Proceedings of the 11th International Conference on Conceptions of Library and Information Science, Oslo Metropolitan University, May 29 - June 1, 2022
Academic information searching and learning by use of Keenious and Google Scholar: a pilot study
Jesper Solheim Johansen, and Pia Borlund
Introduction. This paper reports a pilot study on academic information searching and learning by use of Keenious and Google Scholar. The pilot study aims to evaluate the test design, and to learn about participants’ perceptions about Keenious and Google Scholar as searching and learning systems.
Method.The pilot consists of a convenience sample of 8 students. They searched Keenious and Google Scholar in opposite order to neutralise for order effect and identified research articles of relevance to their term paper. Hereafter they curated a reading list based on their search results. After searching and curation they completed questionnaires about their experiences.
Analysis.Descriptive statistics were used to analyse the search performance results including the scale ratings.
Results. The participants perceive Keenious slightly more positively compared to Google Scholar as a system that inspires for learning and the retrieval of serendipitous results. Further, Keenious and Google Scholar are perceived to complement each other by retrieving different relevant results.
Conclusions. The pilot study demonstrates a sufficient test design. Further, the importance of counterbalancing the search systems was confirmed. The participants will be introduced to Keenious prior to testing to neutralise the bias of the participants being more familiar with Google Scholar.
DOI: https://doi.org/10.47989/colis2231
Introduction
Information technology has changed learning modes (Eschenbrenner and Nah 2019; Thanyaphongphat and Panjaburee 2019). Machionini (2006, p. 42) explains how the Internet ‘has legitimised browsing strategies that depend on selection, navigation, and trial-and-error tactics, which in turn facilitate increasing expectations to use the Web as a source for learning and exploratory discovery. Studies show how university students search the Internet with confidence (Molinillo et al. 2018; Yalcin and Kutlu 2019). To them, the Internet is a tool to acquire their learning knowledge and allows them to experience self-directed learning (Wanner and Palmer 2015). This is supported by the call for information search systems to foster learning during the search process and hereby also to facilitate a learning space which Hansen and Rieh (2016, p. 3) encapsulate as follows:
From the perspective of searching as learning, we propose to reconsider the value of search systems in supporting human learning directly while focusing on the impact, influence and outcomes of using search systems with respect to a learning process. We believe that there are great opportunities to leverage and extend current search systems to foster learning by reconfiguring search systems from information-retrieval tools to rich learning spaces in which search experiences and learning experiences are intertwined and even synergized.
Considering this call, it has been argued that recommender systems have features that correspond with principles from learning theory (Buder and Schwind, 2012). Interestingly, the application of machine learning-based recommender systems in education has increased significantly in the past two years (Urdaneta-Ponte et al., 2021). The Keenious information system is one such system developed for supporting the information searching of research literature and stimulating the learning process of the users. With this paper, we introduce Keenious and report a pilot study in which Keenious is compared to Google Scholar. The ambition of the pilot study, in addition to evaluating the test design, is to learn about users’ perception of Keenious compared to Google Scholar and to gain insights about whether users learn something new during searching and whether the searching inspires and motivates further learning.
Theoretical background
The objective of the present section is to position the theoretical background of the reported study. The point of departure is the excellent review by Vakkari (2016) on searching as learning. The review can be viewed as an introduction to the theoretical understanding of the research area of searching as learning. Vakkari (2016, p. 8) defines learning as ‘gaining new or modifying and reinforcing existing knowledge’. He further explains how ‘[u]nderstanding learning as conceptual change allows us to conceptualise knowledge structures as consisting of concepts and their relations’ (Vakkari, 2016, p. 9). Vakkari (2016, p. 9) aligns this notion to that of the cognitive viewpoint in interactive information retrieval, which conceptualises information as knowledge structures both in humans and in documents. The present paper takes the epistemological stance of the cognitive viewpoint, which is signified by the introduction of the ASK hypothesis (Belkin, 1980; Belkin et al., 1982). Thus, the cognitive viewpoint represents a significant change in the understanding of the nature of information needs, and how they are operationalised in information retrieval research (Robertson and Hancock-Beaulieu, 1992). The information need is acknowledged as connected with the individual user, contextual and potentially dynamic in nature, in contrast to how the information need is treated as a stable concept disconnected from context in the Cranfield model (Cleverdon and Keen, 1966; Cleverdon et al., 1966). According to the ASK hypothesis, an information need arises from a recognised anomaly in the user’s state of knowledge concerning some topic or situation (Belkin et al., 1982, p. 62). As such the cognitive viewpoint is concerned with the concept of an information need and its development process as perceived and acted upon by the user. At a more abstract level, the information need development process is referred to as the changes or transformations of the recipient’s knowledge structures by the act of communication and the processes of perception, evaluation, interpretation, and learning (Ingwersen, 1992). This emphasises the interactive and exploratory nature of information searching, and consequently, the type of information needs in question, which corresponds to the muddled topical information need and conscious topical information need (Ingwersen, 2000, pp. 164-5). In brief, both information needs are variable in nature, in that they concern the exploration of subject matters, but differ from each other with respect to the extent of the user’s prior knowledge of the subject matter. The Keenious system supports these types of information needs by letting the point of departure for information searching be a portion of text, either formulated by the user, e.g., text related to work in progress, or a text snippet that describes the subject matter. The Keenious system is introduced in more detail in the section below.
Introduction to Keenious
Keenious is an academic search and recommendation system in which the user’s document functions as the query instead of explicit keywords (see Figure 1). The technology underlying Keenious analyses the input text and uses the information extracted to identify and recommend relevant research articles from a large open-source dataset (OurResearch, 2022). The Keenious system is available on the web and through applications for Microsoft Word and Google Docs. Keenious aims to support students and researchers in solving everyday challenges with keyword-based information retrieval, such as limitations related to the user’s conceptual apparatus and problems of capturing the broader context in a search. Using the entire text as the basis for a search enables new strategies for exploration. Two such examples are illustrated in figure 1. Point 2 shows how a user can highlight parts of the input text to adjust the search scope. At the same time, the context of the entire text is still being considered. Point 3 exemplifies how the system suggests topics relevant to the text. The user can choose to filter by these topics to navigate the search. In summary, Keenious is developed to stimulate academic curiosity and make relevant research content more accessible. More details about Keenious can be found at https://keenious.com/.
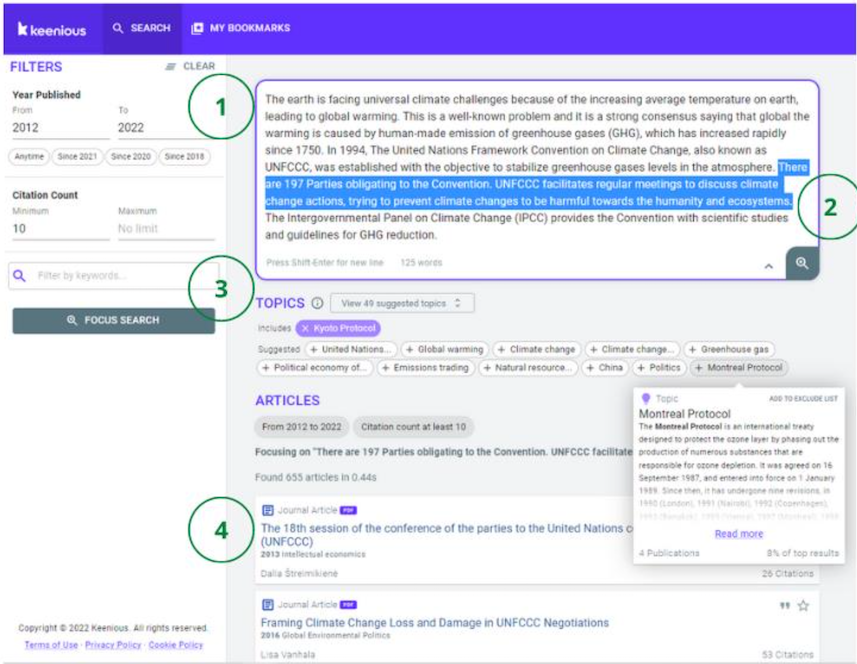
Note. (1) Field for input of text or document. (2) Focus search: the user can focus the searching by highlighting parts of the text. (3) Topic filtering: The system suggests topics relevant to the input text. The user can click to filter on a topic. (4) Results list consisting of recommended articles. Date of screenshot: 27.04.2022.
Methodology
In this section, we outline the test design and data collection methodology. The purpose is to answer the following two research questions:
RQ1: How does the performance of Keenious, from the perspective of the users, compare to Google Scholar?
RQ2: How do the users perceive Keenious and Google Scholar as systems for learning?
Google Scholar has been chosen owing to its prominent position as a tool for academic information searching (Hayes et al., 2021; van Noorden 2014). The test design is focused on collecting data to compare the within-group experience of searching as learning with Keenious and Google Scholar (Kelly, 2009), as well as the experience of combining the systems when solving a genuine information retrieval task.
Participants and recruitment
This pilot study involved 8 female students, aged 23 to 35 (mean = 25.4), recruited as a convenience sample (Kelly, 2009, p. 67) from a master’s course in business administration at the University of Tromsø, the Arctic University of Norway. On the day of the study (4.11.2021), the participants were assigned into two groups to enable counterbalancing of the search systems.
Test design
To motivate the information searching the participants were assigned a task (Borlund, 2016). The task was based on the following assignment that the students were currently working on in the master’s course:
… prepare an individual reading list with a selection of research articles and key empirical works. The reading list will form the basis for the term paper and should fulfil the requirements for academic depth in your selected area of specialisation.
The participants were informed to search for research articles to construct a reading list within the scope of their individual term paper. Hence forming the basis for realistic muddled/conscious topical information needs and search interactions.
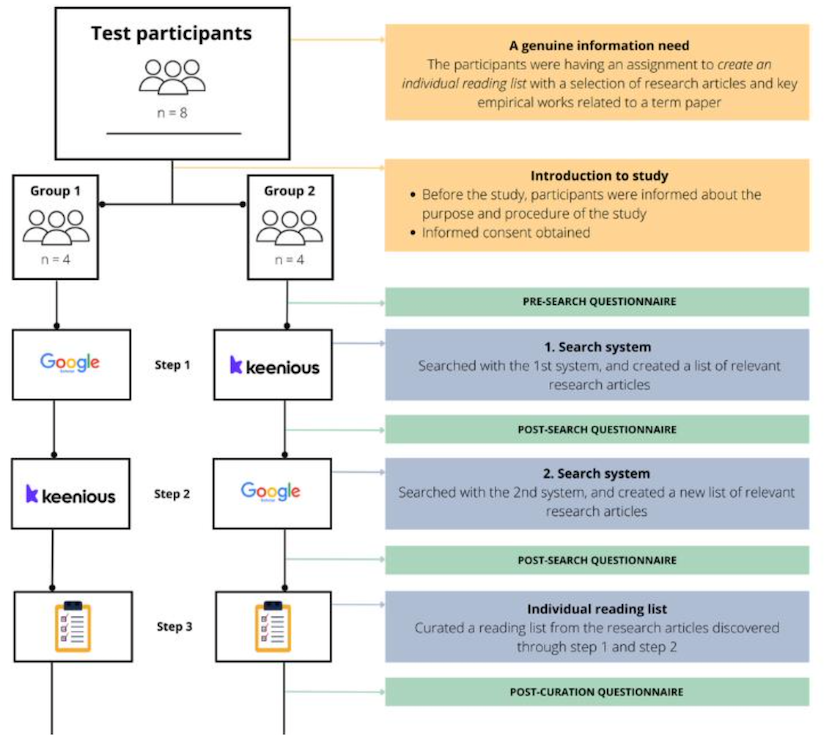
The complete test design and study procedure are depicted in Figure 2. The study opened with the participants being informed about the purpose and procedure of the study. A pre-searching questionnaire was completed to collect participants’ demography and previous search experience. By submitting the questionnaire, the participants confirmed having read the information provided and agreed to participate.
Searching for research articles (step 1 and 2).
The participants searched Keenious or Google Scholar depending on their group affiliation. The instructions were: (1) find relevant research articles to the term paper, and (2) add the APA citations for those articles in a blank document. After 20 minutes, all participants submitted their list of articles and filled out a post-search questionnaire (see Table 2). In step 2, the exact same instructions were followed using the opposite search system. A research article was not considered mutually exclusive, meaning it could appear in both lists. After searching, the list and a new post-search questionnaire were submitted. Before searching with Keenious, the interface and features were briefly demonstrated by the two experimenters. No other introduction to Keenious was given.
Curating a reading list (step 3)
The third step of the study procedure was about curating the reading list. The participants were asked to spend 10 minutes curating a reading list with respect to their term paper, based on step 1 and 2. Afterwards, a post-curation questionnaire about the experience of combining the two search systems was completed (see Table 3 for questions).
Results, discussions, and reflections on the test design
The purpose of a pilot study is to verify the study procedure and to validate that the obtained data can answer the research questions (Kelly, 2009). Hence, we report results and discussions related to RQ1 and RQ2, including reflections on the test design.
RQ1: How does the performance of Keenious, from the perspective of the user, compare to Google Scholar?
To answer RQ1 on how the participants perceive the performance of Keenious and Google Scholar we analysed the actual numbers of relevant research articles identified by the participants (step 1 and 2). Table 1 shows the results.
System system | Group 1 | Group 2 | Groups combined | |||
---|---|---|---|---|---|---|
Mean | SD | Mean | SD | Mean | SD | |
Keenious | 6.80 | 3.46 | 9.00 | 3.77 | 7.90 | 3.56 |
Google Scholar | 10.30 | 0.50 | 8.80 | 0.50 | 9.50 | 0.93 |
Note: Group 1 = Google Scholar first. Group 2 = Keenious first. |
The immediate impression is that Google Scholar performs best if the low standard deviation should be interpreted as a measure of stability. However, it is worth noting how each system that was searched first outmatch each other with higher mean values. Group 1 searched Google Scholar first with a mean value of 10.3 compared to when it was searched as the second system in group 2, then the value was 8.8. Similarly, when Keenious was searched first by group 2 the mean value was 9.0 compared to 6.8 when it was searched by group 1. This is evidence of the order effect. To us, this indicates the importance of counterbalancing the search order to minimise the order effect. It further demonstrates the importance of a larger sample of participants to answer the research question in a non-biased manner. Furthermore, the higher standard deviation values of Keenious might be a result of the participants being less familiar with Keenious compared to Google Scholar. Therefore, a direct comparison disfavours Keenious without the participants’ familiarisation with Keenious prior to testing. That said, the mean values of retrieved perceived relevance by Keenious hint at the instant and intuitive use of Keenious.
RQ2: How do the users perceive Keenious and Google Scholar as systems for learning?
We learnt about the participants’ perceptions of Keenious and Google Scholar as systems for learning via questionnaires submitted after each step. The post-searching questions quired usefulness, immediate learning, serendipity, self-efficacy, and motivation for learning and were rated according to a five-point scale (1= not at all, 5= to a very large extent) (see Table 2). The questions asked with respect to the curation of the reading lists were rated similarly.
The question regarding usefulness reads [t]he literature you found is useful for the term paper? As depicted in Table 2 the scale frequencies show how the participants rate the usefulness of the retrieved research articles by the two systems to be very similar, though slightly more positive in favour of Google Scholar. This confirms the findings of RQ1. As for the question about immediate learning, which concerns the answering of the question [y]ou learnt something new about the topic of your term paper by creating the reading list? Table 2 shows how Keenious is preferred with no 1-ratings and the awarding of scale 4-ratings in contrast to the none of Google Scholar. A study by Collins-Thompsons and colleagues (2016), investigating searching as learning, found that searchers’ perceived learning outcomes closely matched their actual learning outcomes.
Keenious does also receive better ratings compared to Google Scholar with regards to what we boldly label as serendipity. Serendipity is defined as an incident-based, unexpected discovery of information leading to an “aha-moment” when a naturally alert actor is in a passive, non-purposive state or in an active, purposive state, followed by a period of incubation leading to insight and value (Agarwal, 2015, p. 12). The question the participants were asked to rate reads [t]he list contains research articles that were relevant to you in a surprising way? The ratings show that Keenious is perceived to provide slightly more serendipitous results than Google Scholar. Neither Keenious nor Google Scholar is designed for serendipitous discoveries. However, a recent study demonstrated that user interface designs allowing user control and visualisation of research articles can facilitate serendipitous experiences in recommender systems in an academic learning environment (Afridi et al., 2019). This suggests that changing the designs could possibly foster serendipity. When asked about whether the systems have made the participants more confident about the delivery of the term paper, the participants again rated Keenious slightly more positively than Google Scholar. The issue of self-efficacy, the belief in your own capacity to successfully complete a task, is shown to be a good predictor of academic performance (Honicke and Broadbent, 2016). Therefore, it is of interest for educational institutions to facilitate self- efficacy.
Measure | Question | Search system | 1 | 2 | 3 | 4 | 5 |
---|---|---|---|---|---|---|---|
Usefulness | To which extent is the research articles you found useful for the term paper? | Google Scholar | 0 | 0 | 2 | 5 | 1 |
Keenious | 0 | 0 | 3 | 4 | 1 | ||
Immediate learning | To which extent did you learn something new about the topic of your term paper by creating this list? | Google Scholar | 1 | 3 | 4 | 0 | 0 |
Keenious | 0 | 2 | 4 | 2 | 0 | ||
Serendipity | To which extent did the list contain research articles that were relevant to you in a surprising way? | Google Scholar | 1 | 4 | 3 | 0 | 0 |
Keenious | 0 | 4 | 2 | 2 | 0 | ||
Self-efficacy | To which extent do you feel more confident in delivering the term paper after making this list? | Google Scholar | 0 | 2 | 4 | 2 | 0 |
Keenious | 0 | 1 | 4 | 2 | 1 | ||
Motivation | To which extent are you motivated to learn more after making this list? | Google Scholar | 1 | 1 | 2 | 2 | 2 |
Keenious | 0 | 1 | 0 | 4 | 3 | ||
Scale: 1 = not at all, 2 = to a little extent, 3 = to some extent, 4 = to a large extent, 5 = to a very large extent |
A related aspect is motivation, which is a key factor for learning, and hence it was an obvious question to ask the participants whether the searching for information motivated them to learn more. The participants were generous in their ratings of Keenious compared to Google Scholar in that they experience Keenious as a more inspiring and motivating system over Google Scholar. From previous research, we know that high motivation has a significant positive influence on deep learning which in turn leads to higher academic performance (Everaert et al., 2017).
The post-curation questionnaire (Table 3) also provides us with a better understanding of how the participants perceive both the performance and usefulness of Google Scholar and Keenious. Again, the participants were asked to answer the questions by five-point ratings. The first question concerned whether [t]he search results from Google Scholar and Keenious were similar? and the ratings show that only to some extent do the search results overlap. This is confirmed with the replies to the second question on whether [c]ombining the search systems, as opposed to just using one, had a positive impact on the curated reading list? Here the participants are very clear with only ratings saying “to a large extent” (5) and “to a very large extent” (3), and it is evident the two systems complement each other by retrieving different relevant results. This result further supports the poly-representation theory by Ingwersen (1996) on how the quality of search performance improves by multiple search entries. The final question is about whether the [s]earching with the second system was affected by the searching of the first system? The participants report they “to some extent” (3) and “to a large extent” (3) experience inter-system influence, which takes us back to RQ1 and the documented order effect, and hence the necessity of counterbalancing the search systems.
Measure | Question | 1 | 2 | 3 | 4 | 5 |
---|---|---|---|---|---|---|
Results similarity | To which extent were the search results from Google Scholar and Keenious similar? | 1 | 3 | 4 | 0 | 0 |
Combination effect | To which extent had combining the search systems, as opposed to just using one, a positive impact on the curated reading list? | 0 | 0 | 0 | 5 | 3 |
Inter-system influence* | To which extent was searching with the second system affected by the searching of the first system? | 0 | 1 | 3 | 3 | 0 |
* Indicates one missing value Scale: 1 = not at all, 2 = to a little extent, 3 = to some extent, 4 = to a large extent, 5 = to a very large extent. |
Another issue to consider, when reflecting on the pilot study, is the good-subject effect given the fact that one of the authors is affiliated with Keenious. This effect speaks of participants’ willingness to act as they think is desired or expected of them (Nichols & Maner, 2008). Our concern is particularly related to the “pleasing effect” seen by participants aspiring to do well to please the investigator(s) by being cooperative, and perhaps too positive towards Keenious. One way to solve this is to exclude the Keenious author from future data collection. One final item to consider based on the pilot study is to include questions in the future study about the participants’ information needs to obtain insights about how Google Scholar and Keenious satisfy the explorative muddled topical and conscious topical information needs, and whether the maturing of information needs makes a difference to how the participants use the systems.
Summary remarks
The pilot study demonstrates a sufficient test design that provides suitable data to answer the research questions. Further, the use of counterbalancing of the Google Scholar and Keenious systems was confirmed. The main study will meet the issue of the small sample of the pilot study, and the participants will be introduced to Keenious as part of the recruitment prior to testing to neutralise the bias of the participants being more familiar with Google Scholar.
About the authors
Jesper Solheim Johansen is a psychologist and the head of user research at Keenious. He holds a master’s degree in clinical psychology from UiT, The Arctic University of Norway. His research interests lie primarily in the fields of behavioural psychology and human-computer interaction, with a particular curiosity for topics revolving around information behaviour and innovative technologies. His full mailing address is: Keenious, Sykehusvegen 23, 9010 Tromsø, Norway. He can be contacted at: jesper@keenious.com
Pia Borlund is a professor in Library and Information Science at Oslo Metropolitan University. She holds a degree in Librarianship and a master’s degree in Library and Information Science (MLISc) from the Royal School of Library and Information Science, Denmark. She further holds a doctoral degree (PhD) on the evaluation of interactive information retrieval (IIR) systems from Åbo Akademi University, Finland. Her research interests cover the areas of IIR, human-computer interaction, and information seeking (behaviour). She is concerned with methodological issues, test design, and recommendations for evaluation of user-based performance and search interactions. Her full mailing address is: Oslo Metropolitan University (OsloMet), Postboks 4, St. Olavs plass, 0130 Oslo, Norway. She can be contacted at: pia.borlund@oslomet.no
References
- Agarwal, N.K. (2015). Towards a definition of serendipity in information behaviour. Information Research, 20(3), paper 675. Retrieved from http://InformationR.net/ir/20-3/pape675.html (Archived by WebCite® at http://www.webcitation.org/6bIKHqubY)
- Belkin, N.J. (1980). Anomalous states of knowledge as a basis for information retrieval. The Canadian Journal of Information Science, 5, 133-143.
- Belkin, N.J., Oddy, R. & Brooks, H. (1982). ASK for information retrieval: part I. Background and theory. Journal of Documentation, 38(2), 61-71.
- Borlund, P. (2016). A study of the use of simulated work task situations in interactive information retrieval evaluations: A meta-evaluation. Journal of Documentation, 72(3), 394-413. https://doi.org/10.1108/JD-06-2015-0068
- Buder, J., & Schwind, C. (2012). Learning with personalized recommender systems: A psychological view. Computers in Human Behavior, 28(1), 207-216. https://doi.org/10.1016/j.chb.2011.09.002
- Cleverdon, C.W. & Keen, E.M. (1966). Aslib Cranfield research project: factors determining the performance of indexing systems. Vol. 2: results. Cranfield.
- Cleverdon, C.W., Mills, J. & Keen, E.M. (1966). Aslib Cranfield research project: Factors determining the performance of indexing systems. Vol. 1: design. Cranfield.
- Collins-Thompson, K., Rieh, S. Y., Haynes, C. C., & Syed, R. (2016). Assessing learning outcomes in web search: A comparison of tasks and query strategies. In: CHIIR '16: Proceedings of the 2016 ACM on Conference on Human Information Interaction and Retrieval, March 2016, 163–172. https://doi.org/10.1145/2854946.2854972
- Everaert, P., Opdecam, E., & Maussen, S. (2017). The relationship between motivation, learning approaches, academic performance and time spent. Accounting Education, 26(1), 78-107. https://doi.org/10.1080/09639284.2016.1274911
- Eschenbrenner, B. amp; Nah, F. F. H. (2019). Learning through mobile devices: Leveraging affordances as facilitators of engagement. International Journal of Mobile Learning and Organisation, 13(2), 152–170.https://doi.org/10.1504/IJMLO.2019.098193.
- Hansen, P. & Rieh, S. Y. (2016). Recent advances on searching as learning: An introduction to the special issue. Journal of Information Science, 42(1), 3–6. https://doi.org/10.1177/0165551515614473.
- Hayes, M.A., Henry, F.A. & Shaw, R., (2021). Librarian Futures: Charting librarian-patron behaviors and relationships in the networked digital age. [online]: Lean Library. https://doi.org/10.4135/wp.20211103.
- Honicke, T., & Broadbent, J. (2016). The influence of academic self-efficacy on academic performance: A systematic review. Educational Research Review, 17, 63-84. https://doi.org/10.1016/j.edurev.2015.11.002.
- Ingwersen, P. (1992). Information retrieval interaction (Vol. 246). London: Taylor Graham.
- Ingwersen, P. (1996). Cognitive perspectives of information retrieval interaction: elements of a cognitive IR theory. Journal of Documentation, 52(1), 3-50.
- Ingwersen, P. (2000). Users in context. In: Agosti, M., Crestani, F. & Pasi, G., (eds.). Lectures on information retrieval. Heidelberg: Springer-Verlag, 157-178.
- Kelly, D. (2009). Methods for Evaluating Interactive Information Retrieval Systems with Users. Foundations and Trends in Information Retrieval, 3(1-2), 1-224.
- Marchionini, G. (2006). Exploratory search: from finding to understanding. Communications of the ACM, 49(4), 41-46.
- Molinillo, S., Aguilar-Illescas, R., Anaya-Sanchez, R. & Vallespin-Aran, M. (2018). Exploring the impacts of interactions, social presence and emotional engagement on active collaborative learning in a social web-based environment. Computers & Education, 123, 41–52. https://doi.org/10.1016/j.compedu.2018.04.012.
- Nichols, A. L., & Maner, J. K. (2008). The good-subject effect: Investigating participant demand characteristics. The Journal of general psychology, 135(2), 151-166. https://doi.org/10.3200/GENP.135.2.151-166
- OurResearch. (2022). OpenAlex dataset. https://openalex.org/
- Robertson, S.E. & Hancock-Beaulieu, M.M. (1992). On the evaluation of IR systems. Information Processing & Management, 28(4), 457-466.
- Thanyaphongphat, J. & Panjaburee, P. (2019). Effects of a personalized ubiquitous learning support system based on learning style-preferred technology type decision model on university students’ SQL learning performance. International Journal of Mobile Learning and Organisation, 13(3), 233–254. https://doi.org/10.1504/IJMLO.2019.100379.
- Urdaneta-Ponte, M. C., Mendez-Zorrilla, A., & Oleagordia-Ruiz, I. (2021). Recommendation Systems for Education: Systematic Review. Electronics, 10(14), 1611. https://doi.org/10.3390/electronics10141611
- Vakkari, P. (2016). Searching as learning: A systematization based on literature. Journal of Information Science, 42(1), pp. 7–18. https://doi.org/10.1177/0165551515615833.
- van Noorden, R. (2014). Online collaboration: Scientists and the social network. Nature, 512, 126- 129. https://doi.org/10.1038/512126a
- Wanner, T. & Palmer, E. (2015). Personalising learning: Exploring student and teacher perceptions about flexible learning and assessment in a flipped university course. Computers & Education, 88, 354–369. https://doi.org/10.1016/j.compedu.2015.07.008.
- Yalcin, M. E. & Kutlu, B. (2019). Examination of students’ acceptance of and intention to use learning management systems using extended TAM. British Journal of Educational Technology, 50(5), 2414–2432. https://doi.org/10.1111/bjet.12798