Personalised Web search and user satisfaction: a user-centred evaluation
Sara Salehi, Jia Tina Du and Helen Ashman
Introduction. Most university students depend significantly, sometimes exclusively, on the Google search engine for their academic information needs. User satisfaction leads to users’ deeper engagement with an information system that is shown to improve learning in an educational setting. This paper evaluates students’ satisfaction with results from personalised Web search against non-personalised Web search.
Method. During semi-structured study sessions, twenty-eight participants (university students) were required to complete a series of search tasks using both personalised and non-personalised Web search.
Analysis. Evaluation was based on participants’ explicit feedback as well as their implicit behaviour including search time, number of queries and clicked result links per task, finding the answer and relevance of the search results.
Results. There was no apparent significant increase in the participants’ overall level of satisfaction with personalised search results compared to non-personalised results. However, it was found that personalised search reduced the time spent to finish a task and reduced the number of clicks required to arrive at the selected outcome.
Conclusions. Personalisation of search results does not increase students' satisfaction with their search results. However, it does reduce the time spent by students in locating information they judged to be satisfactory answers to their questions.
DOI: https://doi.org/10.47989/irpaper878
Introduction
One of the current challenges in education is finding and delivering relevant learning objects to students. Learning objects are online documents such as Web pages and articles that students use for immediate study or as an extra resource for a course or educational program (Biletskiy et al., 2012). Several studies and technologies have addressed this problem by personalising eLearning systems and improving the effectiveness of learning objects and their retrieval process. The objective of these studies was meeting students’ information needs and providing them with relevant and personalised learning material within their domain of understanding (Ananatharman, 2012; Biletskiy et al., 2009; O'Donoghue, 2010; Sathiyamurthy et al., 2012).
However, regardless of all the advances in eLearning systems, most university students depend on general-purpose search engines such as Google and Bing to find information and conduct research (Du and Evans, 2011; McClure and Clink, 2009; Purdy, 2012). University students predominantly, sometimes exclusively, use the Google general-purpose search engine for educational purposes. Since Google is personalising its search results for all users, even those without a Google account, it is in effect providing individual learning experiences for students, even though the personalisation is not necessarily intended to support educational achievement. This offers plenty of opportunities to improve higher education, and yet also raises some concerns since the Google search engine is not an educational tool but a commercial application. The Google search engine has great power to influence society as it has become university students’ key source of academic information. This impact is through manipulating the search results through personalisation, especially when that personalisation does not consider education-specific characteristics (Judd and Kennedy, 2011; Salehi et al., 2015).
Using personalisation in Web search for academic purposes can be inconsistent in a way that may deprive some students of the same opportunities to locate the best results. It was found that Google is personalising the search results in such a way that the links are only about 50% similar to non-personalised search results. Moreover, the common links often do not appear in the same priority within the result pages. Considering students' level of trust and dependence on Web search engines as academic information resources, the inconsistency of search results content and presentation could have significant impacts (Salehi et al., 2015).
However, given the obvious success of web personalisation in other areas such as e-commerce, personalised Web search if wisely employed, has the potential to benefit students. This observation is the motivation behind a user-centred study examining the role that personalised Web search plays in academic search and whether it influences students’ learning process in any way. The aim is to help Web search engine researchers and designers to bridge the semantic gap between the user and the system by highlighting the positive, and reducing the risks of long-term application of personalised Web search in higher education.
The experiment reported in this paper evaluates the effect of personalised Web search on university students’ satisfaction during their online information-seeking process. User satisfaction is a crucial part of every information system, particularly educational applications, and it is important to assess whether it is affected by personalisation of the search results. A high satisfaction level increases students’ engagement with the system and the quantity of time they spend on learning objects and as a result, enhances their learning (Steichen et al., 2009). This work aims to find out whether personalisation of search results improves students’ satisfaction with their Web search experience.
During individual semi-structured study sessions, participants were asked to complete two Web search tasks. The objective was investigate the level of students’ satisfaction with both personalised and non-personalised Web searches. For one task they used Google search while logged in with their account and for the other one they used non-personalised Google search. Then we evaluated their satisfaction level with both. The goal was to determine whether personalisation of the search results, in any way, affects students’ perception of their search and learning experiences. As this is a user-centred study, the data analysis and evaluation were done solely from the user's perspective and based on both their explicit and implicit feedback. Recorded screen activities (clicking results, timing, new or reformulated queries) plus participants’ direct feedback through filling out post-task questionnaires were used to collect data. The study sessions were all run on one search engine, Google, as it is the most popular search engine among students, both with and without its personalisation features.
Frequently-used terms
Terms used in this paper are explained in this section. A Web search query is a string of words, generally up to three (Senthil and Saravanakumar, 2013) e.g. “Credit Crunch”. The Google search engine returns a page of typically 10 result links for each query. Participants were asked to only consider the organic links that point to third party Websites and appear in the page because of their relevance, and to disregard links to advertisements or links to other services such as Google news. Participants attended an hour-long one-on-one study session with the researcher during which they completed a number of Web search tasks, such as finding the answer to a given question using Google search. During a Web search task, the participant might take several actions including submitting a query, viewing result pages, clicking on result links, reading Web documents, and returning to the Web search engine to reformulate the query or submit a new one. A Web search task is a series of interactions by the participant toward addressing a single information need (Zanganeh and Hariri, 2018). The term learning objects, widely used in e-learning literature, is used as it reflects the educational purpose of the content while allowing that content to be in any digital form.
Related work
This section reviews the application of commercial search engines in education, proven and potential advantages and disadvantages of personalisation for users in education settings, and study of user satisfaction in Web search.
Advantages and disadvantages of personalised Web search
Most university students use the Google commercial search engine for all their academic information needs and consider it as the most convenient, important and reliable academic information resource. Since Google personalises its search results for all users (Kim and Sin, 2007; Salehi et al., 2015), we focus on studies on personalisation in education.
Using personalisation in education notionally increases the time students spend on the learning materials and the system. This can be due to a number of factors, including the humanising effect of personalisation, its convenience, and efficiency of search results (Ashman et al., 2009; Haglund and Olsson, 2008; Kim and Sin, 2007). Independently of the results, personalisation can be a rewarding experience for users as it gives them the feeling of interacting with an entity who understands their likes and dislikes as opposed to mainstream software (Oulasvirta and Blom, 2008). Providing students with a personal touch makes them feel that they really matter, and it seems to increase their motivation and satisfaction with the learning process. Students’ positive attitude towards modern means of online learning is very important, since both distance and traditional education are becoming more student-driven (Ashman et al., 2014). Convenience of personalisation for students can also contribute to enhancing their motivation and engagement. Personalisation keeps the users in their comfort zone (a filter bubble) by providing them with familiar concepts in their domain of interest and filtering out many that do not meet the personalisation criteria. It is convenient for students to obtain reconcilable answers they “expect”, as opposed to unfamiliar and sometimes contradictory information (Pariser 2011a). Research has also shown that students welcome and are interested in, the use of technologies such as personalisation in teaching, and that this could increase the amount of time they spend on personalised systems (Ashman et al., 2009).
However, there are possible consequences of long-term use of personalised context in education including loss of serendipity, creativity, transparency, privacy and control (Dumais, 2014). As students rely on the search engine to decide what results are best for them, they lose control over the information they receive whereas effective learning happens when students are actively involved in shaping their own learning experience (Beetham and Sharpe 2007; Pariser 2011a).
Personalisation could also upset the balance between the mind and the environment; it limits what searchers are exposed to and therefore affects the reasoning abilities that help them to be creative. Personalised information reduces or eliminates the element of random chance by restricting users’ serendipity and exposure to contradictory information. Consequently, it upsets the learning process by decreasing chances of students’ cognitive development and ability to function well in an information-rich environment (e.g. being able to distinguish between high-quality and poor information resources). Personalised Web search also gives users a false sense of cognitive self-esteem by quickly providing the right answer, when in reality they learn and know about the world less than ever before (Ashman et al., 2014; Pariser, 2011b; Wegner and Ward, 2013). It remains an open question whether the drawbacks of using personalised information in higher education justifies the benefits.
User satisfaction
Although many studies have evaluated user satisfaction with Web search results, they mainly consist of system-centred studies to build satisfaction models, improve performance of search engines and estimate relevance. They do not necessarily assess how personalisation of search results is perceived by users, whether it affects their experience, or whether the personalisation is necessary for satisfying users (Dasdan et al., 2010; Wegner and Ward, 2013).
Hassan and Song (2011) and Hassan and White (2013) developed a user satisfaction model capable of accurately predicting satisfaction and dissatisfaction in users. The model could also be used in improving relevance estimation. Similar to the work in this paper they collected users’ explicit judgments as well as monitoring their search activity. However, their work did not focus on the satisfaction with personalisation of any kind and collected data across three search engines (Google, Bing, and Yahoo) rather than focusing specifically on the effect of personalisation within one search engine.
Dasdan et al. (2010) presented metrics that are essential for improving user satisfaction in Web search, including relevance, diversity (particularly for vague queries), coverage, freshness, geographical relevance and presentation of the search results. However, they did not factor personalisation into their metrics. Personalisation is now provided by all major search engines, and it could affect some of these metrics. For instance, it improves geographical relevance of the search results for the user, but reduces diversity by making the results specifically relevant for individual users.
Although White et al. (2013) did not measure user search satisfaction, they factored in user behaviour during search tasks and as a part of their work they investigated methods of modelling search satisfaction in order to improve personalised Web search and retrieval performance. They emphasized the importance of considering whole search tasks rather than just individual search queries during personalisation, as well as the importance of observing users’ search behaviour. This approach is used in this study for evaluating participants’ satisfaction.
It has been established that most students prefer Google to other search engines, often as their main or only information-seeking tool, with approximately 80% of them using search engines for educational purposes on daily basis. Having measured the difference between personalised and non-personalised search results for 120 academic search queries in four categories: Education, IT, Health sciences and Business. The results showed that on average only 53% of links appear, not necessarily in the same order, in both personalised and non-personalised search results for the same queries, with only slight differences in the extent of personalisation based on academic topics (Salehi, Du and Ashman, 2015). However, that work did not address the important question regarding the use of personalised Web search in education: whether personalisation of Web search increases students’ satisfaction in comparison with non-personalised Web search. That question is addressed in this paper.
Explicit and implicit feedback
Relevance feedback is very helpful for determining the quality of search results in response to a given query (Blooma et al., 2012). Relevance feedback can be deliberately given by willing searchers who answer questions such as "did this page answer your question?". This is explicit feedback and can be a very accurate representation of searcher satisfaction. This type of feedback has limited use because only a small proportion of searchers will take the time to answer. So implicit feedback mechanisms are often used. Almost any source of data generated as the searcher interacts with search engine can be interpreted as a form of implicit relevance feedback, for example, observing the ways in which searchers amend their queries if the first one is unsatisfactory (query reformulation), observing what results searchers click on from the returned set of results (clickthrough data), counting the number of queries used to meet the search need, and time taken to complete the search task. The drawback of implicit feedback is that it assumes that the searcher's actions accurately reflect their satisfaction with the search results, which may not be the case if they are side-tracked by an interesting but unrelated article, or if the result they selected turned out to be less relevant than they expected.
The use of explicit and implicit feedback is common in information systems that alter their behaviour based on the searcher's activity. Feedback provided by the users directly in response to questions about the relevance of the presented information is explicit and can often be of real value if the user's response is carefully considered. However, not all users have the time or inclination to provide feedback, and the demand for feedback increases as the providers aim to improve the effectiveness of their sites. This created the need for implicit feedback which requires no specific actions, or even awareness, by the users. Recommender systems (Oard and Jinmook, 1998; Jannach et al., 2017) and adaptive hypermedia systems (Kahabka et al., 1997) were early implementors of implicit feedback as well as explicit, with search engines rapidly expanding the research in the area (White et al., 2001).
In this experiment, participants agreed to provide explicit feedback as part of the experimental process. Combining explicit feedback with implicit feedback, in this case, clickthrough data, time taken and number of queries, reduces the possibility of misinterpreting the implicit feedback while avoiding overburdening the participants with questions about their search.
Methodology
Experimental method
The experimental methodology consists of one-on-one semi-controlled study sessions. During the study session, the participant would complete two Web search tasks to either find information on particular topics or answers to specific questions. Since students' information needs are often complex and the search process usually involves more than one query, individual queries may only point to part of the original information need. This motivated evaluation of complete search tasks rather than of individual queries. There were seven search tasks assigned across participants as follows:
- When were non-sedating antihistamines developed for the first time?;
- What is the third most common language in which Literature Nobel Prize winners write?;
- State a fact you find interesting about the Deepwater Horizon;
- State a fact you find interesting about cholesterol;
- State a fact you find interesting about Immersive Environments;
- State a fact you find interesting about the AI Learning;
- State a fact you find interesting about the Pedagogical Issues.
The data gathering methods used during these sessions were:
- Participants' recorded screen activities:
- Number of result clicks for the task;
- Number of queries per task;
- Search time per task;
- Participant’s direct feedback through filling out post-task questionnaires.
While the participants were doing the tasks, their screen activity was recorded by the Quicktime player on a Mac operating system. The recordings were then used for capturing real-time interactions and providing the flexibility of playing back videos of captured screen activity. The participants were also asked to answer a few questions to evaluate their experience after finishing each search task based on a Likert scale of 1, meaning not satisfied, to 5, meaning highly. This data collection has been widely used in analysis of exploratory user–Web interactions (Du and Spink, 2011) (Mohammad Arif et al., 2015).
The set of experiments had no time restrictions, but participants took on average between 45 to 60 minutes to complete their two search tasks and the evaluation. Although the questions and topics in each task were pre-defined, the searching session for each task only ended when the participant felt that he/she had either collected the needed information or searched enough on the topic. There also were no restrictions on what search keywords/queries the participant may choose, how and when to reformulate the queries, or which links to follow.
Scope and participants
Twenty-eight university students (46% undergraduate, 54% postgraduate) were recruited to participate in the study sessions. All the participants were required to have a Google account that they had been using regularly for at least a year prior to attending the study session.
Implementation
Sessions were run using only the standard Google search engine to ensure that satisfaction was not affected by use of different search engines. Measurable Google search personalisation accrues while users are searching with their logged in accounts, assuming these accounts normally store third-party cookies. Personalisation is mostly based on users’ geographical locations, and users’ account information such as search/browsing history (Hannak et al., 2013).
Therefore, participants logged into their Google accounts on the search engine’s main page to perform the tasks regarding personalised search while their geographical location was visible to the search engine. Account cookies and search and browser history were stored normally as set by default settings prior and throughout the experiment.
To access non-personalised search results for comparison, the following attributes were concealed from the search engine:
- Participant’s geographical location/IP address;
- Third-party cookies;
- Search/browsing history; and
- Participants’ account and demographical information.
For this purpose, the Startpage search engine on a Tor browser was used. Startpage, a privacy-enhanced search engine, returns Google search results but without passing on or storing any of user’s personal information such as IP address (location), identifying cookies or search histories (EuroPrise, 2015; StartPage Privacy Policy, 2016). During non-personalised search tasks not only were users not logged in to Google (the option to log in is not available on Startpage), but their geographical location was set to a country other than their actual location.
Startpage private searches were run on the Tor browser that consists of a modified Mozilla Firefox ESR Web browser with proxy applications and extensions that hide the user’s location and online activities by redirecting Internet traffic through an international network of over five thousand transmits. Third-party cookies and Web history are not stored in this browser (Tor, 2016). Using Startpage on the Tor browser prevents the search results from being localised based on Tor exit nodes, but exit nodes were still set to locations outside Australia. After completing each non-personalised search, the Tor browser session was terminated which automatically cleared all privacy-sensitive data.
Analysis method
Participants’ satisfaction with personalised search process was evaluated by directly comparing it with their attitude towards non-personalised search. The participants started each search task by finding a satisfactory answer to two questions. The questions were designed in a way that they were not necessarily easy to answer, as the aim was to observe the participant’s behaviour during the search process. They were pilot tested on five university students. It took the pilot participants, on average, five minutes of personalised Google search to finish each task.
The first half of the participants used personalised Google search to do the first search task, and non-personalised Google search to finish the second one. This process was reversed for the second half of the participants. This was intended to reduce the noise in the results so that the overall explicit and implicit feedback of participants after each search task would be about the search process itself and less about the possible differences in the difficulty level of questions.
For evaluation, success or failure at the task- locating the relevant information that addresses an information need, is the critical factor in the participant’s perception of the overall search experience and Web search engine’s performance. Participants evaluated their experience by filling out a Likert-type questionnaire after finishing each of their two search tasks (i.e. finding the answer to each question). Their search experience and satisfaction with the search process was assessed based on the explicit answers they provided and by observing their recorded screen activity during the search process (implicit feedback). Figure 1 illustrates the explicit and implicit feedback sources used to evaluate participants’ experience regarding personalised and non-personalised search.
Overall, fifty-six Web search tasks were completed by the twenty-eight participants. During 80% of the search tasks, the participants reformulated their queries. Query reformulation is the process of changing a given query to improve search or retrieval performance (Jansen et al., 2009; Wildemuth et al., 2018).
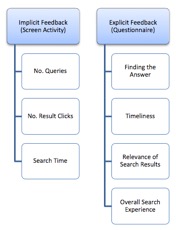
The implicit feedback provided by the following actions is important in evaluating user satisfaction with the search process:
- The number of queries made by the participant during a search task;
- The time the participant spends searching;
- The number of search result links that the participant clicks on.
Participants were asked to continue the search until they found the correct or most appropriate answer. There were no restrictions regarding the time, queries or links that they chose. Therefore, there is an inverse correlation between the implicit feedback and user satisfaction/Web search engine’s performance. As shown in Figure 2, the less time participants spend searching for an answer, the fewer queries they submit and fewer results they open until they address their information need, the better the search experience is since they are successful faster.
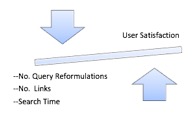
As for the explicit feedback, the participants were asked to rate their experience after each search task based on how satisfied they were with:
- Their final answers;
- The amount of time they spent searching;
- The relevance of search results;
- The overall search experiences.
Their responses were ranked based on a 5-point Likert scale from 1, meaning not satisfied, to 5, meaning highly satisfied.
Noise
Quality of collected data determines the value of any scientific study and extracted knowledge. Evaluating an attitude such as user satisfaction is scalable, but can be very noisy. In this study noise refers to meaningless information in collected data that does not accurately reflect the users’ feedback. Sources of noise in user’s implicit feedback in Web search studies have been identified as below by Sa and Yuan (2015):
- user’s prior knowledge and familiarity with the topic; reading time for users who are familiar with the topic was shorter
- user’s personal Web search strategy that could at times stray off topic and add to the search time.
- user’s interest in the topic; if the topic piqued user’s interest or he/she may want to collect additional data. Users who are interested in the topic spend more time searching and reading content, even if they have found the required information.
While the implicit behaviour in this experiment (number of queries/clicked links and search time per task) are quantifiable and we can take some measures to reduce their level of noise, explicit feedback is a user’s personal judgment and can be affected by factors that are not controllable in the experiment. However, in this experiment the users’ explicit feedback on personalised search was not evaluated exclusively but was compared to the feedback of the same group of users on non-personalised search at just about the same time. As a result, the level of noise in explicit feedback on both personalised and non-personalised search should be relatively equal and should not significantly affect their difference.
For the implicit feedback, the following measures to improve the accuracy of the results were taken:
- The number of queries and clicked links for each Web search task were noted and counted twice: once during the experiment by the researcher and confirmed later by reviewing users’ recorded screen activity;
- Using privacy-enhancing techniques during non-personalised search tasks including redirecting Internet traffic through an international network of over five thousand transmits slowed down the non-personalised search process. This could negatively affect participants’ explicit feedback on their non-personalised search experience (Figure 4).
Although we could not reduce the noise in users’ explicit judgment, we measured the delays in loading the search results and Web pages and took them out of non-personalised search time in users’ implicit feedbacks (Figure 5). The reason is that these delays were specific to this experiment and to the choice of browser and would not occur in a real-world situation.
In order to reduce the noise regarding possible differences in the difficulty level of Web search tasks, the first 14 participants carried out task one using personalised search and task two using non-personalised search. The second 14 participants did the opposite, using non-personalised search for task one and personalised search for task two. The seven tasks were each performed four times and by four different participants, twice under the personalised search settings and twice under non-personalised settings. This was intended to reduce the impact of any variation in task difficulty on the experimental outcomes.
Results
As a general rule, mean, standard deviation and other parametric analyses based on the normal distribution are suitable for analysing interval data (participants’ implicit feedback). However, when the data is on ordinal scales (participants’ explicit feedback) nonparametric procedures and distribution-free methods are the more appropriate means of data analysis (Ary et al., 2010).
The following methods were used to present participants’ implicit and explicit feedbacks:
- Implicit feedback:
- Mean, showing the average time spent searching, number of clicked links and queries;
- Standard deviation (SD), measuring the amount of dispersion in the above numbers is shown in the results graphs.
- Explicit feedback:
- Median, finding the central tendency of the responses as shown in graphs;
- Kendall's Tau-b coefficient, finding the association between users’ explicit feedback on personalised and non-personalised search. Values of Tau-b range from -1 to +1. A value of -1 would indicate a complete negative association between responses, and in this experiment would mean that participants felt very differently about their experience using personalised Web search compared to non-personalised search. A value of +1 would indicate a perfect agreement between responses regarding participants’ personalised and non-personalised search experience. A value of zero specifies the absence of association.
In order to integrate and look at explicit and implicit feedback together, participants’ satisfaction was evaluated using three factors. As shown in figure 3, each factor consists of at least two of explicit and/or implicit feedback sources. Each factor is discussed separately and then a conclusion considering all three factors together is drawn.
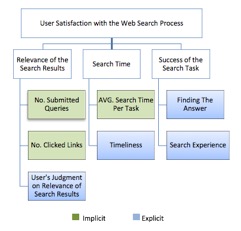
Relevance of the search results
Relevance of the search results is judged based on participants’ direct opinion (explicit feedback) and by observing how many queries they submit to the search engine and how many Web documents they read (implicit feedback). Figure 4 shows only minor differences in the relevance of the personalised search results in comparison with non-personalised search results, based only on the implicit user behaviour. Users’ judgment (explicit feedback) of what is relevant to their specific personal information needs during the search tasks seems to likewise be unaffected by personalisation of the results.
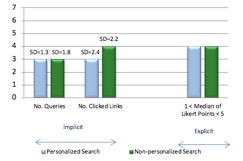
The implicit participants’ feedback is quite similar in personalised and non-personalised search results with the average number of clicked links being slightly higher in non-personalised search tasks. The range of submitted queries and clicked links per task, shown by standard deviation (SD), is also consistent for personalised and non-personalised search tasks. However, this minor difference is not reflected in users’ explicit feedback.
Search time
The less time users spend on search engines trying to address their information needs, considering their attempt is successful, the better their overall experience and satisfaction level will be. In this experiment, personalised search decreases the average search time by 12% and contributes toward improving users’ Web search practice. As shown in Figure 5, the participants spent on average 42 seconds less using personalised search compared to non-personalised search. However, once again this difference does not seem to affect their explicit feedback.
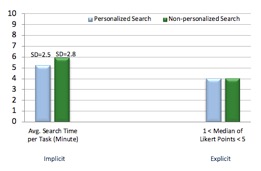
Success of the search task
Participants’ success in the search tasks was evaluated based only on their explicit feedback regarding firstly, how pleased they were with their final answers (all participants stopped the search process once they found an answer they thought was good enough) and secondly, their outlook on the overall search experience after finishing each task. As shown in Figure 6, participants’ responses regarding personalised and non-personalised search describe a similar and positive experience. In this experiment and based on users’ explicit judgment, personalisation of search results does not influence users’ perception of the search session success.
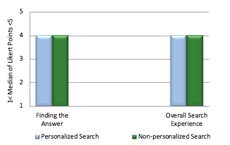
Summary of results
The results of this experiment did not demonstrate any significant difference in participants’ level of satisfaction with personalised search versus non-personalised search, even though improving users’ search experience by being able "to better provide ... the most relevant results possible" is the stated objective of personalised search.
Based solely on participants’ explicit feedback that they provided through questionnaires after completing each task, personalisation did not affect their overall search experience in any way. This is indicated by the medians of Likert points (participants’ responses) in each explicit question asking the participants to rank an aspect of their experience (relevance, time, desirable answer and overall experience). Medians were all similar (=4) for all the questions for both personalised and non-personalised search. Likewise, the Kendall's tau-b coefficient was similar, being between +0.3 to +0.4 for participants’ responses to all the questionnaire items, which indicates that any individual participant’s personalised search experience is positively related to his/her non-personalised search experience, meaning each participant had a moderately similar Web search experience regardless of whether his/her search results were personalised. This further establishes the lack of personalisation impact on users’ satisfaction within this experiment.
So, while personalisation of results in a general-purpose search engine may be perceived to be helpful to users wanting local restaurants for example, it does not improve student perceptions of the usefulness of answers. This does rather raise the question whether students would value the provision of personalisation for their educational queries.
Turning to the implicit feedback from participants, in one of the three feedback types, the number of queries, the average of the difference between personalised results and non-personalised was negligible. However, the standard deviations of 1.3 and 1.8 respectively show that some users managed to complete the personalised search task with relatively fewer queries but some also required more queries. This implies that some of the participants found the non-personalised results less immediately useful, although notably, their explicit feedback did not recognise any disadvantage.
More substantial differences were detected for the other two implicit measures, the number of clicked links and the average search time.
The number of clicked links showed an average of three for personalised versus four for non-personalised tasks, showing that participants found the relevance of non-personalised search results to be a little harder to establish. The relative standard deviations of these (at 80% for personalised and 55% for non-personalised) shows a wider variability of required clicks for personalised tasks, implying that personalisation might advantage some students but disadvantage others, in a way that acts over and above the variability in non-personalised results. However, any such apparent polarisation due to personalisation is outweighed by the clear improvement in number of clicked links in almost all cases.
The time taken to complete the search task is notably improved by the use of personalisation. An improvement of around 42 seconds and almost identical relative standard deviations shows that personalisation appears to fairly uniformly improve the time taken to complete a search task. Despite this, students did not report an improved satisfaction level with personalised results, suggesting that they did not notice (or at least did not care about) taking less time to complete the task. This calls into question any idea that users are more satisfied with a quicker search experience and warrants further investigation. That said, an important point is that students did not find the personalised experience to be less satisfactory, so if all else is equal, any improvement in productivity might be argued to justify the technology.
Students in the above experiment may have been satisfied with the results they received, but their teachers may not have agreed with them. Students are not well-placed to assess the quality of the returned search results because they are, by their very nature, less experienced in the topic they are researching. It has been shown that people will often use a search engine as a learning and discovery tool when they know little or nothing of the search topic (Smith et al. 2012) so it is difficult for students in a learning scenario to judge the quality of their results, even if they themselves perceive they have received satisfactory search results. This means they necessarily rely on the ordering of results by the search engine to provide relevant and high-quality information in the earliest pages. Whether these earliest pages of results contain the most appropriate results is not yet established, and given the broad nature of general-purpose search engines, it is likely that the results are not optimised for the student's educational purpose. Also, there is the possibility that irrelevant factors are used for personalisation, such as when personalisation took place based on factors such as location and browser, which would not contribute meaningfully to an education-related search. A useful future experiment would be to have the students' completion of search tasks assessed by experienced academics.
In summary, the experimental results reported here suggest that while student satisfaction appears to be unaffected by the presence or absence of personalisation, it does improve the time taken to complete a search task, as well as reducing the number of clicks required to arrive at the selected outcome. Thus, query personalisation arguably improves students' productivity, even if they themselves do not notice the improvement.
Conclusions
This paper reports on a user-centred study to evaluate and compare the satisfaction level of university students with personalised search vs. non-personalised Web search. We collected explicit satisfaction ratings from the participants as well as monitoring and recording their screen activity during the search tasks for implicit feedbacks. In total, about fifteen hours of screen activity was collected, comprising twenty-eight participants completing fifty-six pre-designed search tasks using personalised and non-personalised Google searches.
The results show that personalisation of the search results, which mainly occurs based on users’ geographical location, personal account information and Web search history, has a negligible effect in increasing overall user satisfaction with the system. That is an unexpected outcome, as search engines providers often state that personalisation features are designed to benefit users and enhance their Web search experience. Since their development, commercial Web search engines have found new applications along the way such as becoming the main information resource for instructional tasks and for higher education and research students. Hence, it is essential to make the appropriate adjustments in their approach to personalisation and accommodate the new trends.
Technologies such as personalisation of Web search results are not good or bad by default, as it is the way they are applied that defines their benefits and disadvantages. Therefore, it is important to constantly evaluate information technologies in their different applications to improve the performance and identify and control the risks. In the academic context, students often ignore the advice of librarians and academic staff and use a general-purpose search engine almost exclusively for researching, but this is not necessarily a problem in itself. However, the way personalisation has been applied in general search may not be suitable for students searching in the more specific academic context, as the suitability of the returned results for that context has not yet been established.
It would be perhaps better if students and researchers used topic-specific academic search tools where these are available, and indeed the usual advice from academics and librarians is to do exactly that. It would even be some improvement if students focused on a general-topic academic search engine such as Google Scholar. However, since they persistently prefer the general-purpose Google search engine, it becomes important to determine the quality, relevance and completeness of those general-purpose search results within the academic context. The next stage of this research will address this quality metric, by determining how academically useful the personalised search results are. We will investigate the parameters/factors in personalised search, in addition to students’ satisfaction, that influence students’ learning process, including whether students' opportunity to access the most relevant and original information is compromised by search engines' personalisation procedures and positioning of the search results.
The outcomes in this paper motivate future work to establish guidelines for the successful and appropriate use of search engines in an academic context. The search engines themselves may respond by seeking methods to establish whether a searcher is engaged in academic activity and to personalise returned search results based on these guidelines. While the experiment above considered only the student's perceptions of the results they received and the efficiency of the search process with personalisation, this brings a fuller understanding of the use and benefits of personalisation in the education process.
About the authors
Sara Salehi holds a PhD in computer science from the University of South Australia, awarded in 2018. She holds a consultant position in Ernst-Young in Australia.
Jia Tina Du holds a PhD in Information Studies from Queensland University of Technology, Australia. She is an associate professor at the University of South Australia, Mawson Lakes Campus, and can be contacted at tina.du@unisa.edu.au
Helen Ashman holds a Ph.D. in computer science from Royal Melbourne Institute of Technology University. She is an associate professor at the University of South Australia, Mawson Lakes Campus, and can be contacted at helen.ashman@unisa.edu.au
References
Note: A link from the title is to an open access document. A link from the DOI is to the publisher's page for the document.
- Ananatharman, L. (2012). Knowledge management and learning: eLearning and knowledge management system. In Proceedings of the 15th International Conference on Interactive Collaborative Learning, Villach, Austria 26 – 28 September 2012 (6 p.). IEEE. https://doi.org/10.1109/ICL.2012.6402171
- Ashman, H., Brailsford, T. & Brusilovsky, P. (2009). Personal services: debating the wisdom of personalization. In Proceedings of the 8th International Conference on Advances in Web Based Learning, Aachen, Germany, August 19-21, 2009 (pp. 1-11). Springer-Verlag. https://doi.org/10.1007/978-3-642-03426-8_1
- Ashman, H., Brailsford, T., Cristea, A.I., Sheng, Q.Z., Stewart, C., Toms, E.G., & Wade, V. (2014). The ethical and social implications of personalisation technologies for e-learning. Information & Management, 51(6), 819-832. https://doi.org/10.1016/j.im.2014.04.003
- Ary, D., Jacobs, L. C., & Sorensen, C. K. (2010). Introduction to research in education (8th ed.). Wadsworth/Thomson Learning.
- Beetham, H. & Sharpe, R. (2007). Rethinking pedagogy for a digital age: designing for 21st century learning. Taylor and Francis.
- Biletskiy, Y., Baghi, H., Keleberda, I., & Fleming, M. (2009). An adjustable personalisation of search and delivery of learning objects to learners. Expert Systems With Applications, 36(5), 9113-9120. https://doi.org/10.1016/j.eswa.2008.12.038
- Biletskiy, Y., Baghi, H., Steele, J. and Vovk, R., 2012. A rule-based system for hybrid search and delivery of learning objects to learners. Interactive Technology and Smart Education, 9(4), pp.263-279. https://doi.org/10.1108/17415651211284048
- Blooma, M. J., Goh, D. H.-L., & Chua, A. Y-K. (2012). Predictors of high-quality answers. Online Information Review, 36(3), 383-400. https://doi.org/10.1108/14684521211241413
- Dasdan, A., Tsioutsiouliklis, K., & Velipasaoglu, E. (2010). Web search engine metrics: direct metrics to measure user satisfaction. In Proceedings of the 19th international conference on World Wide Web, Raleigh, North Carolina, USA, April 26-30, 2010 (pp. 1343-1344). Association for Computing Machinery. https://doi.org/10.1145/1772690.1772921
- Du, J. T., & Evans, N. (2011). Academic users’ information searching on research topics: characteristics of research tasks and search strategies. The Journal of Academic Librarianship, 37(4), 299-306. https://doi.org/10.1016/j.acalib.2011.04.003
- Du, J. T. & Spink, A. (2011). Towards a Web search model: integrating multitasking, cognitive coordination and cognitive shifts. Journal of the American Society for Information Science and Technology, 62(8), 1446-1472. https://doi.org/10.1145/2362724.2362769
- Dumais, S. T. (2014). Personalised search: potential and pitfalls. In Proceedings of the 25th ACM International on Conference on Information and Knowledge Management, Indianapolis, Indiana, USA, October, 2016 (p. 689). Association for Computing Machinery. https://doi.org/10.1145/2983323.2983367
- EuroPrise. (2015). European privacy seal for Ixquick and Startpage. EuroPriSe. (Archived by the Internet Archive at https://bit.ly/35nPQlG)
- Haglund, L., & Olsson, P. (2008). The impact on university libraries of changes in information behavior among academic researchers: a multiple case study. The Journal of Academic Librarianship, 34(1), 52-59. https://doi.org/10.1016/j.acalib.2007.11.010
- Hannak, A., Sapiezynski, P., Kakhki, A.M., Krishnamurthy, B., Lazer, D., Mislove, A., & Wilson, C. (2013). Measuring personalisation of Web search. In Proceedings of the 22nd International Conference on World Wide Web, Rio de Janeiro, Brazil, May 13-17, 2013 (pp. 527-538). Association for Computing Machinery. https://doi.org/10.1145/2488388.2488435
- Hassan, A., Song, Y., & He, L. W. (2011). A task level metric for measuring Web search satisfaction and its application on improving relevance estimation. In Proceedings of the 20th ACM International Conference on Information and Knowledge Management, Glasgow, UK, 24th-28th October 2011 (pp. 125-134). Association for Computing Machinery. https://doi.org/10.1145/2063576.2063599
- Hassan, A., & White, R. W. (2013). Personalised models of search satisfaction. In Proceedings of the 22nd ACM International Conference on Information & Knowledge Management, San Francisco, California, USA, October 27 - November 1, 2103 (pp. 2009-2015). Association for Computing Machinery. https://doi.org/10.1145/2505515.2505681
- Jannach, D., Lerche, L. & Zanker, M. (2017). Recommending based on implicit feedback. In P. Brusilovsky and D. He (Eds.), Social information access (pp. 510-569). Springer.
- Jansen, B. J., Booth, D. L., & Spink, A. (2009). Patterns of query reformulation during Web searching. Journal of the American Society for Information Science and Technology, 60(7), 1358-1371. https://doi.org/10.1002/asi.21071
- Judd, T., & Kennedy, G. (2011). Expediency-based practice? Medical students’ reliance on Google and Wikipedia for biomedical inquiries. British Educational Research Journal, 42(2), 351-360. https://doi.org/10.1111/j.1467-8535.2009.01019.x
- Kahabka, T., Korkea-Aho, M., & Specht, G. (1997). GRAS: an adaptive personalization scheme for hypermedia databases. In Proceedings of the 2nd Conference on Hypertext, Information Retrieval, Multimedia, Dortmund, Germany, 29 September - 2 October, 1997 (pp. 279–292). Schriften zur Informationswissenschaf. http://citeseerx.ist.psu.edu/viewdoc/download?doi=10.1.1.53.511&rep=rep1&type=pdf
- Kim, K., & Sin, S.J. (2007). Perception and selection of information sources by undergraduate students: effects of avoidant style, confidence, and personal control in problem-solving. The Journal of Academic Librarianship, 33(6), 655–665. https://doi.org/10.1016/j.acalib.2007.09.012
- McClure, R., & Clink, K. (2009). How do you know that? An investigation of student research practices in the digital age. Portal: Libraries and the Academy, 9(1), 115-132. https://doi.org/10.1353/pla.0.0033
- Mohammad Arif, A. S., Du, J. T., & Lee, I. (2015). Understanding tourists’ collaborative information retrieval behavior to inform design. Journal of the Association for Information Science and Technology, 66(11), 2285–2303. https://doi.org/10.1002/asi.23319
- Oard, D. W., & Jinmook, K. (1998) Implicit feedback for recommender systems. In Papers from the AAAI Workshop on Recommender Systems, Madison, Wisconsin, USA, July 27, 1998 (pp. 81-83). Association for the Advancement of Artificial Intelligence. https://www.aaai.org/Papers/Workshops/1998/WS-98-08/WS98-08-021.pdf (Archived by the Internet Archive at https://bit.ly/36ynwwa)
- O’Donoghue, J. (2010). Technology-supported environments for personalised learning methods and case studies. IGI Global.
- Oulasvirta, A., & Blom, J. (2008). Motivations in personalisation behaviour. Interacting with Computers, 20(1), 1-16. https://doi.org/10.1016/j.intcom.2007.06.002
- Pariser, E. (2011a). The filter bubble: what the Internet is hiding from you. Penguin Press.
- Pariser, E. (2011b). The troubling future of internet search. The Futurist, 45(5), pp. 6-8.
- Purdy, J. P. (2012). Why first-year college students select online research resources as their favorite. First Monday, 17(9). https://doi.org/10.5210/fm.v0i0.4088 (Archived by the Internet Archive at https://bit.ly/3eVTR3S)
- Sa, N., & Yuan X. (2015). Sources of noise in interactive information search. In Proceedings of ISIC, the Information Behaviour Conference, Leeds, 2-5 September, 2014: Part 2, (paper isicsp11). http://InformationR.net/ir/20-1/isic2/isicsp11.html . (Archived by Archived by WebCite® at http://www.webcitation.org/6WxJWRw9h).
- Salehi, S., Du, J., & Ashman, H. (2015). Examining personalisation in academic Web search. In Proceedings of the 26th International Conference on Hypertext and Social Media, Guzelyurt, Cyprus, September 1-4, 2015 (pp. 103-111). Association for Computing Machinery. https://doi.org/10.1145/2700171.2791039
- Salehi S., Du, J. T., & Ashman, H. (2018). Use of Web search engines and personalisation in information searching for educational purposes. Information Research, 23(2), paper 788. http://InformationR.net/ir/23-2/paper788.html (Archived by WebCite® at http://www.webcitation.org/6zzbbBlN3)
- Sathiyamurthy, K., Geetha, T., & Senthilvelan, M. (2012). An approach towards dynamic assembling of learning objects. In Proceeding of the International Conference on Advances in Computing, Communications and Informatics, Chennai, India, August 4-5, 2012 (pp. 1193-1198). Association for Computing Machinery. https://doi.org/10.1145/2345396.2345587
- Senthil Kumar, N., & Saravanakumar, K. (2013). Web query expansion and refinement using query-level clustering. International Journal of Engineering and Technology, 5(2), 705-712. https://citeseerx.ist.psu.edu/viewdoc/download?doi=10.1.1.411.7189&rep=rep1&type=pdf
- Smith, G., Brien, C., & Ashman, H. (2012). Evaluating implicit judgments from image search clickthrough data. Journal of the American Society for Information Science and Technology, 63(12), 2451-2462. https://doi.org/10.1002/asi.22742
- StartPage. (2016). Our privacy policy.. StartPage.com (Archived by Internet Archive at https://bit.ly/32z495g)
- Steichen, B., Lawless, S., O'Connor, A., & Wade, V. (2009). Dynamic hypertext generation for reusing open corpus content. In Proceeding of the 20th ACM conference on Hypertext and Hypermedia, Torino, Italy, June 29 - July 1, 2009 (pp. 119-128). Association for Computing Machinery. https://doi.org/10.1145/1557914.1557937
- Tor. (2016). History. . (Archived by Internet Archive at https://bit.ly/3klTaSC)
- Wegner, D., & Ward, A. (2013). The internet has become the external hard drive for our memories. Scientific American, 309(6), 58-61. https://bit.ly/3kvDc8H (Archived by the Internet Archive at https://bit.ly/32HvT7z)
- White, R. W., Chu, W., Hassan, A., He, X., Song, Y., & Wang, H. (2013). Enhancing personalised search by mining and modeling task behavior. In Proceedings of the 22nd International Conference on World Wide Web, Rio de Janeiro, Brazil, May 13-17, 2013 (pp. 1411-1420). Association for Computing Machinery. https://doi.org/10.1145/2488388.2488511
- White, R. W., Jose, J. M., & Ruthven, I. (2001). Comparing explicit and implicit feedback techniques for Web retrieval: TREC-10 interactive track report. In Proceedings of the Tenth Text Retrieval Conference (TREC-10), Gaithersburg, Maryland, USA, 13-16 November, 2001 (pp. 534-538). https://trec.nist.gov/pubs/trec10/papers/glasgow.pdf (Archived by the Internet Archive at https://bit.ly/36CeYV4) National Institute of Standards and Technology.
- Wildemuth, B. M., Kelly, D., Boettcher, E., Moore, E., & Dimitrova, G. (2018). Examining the impact of domain and cognitive complexity on query formulation and reformulation. Information Processing and Management, 54(3), 433-450. https://doi.org/10.1016/j.ipm.2018.01.009
- Zanganeh, M. Y., & Hariri, N. (2018). The role of emotional aspects in the information retrieval from the Web. Online Information Review, 42(4), 520-534. https://doi.org/10.1108/OIR-04-2016-0121