cronbach’s α |
---|---|---|---|
Model 1 | |||
Knowledge gap (KGap) | 4 | 0.526 | 0.750 |
Perceived risk (PerRisk) | 4 | 0.832 | 0.933 |
Motivation to seek information (InfoSeek) | 4 | 0.780 | 0.905 |
Model 2 | |||
Knowledge gap (KGap) | 4 | 0.526 | 0.750 |
Knowledge gap × perceived risk (RiskGap) | 16 | 0.703 | 0.972 |
Perceived risk (PerRisk) | 4 | 0.832 | 0.933 |
Motivation to seek information (InfoSeek) | 4 | 0.780 | 0.905 |
RiskProp | KGap | RiskGap | PerRisk | InfoSeek | |
---|---|---|---|---|---|
Model 1 | |||||
RiskProp | 1.000 | ||||
KGap | −0.103 | 1.000 | |||
PerRisk | −0.280 | 0.268 | 1.000 | ||
InfoSeek | −0.176 | 0.370 | 0.302 | 1.000 | |
Model 2 | |||||
RiskProp | 1.000 | ||||
KGap | −0.103 | 1.000 | |||
RiskGap | −0.245 | 0.762 | 1.000 | ||
PerRisk | −0.280 | 0.268 | 0.788 | 1.000 | |
InfoSeek | −0.176 | 0.370 | 0.412 | 0.302 | 1.000 |
Since the research design relied on a single measurement method (mono-method research design), a potential concern would be the presence of common method bias (CMB). In the presence of common method bias, the measured variance in constructs may be attributable to the measurement method rather than to the construct of interest itself. To test for common method bias, one approach is to perform the Harman’s test for the presence of one factor (Podsakoff, MacKenzie, Lee and Podsakoff, 2003). Factor analysis results indicate that 18.347% of total variance in the 27 items (4 for PerRisk, 4 for KGap, 4 for InfoSeek and 15 for RiskProp) is explained by that one factor. This is less than 50%, therefore common method bias is not a problem.
Structural models: hypotheses tests
With an adequate measurement model and acceptable level of multicollinearity, the complex cause-effect relationships between the latent variables in the proposed models were tested with partial least squares structural equation modelling. In structural equation models, the model’s quality and significance are determined using the path coefficient values. These coefficients are interpreted as standardised regression coefficients (Fornell and Larcker, 1981). The structure of the models and constructs involved in this study are presented in Figure 3 and Table 8.
Results for model 1 indicate a significant path coefficient between knowledge gap and motivation to seek information (b = 0.3, t = 5.6, p < 0.001), validating H1. The positive relationship between knowledge gap and perceived risk was also found to be significant (b = 0.242, t = 4.38, p < 0.001), supporting H2. The negative effect of risk propensity on perceived risk is significant (b = −0.255, t = −4.62, p < 0.001), supporting H3. The path coefficient between perceived risk and motivation to seek information is significant (b = 0.219, t = 3.94, p < 0.001), supporting H4. Variance explained (R2) is 13.6% for perceived risk and 18.1% for motivation to seek information.
Regarding the power of the statistical tests performed in the two models, following Hair, Hault, Ringle and Sarstedt (2014, p. 22), since the maximum number of independent variables pointing at any construct in the models is two, a minimum of 158 observations would be needed to achieve the commonly used statistical power level of 80% and to detect R2 values of at least 10% with a 1% probability of type-I error. Since the sample size of the study is 289, the models have sufficient statistical power that exceeds 80%.
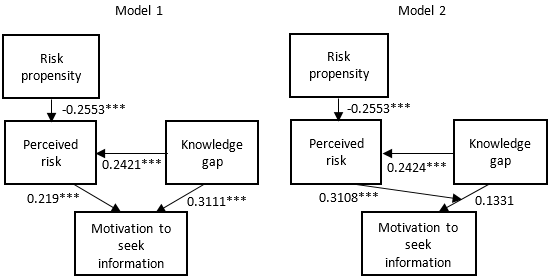
Figure 3: Results of structural model analysis for the two models
Path | Path coeff. | t-value | p-value | Result | |
---|---|---|---|---|---|
Model 1 | |||||
H1 | KGap → InfoSeek | 0.311 | 5.600 | *** | Supported |
H2 | KGap → PerRisk | 0.242 | 4.380 | *** | Supported |
H3 | RiskProp → PerRisk | −0.255 | −4.620 | *** | Supported |
H4 | PerRisk → InfoSeek | 0.219 | 3.940 | *** | Supported |
Model 2 | |||||
H1 | KGap → InfoSeek | 0.133 | 1.610 | 0.109 | Not supported |
H2 | KGap → PerRisk | 0.242 | 4.390 | *** | Supported |
H3 | RiskProp → PerRisk | −0.255 | −4.620 | *** | Supported |
H5 | RiskGap → InfoSeek | 0.311 | 3.750 | *** | Supported |
Bootstrap resampling was used to validate the structural models (Chin, 2010, p. 83). 1,000 bootstrap iterations were used. All loadings were again above 0.6 as recommended by Chin (1998). However, the confidence intervals for the path coefficient between risk propensity and perceived risk in both models includes zero, indicating a lack of consistency in the significance of this coefficient among the 1,000 bootstrapped samples.
Goodness-of-fit measures
In partial least squares path modelling, the quality of causal models can be determined by a goodness-of-fit index for endogenous constructs (Tenenhaus, Esposito, Vinzi, Chatelin, and Lauro, 2005). The computed goodness-of-fit scores for model 1 (main effect) and model 2 (interactive) are 0.2415 and 0.278, respectively. Following Wetzels, Odekerken-Schröder and van Oppen’s (2009) guidelines, the goodness-of-fit score for the main effect model (model 1) exceeds the baseline value of goodness-of-fit(small) = 0.1 but does not exceed the goodness-of-fit(medium) = 0.25 threshold. This indicates that the main effect model marginally satisfies the criterion for medium fit. However, the goodness-of-fit score for the interaction model (model 2) exceeds the baseline value of goodness-of-fit(medium) = 0.25, indicating that the second model satisfies the criterion for medium fit and has better explaining power than the first model.
Discussion
The objective of this study was to explain what motivates people to seek information when risk is involved. This would add to the collective understanding of motivational drivers that underlie individuals’ information-seeking behaviour when they encounter risk. Accordingly, three potentially salient motivational factors (risk propensity, perceived risk, knowledge gap) associated with the risk behaviour model (Sitkin and Pablo, 1992), anomalous state of knowledge hypothesis (Belkin, 1980), the risk perception attitude framework (Rimal and Real, 2003), and the theories of uncertainty management (Brashers, Neidig, Hass, Dobbs, Cardillo and Russell, 2000) and uncertainty reduction (Berger and Calabrese, 1975) were applied as antecedents to motivation to seek information. Two competing theoretical models were designed to account for the direct and moderating effect of perceived risk. Data were collected through a scenario-based survey and partial least squares structural equation modelling analysis was conducted. All four relationships posited in model 1 and three out of four in model 2 were supported. The primary difference between the two models is the relationship of perceived risk to motivation to seek information. In model 1, perceived risk is directly related to motivation, whereas in model 2, perceived risk moderates the relationship between knowledge gap and motivation to seek information. The bootstrap validation resulted in partially consistent findings. This study’s findings contribute to the development of theory in information behaviour. In particular, the study provides the following significant insights.
The first finding is that the relationship between risk propensity and perceived risk is significant, supporting H3, when the entire sample of 289 participants is used. This is consistent with Sitkin and Pablo (1992) or Sitkin and Weingart (1995), where a general tendency of a decision-maker to take or avoid risk was reported to have an important effect on the decision-maker’s risk perception. However, the results obtained from the bootstrap resampling, where much smaller sample sizes are used, do not support this relationship. One explanation for this finding is possible bias of the samples with respect to subject attributes such as gender, age, or personality type. To account for possible effects of gender, this attribute was added to the model, but the relationship between the two constructs remained insignificant. Another explanation is that the relationship between the constructs is relatively weak, producing statistically insignificant results when the smaller bootstrap samples are used. It is important to note that the present study considers risk propensity as a general personality trait. Subject personalities are rather complex, and the specific measuring instrument used in the survey accounts only for the social dimension of risk propensity. Therefore, the resulting data may have produced an incomplete picture of the relationship between risk propensity and perceived risk. Nevertheless, an implication of this observation for researchers is that the relationship between these two constructs is rather complex and needs to be examined carefully by considering personality and contextual factors such as age, culture, etc. With respect to the present study, however, it is important to note that the nature of the relationship between these two constructs is not a direct focus. Risk propensity is used in the two models presented here as a control construct, so that the relationships among the other constructs, addressed in hypotheses H1, H2, H4, and H5, are correctly assessed, after accounting for any direct or indirect effects of risk propensity on those relationships. This is consistent with the approach taken by Nuijten, Keil, van der Pijl and Commandeur (2018), where risk propensity is also used as a control construct, and the relationship between risk propensity and perceived risk is reported as marginally significant.
The second finding is that individuals’ lack of understanding of the disease outbreak situation, or knowledge gap, influences their perception of risk, which, in turn, motivates engagement in effortful analysis of the attributes of the situation by seeking relevant information. The obtained information is used for the evaluation of potential consequences involved in a behaviour or a decision. This finding agrees with Sitkin and Pablo’s (1992) proposition that decision-makers with high levels of domain knowledge will make more accurate estimates of risk and have more confidence in making those estimates. As the assessment of risk is a highly analytical cognitive process, the relationship between knowledge gap and perceived risk implies a more deliberate, data-driven decision-making process. This finding is supported by the dual-process theories. For example, the motivation and opportunity as determinants of attitude-behaviour processes (MODE) model proposed by Fazio (1990) posits that individuals invoke the analytical system, and rely on data-driven, attribute-based processing strategies when they are motivated to form correct risk judgments and make good decisions. We believe that a higher level of knowledge gap provides such a motivation. On the other hand, when people engage in simpler activities that require less cognitive effort, such as driving a car on an empty road or completing the phrase ‘bread and…,’ they choose spontaneous processing strategies by making judgments based on their emotions and memories (Kahneman, 2011, p. 21). In such situations, people are less likely to form incorrect risk judgments and make poor decisions by relying on their emotions. Overall, the second finding of the present study suggests that the relationship between knowledge gap and motivation to seek information is not a simple one. Both models considered in the study include a direct, as well as an indirect path from knowledge gap to information seeking. Therefore, in the presence of risk, the information seeking mechanism is somewhat complex, including direct and indirect relationships. An ongoing follow-up analysis shows that knowledge gap remains a strong determinant of perceived risk among male participants but becomes insignificant among female participants. This is another example of the complex way in which perceived risk is involved in information-seeking behaviour.
The third finding is that the interaction effect of perceived risk and knowledge gap on information seeking is stronger than the direct effect of perceived risk on information seeking. This is interpreted as follows. When the two constructs are independent antecedents to information-seeking motivation, a low perceived risk level could still be associated with high motivation for information seeking, if knowledge gap is moderately high (model 1). However, in model 2, due to the interaction effect, when perceived risk is very low, the individual may decide not to seek information, regardless of the knowledge gap level. Therefore, the presence of perceived risk as a moderator emphasises its role in information-seeking behaviour. For reference purposes, the interaction effect proposed in model 2 shall be called the risk-gap interaction hypothesis (RGIH).
The fourth finding addresses the relationship between knowledge gap and information seeking. The relationship is well-established in the information science literature. According to Belkin’s (1980) anomalous state of knowledge and Dervin’s (1999) sense making theory, when users perceive inadequacies in their states of knowledge, they get motivated to search for information to address the inadequacy and be able to make decisions. The present study supports this relationship when knowledge gap and perceived risk are hypothesised to be directly connected to motivation to seek information, as in model 1. However, it is worth noting that more recent studies (Kahlor, 2010; Yang and Kahlor, 2013) found no support for the link between knowledge gap and information seeking intent. The lack of support for this direct relationship is replicated in the present study in model 2, where the interaction between perceived risk and knowledge gap is present, as suggested by the risk-gap interaction hypothesis, and the direct effect between knowledge gap and motivation to seek information becomes insignificant. This points to a moderating effect of perceived risk, which is not well-studied in the information science literature. Although the relationship between knowledge gap and information seeking has been proposed, few studies have explored the role of knowledge gap in motivating information seeking quantitatively. Therefore, although it seems rational to assume that a perceived gap in one’s knowledge would lead to information seeking, seeking behaviours have been found to be complex and driven by many factors (Dunwoody and Griffin, 2015, p. 114; Robson and Robinson, 2013). The present study introduces an interaction effect (risk-gap interaction hypothesis) as a possible explanation of the inconsistencies found in the literature. In any case, this phenomenon needs to be further investigated in future studies.
The analysis presented in this article advances an understanding of information seeking under risk by pointing to the potential importance of perceived risk, and answers the research question, ‘how do knowledge gap and perceived risk interact as antecedents of information seeking in risk situations?’ The study found that both knowledge gap and perceived risk play a significant role in motivating information-seeking behaviour. However, the interaction between the two constructs has a stronger effect on motivation to seek information than perceived risk itself. The findings also suggest that when the interaction term is present, knowledge gap is only a driver of perceived risk, but not information seeking. However, the findings must be interpreted in the light of the study’s limitations. First, as the data are cross-sectional and not longitudinal, the posited causal relationships, although firmly based on generally accepted theories, could only be inferred rather than proven. A future longitudinal study could measure the constructs at two different points in time, in order to establish causality between the constructs. Second, although the study sample included international students from different cultures, the majority of respondents were from the United States, making the study vulnerable to single source bias. Future studies could explore the relationships described in the models in different cultural environments. Since the study focuses on the three constructs of perceived risk, knowledge gap and motivation to seek information, other significant determinants, such as personal relevance (Petty and Wegener, 1999; Petty and Cacioppo, 1990), fear (Finucane, Alhakami, Slovic and Johnson, 2000; Slovic, Finucane, Peters and MacGregor, 2007), curiosity (Wilson, 1997), and time-pressure were not examined by design. Given these limitations, future validations of the model through more rigorous research designs and across different contexts, are suggested. Finally, future studies could also extend the research models to include consequences of motivation to seek information, such as individuals’ actual information seeking.
Conclusions
By surfacing motivational drivers associated with individuals' information-seeking behaviour and providing empirical evidence regarding the efficacy of these motivational drivers, this study has contributed to the development of a richer understanding of the processes underlying information seeking in the presence of risk. The study has identified risk propensity, perceived risk, and knowledge gap, as key factors that explain individuals’ intention to seek information when risk is involved. The main contribution of the study is the proposal of the risk-gap interaction hypothesis (RGIH), which suggests that risk perceptions interact with knowledge gap to motivate information seeking. Since the interaction between perceived risk and knowledge gap, and its effect on motivating information seeking, have not been previously investigated, the findings of this study contribute to the advancement of the information behaviour literature. The conclusion from the comparison of the two competing models is that perceived risk is a primary predictor of information seeking in risk situations. The motivation for engaging in a particular information behaviour is driven by risk perceptions. Such perceptions are influenced by gaps in the existing knowledge structure and reflect subjective assessments of risk. This study also highlights the need for deliberately reflecting upon behavioural attitudes. This implies the significance of having or acquiring domain knowledge to make accurate estimations of the likely consequences of the decisions.
About the authors
Shadi Shakeri is a PhD candidate at the University of North Texas College of Information, Department of Information Science. Her research interests include risk information behavior, text analytics and decision making, and data modeling. She can be contacted at shadishakeri@my.unt.edu
Nicholas Evangelopoulos is a Professor at the University of North Texas College of Business, Department of Information Technology and Decision Sciences. His research and teaching focus on business analytics, text analytics and decision making. He can be contacted at Nick.Evangelopoulos@unt.edu
Oksana L. Zavalina is an Associate Professor at the University of North Texas College of Information Department of Information Science. Her research and teaching focus on information organization and information seeking behavior. She can be contacted at Oksana.Zavalina@unt.edu
References
- Akter, S., D’Ambra, J. & Ray, P. (2011). Trustworthiness in health information services: an assessment of a hierarchical model with mediating and moderating effects using partial least squares (PLS). Journal of the American Society for Information Science and Technology, 62(1), 100–116.
- Alhakami, A. S. & Slovic, P. (1994). A psychological study of the inverse relationship between perceived risk and perceived benefit. Risk Analysis, 14(6), 1085–1096.
- Anderson, J. C. & Gerbing, D. W. (1988). Structural equation modeling in practice: a review and recommended two-step approach. Psychological Bulletin, 103(3), 411–423.
- Apostolou, B., Bélanger, F. & Schaupp, L. C. (2017). Online communities: satisfaction and continued use intention. Information Research, 22(4), paper 774. Retrieved from http://www.informationr.net/ir/22-4/paper774.html.(Archived by WebCite® at http://www.webcitation.org/724CouNoQ).
- Atkin, C. K. (1973). Instrumental utilities and information seeking. In P. Clarke (Ed.), New models for mass communication research (pp. 205–242). Beverly Hills, CA: Sage Publications.
- Bates, Marcia J. (2010). Information Behaviour. In M. J. Bates & M. N. Maack (Eds.), Encyclopedia of library and information sciences (pp. 2381–2391). New York, NY: CRC Press.
- Belkin, N.J. (1980). Anomalous states of knowledge as a basis for information retrieval. Canadian Journal of Information Science, 5(1), 133–143.
- Belkin, N. J., Oddy, R. N. & Brooks, H. M. (1982). Ask for information retrieval: part I. Background and theory. Journal of Documentation, 38(2), 61–71.
- Bentley, A., Earls, M. & O’Brien, M. J. (2011). I’ll have what she’s having: mapping social behavior. Cambridge, MA: The MIT Press.
- Berger, C. R. (2002). Strategic and nonstrategic information acquisition. Human Communication Research, 28(2), 287–297.
- Berger, C. R. & Calabrese, R. J. (1975). Some exploration in initial interaction and beyond: toward a developmental theory and interpersonal communication. Human Communication Research, 1(2), 99–112.
- Blais, A. R. & Weber, E. U. (2006). A domain-specific risk-taking (DOSPERT) scale for adult populations. Judgment and Decision Making, 1(1), 33–47.
- Bowler, L. (2011). Into the land of adolescent metacognitive knowledge during the information search process: a metacognitive ethnography. In A. Spink & J. Heinstrӧm (Eds.), New directions in information behaviour (pp. 93–125). Bingley, UK: Emerald Group Publishing Limited.
- Bradac, J. J. (2001), Theory Comparison: uncertainty reduction, problematic integration, uncertainty management, and other curious constructs. Journal of Communication, 51(3), 456–476.
- Brashers, D. E. (2001). Communication and uncertainty management. Journal of Communication, 51(3), 477–497.
- Brashers, D. E., Neidig, J. L., Hass, S. F., Dobbs, L. K., Cardillo, L. W. & Russell, J. A. (2000). Communication in the management of uncertainty: the case of persons living with HIV or AIDS. Communication Monographs, 67(1), 63–84.
- Breakwell, G. M. (2007). The psychology of risk. Cambridge: Cambridge University Press.
- Carland, J. W., Hoy, F., Boulton, W. & Carland, J. A. C. (1984). Differentiating entrepreneurs from small business owners: a conceptualization. Academy of Management Review, 9(2), 354–359.
- Case, D. O. (2012). Looking for information: a survey of research on information seeking, needs, and behaviour. Bingley, UK: Emerald Group Publishing Limited.
- Chatman, E. A. (1999). A theory of life in the round. Journal of the American Society for Information Science, 47(3), 193–206.
- Chin, W. W. (1998). The partial least squares approach to structural equation modelling. In G. A. Marcoulides (Ed.), Modern methods for business research (pp. 295–336). Mahwah, NJ: Lawrence Erlbaum.
- Chin, W. W. (2010). Bootstrap cross-validation indices for PLS path model assessment. In V. E Vinzi, W. W. Chin, J. Henseler, H. Wang (Eds.), Handbook of partiallLeastsSquares, Springer Handbooks of Computational Statistics (pp. 83–97). Berlin: Springer-Verlag Berlin Heidelberg.
- Cole, C. (2011). A theory of information need for information retrieval that connects information to knowledge. Journal of the American Society for Information Science and Technology, 62(7), 1216–1231.
- Cole, C. (2012). Information need: a theory connecting information search to knowledge formation. Medford, NJ: Information Today.
- Conan Doyle, A. (2009). The hound of the Baskervilles (reprint ed.). New York, NY: Barnes & Noble.
- Cooper, A. C., Woo, C. Y. & Dunkelberg, W. C. (1988). Entrepreneurs’ perceived chances for success. Journal of Business Venturing, 3(2), 97–108.
- Cox, D. F. & Rich, S. U. (1964). Perceived risk and consumer decision-making: the case of telephone shopping. Journal of Marketing Research, 1(4), 32–39.
- D’Ambra, J., Wilson, C. S. & Akter, S. (2013). Application of the task-technology fit model to structure and evaluate the adoption of e-books by academics. Journal of the American Society for Information Science and Technology, 64(1), 48–64.
- De Dreu, C. K. (2003). Time pressure and closing of the mind in negotiation. Organizational Behaviour and Human Decision Processes, 91(2), 280–295.
- De Dreu, C.K., Nijstad B.A. & van Knippenberg D. (2008). Motivated information processing in group judgment and decision making. Personality and Social Psychology Review, 12(1), 22-49.
- Denga, Z. & Liu, S. (2017). Understanding consumer health information-seeking behaviour from the perspective of the risk perception attitude framework and social support in mobile social media websites. International Journal of Medical Informatics, 105, 98–109.
- Dervin, B. (1999). On studying information seeking methodologically: the implications of connecting metatheory to method. Information Processing & Management, 35(9), 727–750.
- Dunwoody, S. & Griffin, R. (2015). Risk information seeking and processing model. In H. Cho, T. Reimer and K. A. McComas (Eds.), SAGE handbook of risk communication (pp. 102–119). Thousand Oaks, CA: Sage Publications.
- Eagly, A. H. & Chaiken, S. (1993). The psychology of attitudes. Belmont, CA: Wadsworth.
- Ellis, D. (1989). A behavioural approach to information retrieval design. Journal of Documentation, 45(3), 171–212.
- Evans, J. St. B. T. & Stanovich, K. E. (2013). Dual-process theories of higher cognition: advancing the debate. Perspectives on Psychological Science, 8(3), 223–241.
- Fagley, N. S. & Miller, P. M. (1997). The effects of decision framing on choice of risky vs. certain options. Organizational Behaviour and Human Decision Processes, 39(2), 264–77.
- Falk, R. F. & Miller, N. B. (1992). A primer for soft modeling. Akron, Ohio: University of Akron Press.
- Fazio, R. H. (1990). Multiple processes by which attitudes guide behaviour: the MODE model as an integrative framework. In M. P. Zanna (Ed.), Advances in experimental social psychology (pp. 75–109). New York, NY: Academic Press.
- Finucane, M. L., Alhakami, A., Slovic, P. & Johnson, S.M., (2000). The affect heuristic in judgments of risks and benefits. Journal of Behavioural Decision Making, 13(1), 1–17.
- Fischhoff, B., Slovic, P., Lichtenstein, S., Read, S. & Combs, B. (1978). How safe is safe enough? A psychometric study of attitudes toward technological risks and benefits. Policy Sciences, 9(2), 127–152.
- Fornell, C. & Larcker, D. F. (1981). Evaluating structural equation models with unobservable variables and measurement error. Journal of Marketing Research, 18(1), 39–50.
- Fornell, C. G. & Cha, J. (1994). Partial least squares. In R. P. Bagozzi (Ed.), Advanced methods of marketing research (pp. 52–78). Oxford: Blackwell.
- Gay, L. R., Mills, G. E. & Airasian, P. (2009). Educational Research: competencies for analysis and applications. Upper Saddle River, NJ: Pearson.
- Golman, R., Hagmann, D. & Loewenstein, G. (2017). Information avoidance. Journal of Economic Literature, 55(1), 96–135.
- González Teruel, A., González Alcaide, G., Barrios, M. & Abad-García, M.F. (2015). Mapping recent information behavior research: an analysis of coauthorship and co-citation networks. Scientometrics, 103(2), 687–705.
- Greifeneder, E. (2014). Trends in information behaviour research. Information Research, 19(4). Retrieved from http://www.informationr.net/ir/19-4/isic/isic13.html (Archived by WebCite® at http://www.webcitation.org/724CzRqGF).
- Griffin, R. J., Dunwoody, S. & Neuwirth, K. (1999). Proposed model of the relationship of risk information seeking and processing to the development of preventive behaviours. Environmental Research, 80(2), S230–S245.
- Griffin, R. J., Neuwirth, K., Dunwoody, S. & Giese, J. (2004). Information sufficiency and risk communication. Media Psychology, 6(1), 23–61.
- Hair, J. F., Hault, G. T. M., Ringle, C. M. & Sarstedt, M. (2014). A primer on partial least squares structural equation modeling (PLS-SEM). Thousand Oaks, CA: SAGE Publications.
- Hair, J. F., Ringle, C. M. & Sarstedt, M. (2011). PLS-SEM: indeed a silver bullet. Journal of Marketing Theory and Practice, 19(2), 139–151.
- Henseler, J., Ringle, C. M. & Sinkovics, R. R. (2009). The use of partial least squares path modeling in international marketing. In R. R. Sinkovics & P. N. Ghauri (Eds.), New Challenges to International Marketing (Advances in International Marketing, Volume 20) (pp. 277–319). Bingley, UK: Emerald Group Publishing Limited.
- Henseler, J. Dijkstra, T. K., Sarstedt, M., Ringle, C. M., Diamantopoulos, A., Straub, ... Calantone, R. J. (2014). Common beliefs and reality about PLS: comments on Rönkkö and Evermann (2013). Organizational Research Methods, 17(2), 182–209.
- Highhouse, S. & Yüce, P. (1996). Perspectives, perceptions and risk-taking behaviour. Organizational Behaviour and Human Decision Processes, 65(2), 159–167.
- Hollenbeck, J. R., Ilgen, D. R., Phillips, J. M. & Hedlund, J. (1994). Decision risk in dynamic two stage contexts: beyond the status quo. Journal of Applied Psychology, 79(4), 592–598.
- Hoskisson, R., Chirico, F., Zyung, J. D. & Gambeta, E. (2017). Managerial risk taking: a multitheoretical review and future research agenda. Journal of Management, 43(1), 137–169.
- Hovick, S. R., Kahlor, L. & Liang, M. C. (2014). Personal cancer knowledge and information seeking through PRISM: the planned risk information seeking model. Journal of Health Communication, 19(4), 511–527.
- Julien, H., Pecoskie, J. & Reed, K. (2011). Trends in information behavior research, 1999–2008: a content analysis. Library & Information Science Research, 33(1), 19–24.
- Kahlor, L. (2010). PRISM: a planned risk information seeking model. Health Communication, 25(4), 345–356.
- Kahneman, D. (2011). Thinking, fast and slow. New York, NY: Farrar, Straus and Giroux.
- Kahneman, D. & Tversky, A. (2013). Prospect theory: an analysis of decision under risk. In (L. C. MacLean &W. T. Ziemba (Eds.), Handbook of the fundamentals of financial decision making (Vol. 4, pp. 99–127). Hackensak, NJ: World Scientific.
- Kirk, R. E (2013). Experimental design: procedures for the behavioral sciences (4th ed.). Thousand Oaks, CA: Sage Publications.
- Kouw, M., van den Heuvel, C. & Scharnhorst, A. (2013). Exploring uncertainty in knowledge representations: classification, simulations, and models of the world. In P. Wouters, A. Beaulieu, A. Scharnhorst, & S. Wyatt (Eds.), Virtual knowledge: experimenting in the humanities and the social sciences (pp. 89–125). Cambridge, MA: The MIT Press.
- Krikelas, J. (1983). Information-seeking behavior: patterns and concepts. Drexel Library Quarterly, 19(2), 5–20.
- Kuhlthau, C.C. (1991). Inside the search process: information seeking from the user’s perspective. Journal of the American Society for Information Science, 42(5), 361–371.
- Kuhlthau, C.C. (1993). A principle of uncertainty for information seeking. Journal of Documentation, 49(4), 339–355.
- Kuhlthau, C.C. (2005). Kuhlthau’s information search process. In K. E. Fisher, S. Erdelez & E. F. McKechnie (Eds.), Theories of information behavior (pp. 230–234). Medford, NJ: Information Today.
- Libby, R. & Fishburn, P. C. (1977). Behavioral models of risk taking in business decision: a survey and evaluation. Journal of Accounting Research, 15(2), 272–292.
- Lachlan, K. A., Spence, P. R. & Nelson, L. N. (2010). Gender differences in negative psychological responses to crisis news: the case of the I-35W collapse. Communication Research Reports, 27(1), 38–48.
- Liu, B. F., Bartz, L. & Duke, N. (2016). Communicating crisis uncertainty: a review of the knowledge gaps. Public Relations Review, 42(3), 479–487.
- Locander, W. B. & Hermann, P. W. (1979). The effect of self-confidence and anxiety on information seeking in consumer risk reduction. Journal of Marketing Research, 16(2), 268-274.
- Markon, M-P. L. & Lemyre, L. (2013). Public reactions to risk messages communicating different sources of uncertainty: an experimental test. Human and Ecological Risk Assessment: An International Journal, 19(4), 1102–1126.
- March, J. G. (1978). Bounded rationality, ambiguity, and the engineering of choice. Bell Journal of Economics, 9(2), 587–608.
- McDaniels, T. L., Axelrod, L. J., Cavanagh, N. S. & Slovic, P. (1997). Perception of ecological risk to water environments. Risk Analysis, 17(3), 341–352.
- Marcoulides, G. A. & Saunders, C. (2006). A silver bullet? MIS Quarterly, 30(2), iii-ix.
- Milojevic, S., Sugimoto, C. R., Yan, E. & Ding, Y. (2011). The cognitive structure of library and information science: analysis of article title words. Journal of the American Society for Information Science and Technology, 62(10), 1933–1953.
- Mitchell, V-W., Bakewell, C., Jackson, P. R. & Heslin, C. (2015). How message framing affects consumer attitudes in food crises. British Food Journal, 117(8), pp. 2200–2211.
- Nicholson, N., Soane, E., Fenton-O’Creevy, M. & Willman, P. (2005). Personality and domain-specific risk taking. Journal of Risk Research, 8(2), 157–176.
- Niedzwiedzka, B. (2003). A proposed general model of information behaviour. Information Research, 9(1), 1–18.
- Nuijten, A., Keil, M., van der Pijl, G. & Commandeur, H. (2018). IT managers’ vs. IT auditors’ perceptions of risks: an actor–observer asymmetry perspective. Information & Management, 55(1), 80–93.
- Nunnally, J. C. & Bernstein, I. H. (1994). Psychometric Theory. New York, NY: McGraw-Hill.
- Osborn, R. N. & Jackson, D. H. (1988). Leaders, riverboat gamblers, or purposeful unintended consequences in the management of complex dangerous technologies. Academy of Management Journal, 31(4), 924–947.
- Peters, E. & Slovic, P. (2000). The springs of action: affective and analytical information processing in choice. Personality and Social Psychology Bulletin, 26(12), 1465–1475.
- Petty, R.E. & Cacioppo, J.T. (1990). Involvement and persuasion: tradition versus integration. Psychological Bulletin, 107(3), 367–374.
- Petty, R. E., Cacioppo, J. T. & Kasmer, J. A. (2015). The role of affect in the elaboration likelihood model of persuasion. In L. Donohew, H. E. Sypher & E. T. Higgins (Eds.), Communication, social cognition, and affect (p. 117). London: Psychology Press.
- Petty, R. E. & Wegener, D. T. (1999). The elaboration likelihood model: current status and controversies. In S. Chaiken & Y. Trope (Eds.), Dual process theories in social psychology (pp. 41–72). New York, NY: Guilford Press.
- Podsakoff, P. M., MacKenzie, S. B., Lee, J.-Y. & Podsakoff, N. P. (2003). Common method biases in behavioural research: a critical review of the literature and recommended remedies. Journal of Applied Psychology, 88(5), 879–903.
- Rimal, R. N. & Real, K. (2003). Perceived risk and efficacy beliefs as motivators of change: use of risk perception attitude (RPA) framework to understand health behaviours. Human Communication Research, 29(3), 370–399.
- Robson, A., & Robinson, L. (2013). Building on models of information behavior: linking information seeking and communication. Journal of Documentation, 69(2), 169–193.
- Rungtusanatham, M., Wallin, C. & Eckerd, S. (2011). The vignette in a scenario-based role-playing experiment. Journal of Supply Chain Management, 47(3), 9–16.
- Savolainen, R. (2016). Approaching the affective barriers to information seeking: the viewpoint of appraisal theory. In Proceedings of ISIC, the Information Behaviour Conference, Zadar, Croatia: Part 1. Information Research, 21(4), paper isic1603. Retrieved from http://InformationR.net/ir/21-4/isic/isic1603.html. (Archived by WebCite® at http://www.webcitation.org/6mHhZwHsd).
- Sitkin, S.B. & Pablo, A.L. (1992). Reconceptualizing the determinants of risk behavior. Academy of Management Review, 17(1), 9–38.
- Sitkin, S.B. & Weingart, L.R. (1995). Determinants of risky decision-making behaviour: a test of the mediating role of risk perceptions and propensity. Academy of Management Journal, 38(6), 1573–1592.
- Sjöberg, L. (2006). Nuclear waste risk perceptions and attitudes in siting a final repository for spent nuclear fuel. In K. Andersson (Ed.), VALDOR 2006 Proceedings-Values in decisions on risk. Stockholm: Congrex AB.
- Sjöberg, L. (2007). Emotions and risk perception. Risk Management, 9(4), 223–237.
- Slovic, P. (1987). Perception of risk. Science, 236(4799), 280–285.
- Slovic, P., Finucane, M. L., Peters, E. & MacGregor, D. G. (2004). Risk as analysis and risk as feelings: some thoughts about affect, reason, risk, and rationality. Risk Analysis, 24(2), 311–322.
- Slovic, P., Finucane, M. L., Peters, E. & MacGregor, D. G. (2007). The affect heuristic. European Journal of Operational Research, 177(3), 1333–1352.
- Slovic, P., Kraus, N., Lappe, H. & Major, M. (1991). Risk perception of prescription drugs: report on a survey in Canada. Canadian Journal of Public Health, 82(3), S15–S20.
- Smith, C. (2016). Privacy and trust attitudes in the intent to volunteer for data-tracking research. Information Research, 21(4), paper 726. Retrieved from http://www.informationr.net/ir/21-4/paper726.html. (Archived by WebCite® at http://www.webcitation.org/6m5H0ikHJ).
- Taylor, R. S. (2015). Question-negotiation and information seeking in libraries. College and Research Libraries, 76(3), 251–267.
- Tenenhaus, M. (2008). Component-based structural equation modelling. Total Quality Management & Business Excellence, 19(7-8), 871-886.
- Tenenhaus, M., Esposito Vinzi, V., Chatelin, Y.-M. & Lauro, C. (2005). PLS path modeling. Computational Statistics & Data Analysis, 48(1), 159–205.
- Thaler, R. H. & Johnson, E. J. (1990). Gambling with the house money and trying to break even: the effects of prior outcomes on risky choice. Management Science, 36(6), 643–660.
- Tichenor, P. J., Donohue, G. A. & Olien, C. N. (1970). Mass media flow and differential growth of knowledge. Public Opinion Quarterly, 34(2), 159–170.
- Tversky, A. & Kahneman, D. (1974). Judgment under uncertainty: heuristics and biases. Science, 185(4157), 1124–1131.
- Tversky, A. & Kahneman, D. (1981). The framing of decisions and the psychology of choice. Science, 211(4481), 453–458.
- Vakkari, P. (2008). Trends and approaches in information behaviour research. Information Research, 13(4), paper 361. Retrieved from http://www.informationr.net/ir/13-4/paper361.html. (Archived by WebCite® at http://www.webcitation.org/724Dn0Kjs).
- Vigoda‐Gadot, E. (2007). Leadership style, organizational politics, and employees' performance: an empirical examination of two competing models. Personnel Review, 36(5), 661–683.
- Vitaro, F., Tremblay, R. E., Kerr, M., Pagani, L. & Bukowski, W. M. (1997). Disruptiveness, friends' characteristics, and delinquency in early adolescence: a test of two competing models of development. Child Development, 68(4), 676–689.
- Weber, E. U., Blais, A. & Betz, N. E. (2002). A domain-specific risk-attitude scale: measuring risk perceptions and risk behaviours. Journal of Behavioural Decision Making, 15(4), 263–290.
- Weber, E. U. & Bottom, W. P. (1989). Axiomatic measures of perceived risk: some tests and extensions. Journal of Behavioral Decision Making, 2(2), 113–131.
- Weber, E. U. & Milliman, R. A. (1997). Perceived risk attitudes: relating risk perception to risky choice. Management Science, 43(2), 123–144.
- Wetzels, M., Odekerken-Schröder, G. & van Oppen, C. (2009). Using PLS path modeling for assessing hierarchical construct models: guidelines and empirical illustration. MIS Quarterly, 33(1), 177–195.
- Wilson, T. D. (1981). On user studies and information needs. Journal of Documentation, 37(1), 3–15.
- Wilson, T. D. (1997). Information behaviour: an interdisciplinary perspective. Information Processing & Management, 33(4), 551–572.
- Wilson, T.D. (1999). Models in information behaviour research. Journal of Documentation, 55(3), 249–133.
- Wilson, T. D. (2006). 60 years of the best in information research. On user studies and information needs. Journal of Documentation, 62(6), 658–670.
- Wittek, P., Liu, Y.-H., Darányi, S., Gedeon, T. & Lim, I. S. (2016). Risk and ambiguity in information seeking: eye gaze patterns reveal contextual behavior in dealing with uncertainty. Frontiers in Psychology, 7, paper 1790. Retrieved from https://www.frontiersin.org/articles/10.3389/fpsyg.2016.01790/full. (Archived by WebCite® at http://www.webcitation.org/729RNIENP).
- Yang, Z. J., Aloe, A. M. & Feeley, T. H. (2014). Risk information seeking and processing model: a meta-analysis. Journal of Communication, 64(1), 20–41.
- Yang, Z. J. & Kahlor, L. (2013). What, me worry? The role of affect in information seeking and avoidance. Science Communication, 35(2), 189–212.
- Zhao, H., Seibert, S. E. & Hills, G. E. (2005). The mediating role of self-efficacy in the development of entrepreneurial intentions. Journal of Applied Psychology, 90(6), 1265–1272.
- Zuckerman, M. (1974). The sensation-seeking motive. In B. Maher (Ed.), Progress in Experimental Personality Research (pp. 79–148). New York, NY: Academic Press.
- Zuckerman, M. & Kuhlman, D. M. (2000). Personality and risk-taking: common biosocial factors. Journal of Personality, 68(6), 999–1029.
Appendix A
Example scenario
[Scenario A: large gap, high risk, high relevance] After the preliminary screening search process, a company has invited you to visit their headquarters in New York City (NYC) for an interview with a hiring director. This job represents a big professional step up for you, and a huge raise over your current salary, even after you adjust it for the cost of living. Basically, it is your dream job. If you miss the interview, it may be hard to reschedule. The company will pay all expenses for your trip including flight ticket and hotel. The day before your flight, a breaking news story attracts your attention. They report that an infectious disease has started spreading on the East Coast. What exactly this disease is, and how serious it is, is a big mystery to you. The stories appear to be conflicting, depending on the source. You watched a video where a Center for Diseases Control and Prevention (CDC) official appeared concerned about the severity of the outbreak, how fast the disease appears to be spreading, and whether it is even possible to come up with any vaccination or another prevention plan. Other authorities seem uncertain about the status of the disease. A video shows the NYC mayor asking the public to avoid non-essential traveling to NYC until the situation clears up.
Appendix B
Survey items
[Knowledge Gap]
1.1. I feel I already have all the information I need
1.2. I think that I understand the situation pretty well
1.3. I do not have a complete understanding of this situation
1.4. I have so many questions about this situation!
[Perceived Risk]
2.1. I believe the overall risk of traveling to NYC right now is pretty high
2.2. The way I see it right now, traveling to NYC is a risky proposition
2.3. Right now, traveling to NYC exposes me to a great danger
2.4. If I travel to NYC, I feel that the risk of getting infected is high
[Motivation to Seek Information]
3.1. I really need to learn more about this issue
3.2. I am willing to search for additional information
3.3. I need to use various resources that would provide me with more information
3.4. I am motivated to search for additional information
Appendix C
Construct | Loadings | |||
---|---|---|---|---|
KGap | ||||
Gap1 | −0.0523 | 0.7105 | 0.1219 | 0.2182 |
Gap2 | −0.0807 | 0.6101 | 0.0492 | 0.1054 |
Gap3 | −0.0094 | 0.6787 | 0.0553 | 0.1913 |
Gap4 | −0.1178 | 0.8754 | 0.3390 | 0.3934 |
PerRisk | ||||
Risk1 | −0.2417 | 0.2437 | 0.9187 | 0.2830 |
Risk2 | −0.2927 | 0.3010 | 0.9326 | 0.3115 |
Risk3 | −0.2477 | 0.2036 | 0.9196 | 0.2544 |
Risk4 | −0.2317 | 0.2164 | 0.8777 | 0.2456 |
InfoSeek | ||||
Seek1 | −0.1347 | 0.3072 | 0.2842 | 0.8293 |
Seek2 | −0.1881 | 0.3351 | 0.2260 | 0.8992 |
Seek3 | −0.1589 | 0.3529 | 0.2842 | 0.8999 |
Seek4 | −0.1397 | 0.3083 | 0.2703 | 0.9012 |