Is anybody in there? Towards a model of affect and trust in human–AI information interactions
Danica Pawlick-Potts
Introduction. Advancements in search engines that utilize machine learning increase the likelihood that users will perceive these systems as worthy of trust.
Method. The nature and implications of trust in the context of algorithmic systems that utilize machine learning is examined and the resulting conception of trust is modelled.
Analysis. While current artificial intelligence does not meet the requirements of moral autonomy necessary to be considered trustworthy, people may still engage in misplaced trust based on the perception of moral autonomy. Users who place their trust in algorithmic systems limit their critical engagement with, and assessment of, the information interaction.
Results. A preliminary high-level model of trust’s role in information interactions adapting Ingwersen and Jarvelin’s Integrative Model for Interactive Information Seeking and Retrieval is proposed using the Google search engine as an example.
Conclusions. We need to recognize that is it possible for users to react to information systems in a social manner that may lead to the formation of trust attitudes. As information professionals we want to develop interventions that will encourage users to stay critically engaged with their interactions with information systems, even when they perceive them to be autonomous.
DOI: https://doi.org/10.47989/irisic2230
Introduction
The field has long recognized that there is an affective element to the information seeking process. Kulthau (1991) and Wilson (1999) included uncertainty in their models as a key element at the beginning of a user’s search process. The use of algorithmic search engines appears to reduce the amount of decisions that a user makes in their search process. Take Google for example: Google interprets whatever words the user places in the search box, is able to search a multitude of sources—allowing the user to forgo identifying and selecting different search databases and web sites—and can determine what it believes to be the most relevant search results based on the search words as well as other factors it has learned. Research has shown that some people display a very high level of trust in Google, including its ranking algorithms (Andersson, 2017; Sundin and Carlsson, 2016; Sundin, et al., 2017). In some cases, we may even be able to say that Google search has replaced user’s stage of uncertainty with trust.
Trust, however, is an amorphous concept with no universal definition. In undertaking a review of empirical research on trust and AI, Glikson and Wooley (2020) found that the bulk of research focused on a rational-cognitive accounts of trust—particularly robust in the area of robotics, with limited research on affective trust—particularly sparce in the area of embodied AI. If we look to fields such as information literacy or the emerging field of algorithmic literacy (Ridley and Pawlick-Potts, 2021), it is clear that as librarians and information professionals we value and encourage user’s critical engagement towards both information sources and the information seeking process itself. I take the view that trust in itself is a neutral phenomenon that can be helpful or harmful depending on the context in which it arises, but it is important for us to understand how it functions in the information seeking and retrieval process. Trust is not necessarily an inherently rational phenomenon and trades critical assessment and carefully informed choices for reducing complexity and decision-making efforts. When we trust someone, we rely on them to do whatever it is we trust them to do without our oversight or interference—it is a way of coping with the uncertainty that stems from the freedom of others. This is significant because trust, by its nature, leads people to be vulnerable and more likely to accept information without further questions once trust has been established.
Rational cognitive accounts of trust likely dominate because they are relatively straight forward to apply in information system contexts because they are grounded in features akin to risk assessment and can more easily be operationalized for study and system design. Information systems have also historically lacked any meaningful autonomy or discretionary power. If, however, we look to the philosophical literature on trust we see that a central issue to seeking a conception of trust is in distinguishing trust from mere reliance. This distinction is more than semantics and should be considered in how we approach the issue of trust in information behaviour. Motivation-attributing accounts of trust require there to be at least the perception that the subject of trust possesses autonomy and discretion in the interaction—that discretion is what creates the vulnerability that makes trust truly useful.
When it comes to AI, most agree the technology does not possess any meaningful moral autonomy; however, as algorithmic systems advance there is the opportunity for some kinds of discretionary power. This is critical because under an affective-motivation attributing account of trust it is inappropriate to talk about trust except between two autonomous agents. Meaning, simply the perception of autonomy may elevate an interaction from one of mere reliance to trust. That brings us to the crux of the issue as it is extremely debatable whether current AI technology, especially that in commercial use, qualifies for autonomous agent status, but trust is not necessarily based on technical realities, but on the user’s perceptions. As most of us probably know, it is entirely possible to trust someone who is untrustworthy. So, what matters when we are concerned with our users, is how they perceive and react to the information systems.
The first half of this paper will argue for the utility of considering an affective motivation attributing account of trust in information interactions because of the impact of perceived motivation or goodwill on a user’s critical engagement. The second half of the paper will propose a high-level adaption of Ingwersen and Jarvelin’s (2005) Integrative Model for Interactive Information Seeking and Retrieval that can accommodate the affective dimensions of trust.
Conceptualizing trust
Though widely discussed across many fields, there is not a unified definition or understanding of trust. However, there are some key features shared across most definitions. First we must establish the kind of trust interaction we are interested in: a particular interaction between two agents in a particular context. Generally, it is agreed that trust requires you to place something in another’s care—and the something can be interpreted broadly. The thing we are trusting artificial agents to do in the case of information interactions is to make judgements about which sources to recommend to a user given the ‘numerous sources relevant to a given query, ranging from institutional to personal, from government to private citizen, from objective report to editorial opinion, etc’ (Artz and Gil, 2007, p. 58). Trust additionally requires three things:
- that we are vulnerable to betrayal by others;
- that we think well of others in the relevant domain; and
- that we are optimistic that they are competent in relevant respects (McLeod, 2020)
The idea of vulnerability is integral to the idea of trust. Another way of looking at it is that you need to be taking a risk of some kind, risk must be perceived by the trusting party. This idea of vulnerability is key. Trust endures, and some may argue becomes truly useful, when one is not able to monitor or constrain the person whom they are trusting. This suggests that trust requires some degree of discretion and/or freedom on the part of the trustee (McLeod, 2020; Baier, 1986). All of these things must be present to some degree but do exist on a sliding scale and vary based on the context and nature of the relationship.
Rational cognitive vs affective motivating accounts of trust
Armed with the foundation of trust, we can examine the difference between two competing accounts of trust: rational cognitive and affective-motivation attributing. Rational cognitive is the predominant approach to trust in the context of technology and information technology, but here we will demonstrate the utility of motivation-attributing accounts of trust when considering information behaviour, especially in the context of systems that utilize artificial intelligence.The key difference between the two accounts lies in the source of the trustor’s confidence that the trustee will do that with which they are trusted. A rational cognitive approach to trust is put simply, predicting the probability that the trustee will uphold the trust placed in them. It is a simple risk assessment (Nickel, et al., 2010). It is easy to see why this is favoured in the technology literature—you can simply use performance values such as a system’s reliability to determine the likelihood of a particular outcome and determine if that is an acceptable risk. Such an approach is certainly rational, and in most cases what we would consider an ideal way to determine whether or not to rely on a technology… but that is just it—it is simple reliance. We can turn to Baier’s (1986) critical question: what is the difference between trusting others and simply relying on them? If we take a rational cognitive approach to trust—there is not one. It is also unclear when you might feel betrayed and when you would simply accept that you knew there was a probability that the trustee would not fulfil the trusted action.
Here is where the idea of betrayal becomes important. An example that is often used is that we can rely on a chair, but if that chair were to break when we sat down, we would not feel that the chair betrayed us. The chair itself did not have any control. You cannot feel betrayed by something that does not possess free will or any kind of meaningful discretion. This distinction between reliance and trust is often used to explain why trust is only appropriate for agents, and not inanimate objects. Motivation attributing accounts of trust explain that we cannot feel betrayed by an inanimate object because it does not possess a will to have any kind of motive or ability to control its actions.
One influential motive attributing approach to trust is Jones’ (1996) will based account of trust. Will-based accounts define the motive as goodwill — that those we trust will be ‘directly and favourably moved by the thought that we are counting on [them]’ (Baier, 1986). Jones (1996) argues that we can view our assessment of another’s trustworthiness as divided between cognitive and affective or emotional elements (p. 5). The affective element allows us to trust based on an attitude of optimism that we believe another has a reason for feeling goodwill towards us; the reasons for this can vary, provided we believe they are moved by the thought that we are counting on them. An affective account differs from others because we accept that the assessment of trust is influenced by affective elements such as emotions and does not only rely on evidentialism. Evidence is instead interpreted through an emotional lens which ‘suggests a particular line of inquiry and makes some beliefs seem compelling and not others’ (p. 11). Examining trust as an affective attitude also highlights the importance of perceptions, and how perceptions can be incorrect—either by the trustor’s own misconception, or deliberate manipulation.
Moral agency, perception, and impact
We have established that undertaking an affective motivation attributing account of trust means that to be trustworthy one must have an agency that allows them to hold attitudes such as goodwill towards another, i.e., they must possess moral agency (McLeod, 2020). This type of goodwill can also be linked to the positive correlation in personalization and trust between humans and algorithmic systems which speaks to the perceived motivation and commitment to a particular user (Glikson and Wooley, 2020). Though it is largely agreed that current algorithmic systems, even those that utilize machine learning and are considered AI, do not meet the requirements for true moral agency, advancements in machine learning introduced the possibility that algorithmic systems do make decisions and possess some kind of discretionary power—but is it enough to be considered a moral agent?
Reality of moral agency
Most standard moral theories draw heavily on the theory of mind and internal states to argue that moral agency requires free-will and to know the difference between right and wrong. The absence of motivation-attributing accounts of trust can be attributed to the common position that current algorithmic systems lack the ability to have the knowledge and discretionary power to understand the moral consequences of its decisions and actions and to act accordingly (Nickel, et al., 2010). Ryan (2020) succinctly puts it that ‘AI may be programmed to have motivational states, but it does not have the capacity to consciously feel emotional dispositions, such as satisfaction or suffering, resulting from caring, which is an essential component of affective trust’ (p. 2760). We would be trusting search engine agents to make judgements about the quality of the sources and content relevant to us. Here we are interested not in the trust judgements the system itself must engage in but the user’s beliefs about the system’s capabilities. It has indeed been argued that artificial agents may excel in reckoning but are not equipped to make value judgments (Smith, 2019). Algorithmic systems lack the sufficient internal states to be considered moral agents capable of value judgements and thus are not worthy of trust.
Floridi and Sanders (2004) do propose an account of moral agency that separates moral agency from moral responsibility by proposing a ‘mind-less morality’. They propose that a system can be considered a moral actor if it displays interactivity, autonomy, and adaptability. Under this account of moral agency systems can act in a way that has moral consequences on the stakeholders involved, despite lacking the required inner states to possess free-will that would imbue responsibility for those actions. While this account of moral agency would allow us to extend algorithmic information systems the status of moral agency, such a status is insufficient for a morally laden interaction such as trust where the moral agency component hinges on the feature of free-will or at least some kind or morally laden intentionality such as goodwill.
Perception of moral agency
While we have established that current algorithmic systems are not trustworthy, trustworthiness is not the same as a trust stance. This is an important distinction because misplaced trust is still possible and still carries the moral and ethical implications associated with trust. In this case, the perception that an algorithmic system possesses some degree of the moral agency required to have a motivation such as goodwill—even if the technical reality says otherwise. There are two things that can happen here that could potentially influence trust, one conscious and one subconscious. The first is that someone could consciously perceive an information system to have some degree of autonomy to the point that they perceive the system to be acting in their best interests, likely due to some degree of anthropomorphising—similar to trusting a human information professional to have the intention to meet a user’s information needs. There is evidence that people may have unrealistic optimism about the abilities of new technology and lab-based studies have demonstrated that people tend to exhibit high initial trust in embedded AI as an algorithmic decision-providing software (Glickson and Wooley, 2020; Dzindolet, et al., 2003). When we look at existing research on trust and Google, there is some evidence in participant’s responses to suggest that they are anthropomorphising Google to a certain extent and perceive something akin to Goodwill towards them (Andersson, 2017; Pan, et al., 2007).
Coeckelbergh (2009) argues that when considering the moral agency of others, we should shift our emphasis from the ‘inner’ reality and truth to the ‘outer’ appearance and performance. The justification is a normative one—that even with humans we judge others by their appearance and outward behaviour. We do not have access to their inner states beyond imagining. When interacting with an agent, the moral significance of the interaction is on the outward effect, and the perception of the agent’s moral status is just that—perception. Regardless of the ‘internal’ nature of the system we ascribe moral responsibility based our experience and perception of other agents, ‘if we perceive moral agency in the other, we also hold the other responsible’ (p.188). We do not need to go so far as Coeckelbergh in needing to establish algorithmic systems as moral agents—what is important is to establish that in interactions with people—i.e. users—that those people will decide whether or not to trust a system based on their perceptions of autonomy and discretionary power. If a human perceives an algorithmic system to possess moral agency, they will ascribe responsibility and make their decisions based on that knowledge.
We are concerned with the information seeker’s model of the algorithmic system and its capabilities, not the technological and philosophical realities of the system’s status as a moral agent. If users view Google search as acting in their best interest, then they are perceiving it to fulfil the goodwill requirement.
Subconscious social reactions and premature cognitive commitments
The second potential influence is subconscious, and as we discussed earlier trust is not inherently rational—subconscious responses such as emotion can have an impact on whether or not trust occurs. While not specific to trust, a collection of empirical research, known as ‘Computers as Social Actors’, shows that humans demonstrated a tendency to respond to computers socially as early as the 90s. In their influential paper Machines and Mindlessness, Nass and Moon (2000) summarized the findings of a series of experiments examining how humans interacted with computers. The studies found that people demonstrated social behaviours such as politeness and reciprocity towards computers, made premature cognitive commitments, and responded to the perceived personality of a computer—and these we basic computational machines, no suggestion of artificial intelligence. In fact, a condition of the mindless response in the experiments was that while the object should have enough cues to lead someone to categorize it as worthy of social response, someone sensitive to the entire situation would determine those social responses were inappropriate. When interviewed, participants ‘vehemently denie’that they would react to a computer in a social manner. They held no conscious beliefs that the technology possessed human qualities that made it worthy of social responses.
A possible explanation for the experiments was overlearned social behaviours and so the group conducted experiments on premature cognitive commitment. Simply labelling televisions to create the perception of expertise, i.e. new compared to news and entertainment, led participants to believe the news segments were of higher quality—despite seeing the exact same content as the generalist group. Similarly, in another experiment that had the computers emulate dominant and submissive personality types, participants were more likely to assign greater intelligence to and conform more to the computer that emulated their own personality type. Further, they were more likely to give the computer credit for successes and less likely to attribute blame for failures.
This idea of premature cognitive commitment echoes how trust acts as a kind of premature cognitive commitment and can lead people to interpret evidence differently depending on whether or not they trust someone. It is also easy to see how people being told that a system employs artificial intelligence might perceive the system as having more autonomy than it truly demonstrates. These findings are significant as trust is a social phenomena and these findings show that it is possible for people’s behaviour to reflect subconscious social reactions and even premature cognitive commitments to technology even if they would not consciously ascribe human characteristics to that technology. In other words, people could act as if they trust a system even if they did not consciously ascribe moral agency to that system. In the next section we will explore why the ascription of moral agency, even erroneously or subconsciously, is significant for user’s information behaviour.
Ethical implications of trust
It is important to consider motivation attributing accounts of trust from an ethical standpoint concerned with our users. We are not simply distinguishing between the two approaches to trust as a simple issue of semantics. There are different ethical considerations and implications depending on which type of trust you are talking about.
If we take a rational cognitive approach to trust, we are assuming that the trustor is making a conscious decision on whether or not to rely on the trustee. This can still, of course, be a misguided or misinformed decision, but we are assuming that the trustor is indeed making a rational decision based on information available to them. That is different that an affective motivation attributing account of trust where, as we have established, trust is not derived entirely from evidence and can be formed based on affective influences and incorrect perceptions of the motives of another.
Trusting thus functions analogously to blinkered vision: it shields from view a whole range of interpretations about the motives of another and restricts the inferences we will make about the likely actions of another. Trusting thus opens one up to harm, for it gives rise to selective interpretation, which means that one can be fooled, the truth might lie, as it were, outside one’s gaze. (Jones, 1996, p. 12)
As we have just seen, it is possible for people to subconsciously perceive and react to computers much less advanced than AI in a social manner. When it comes to information seeking and retrieval, as evidenced by the field of information literacy, we do not want to encourage or engender trust based on anything other than critical assessment of the trustee, in our case, information systems. In a way we want users to trust in a rational cognitive sense—when appropriate. It is important to distinguish this other type of trust that forms sometimes without us even being able to articulate exactly why. If that trust stance sets in, the trustor stops being critical of the trustee. An extreme example of this is how often people will trust close friends or family members who then commit some sort of crime, and refuse to consider any evidence regardless of how compelling and/or damning it may be. Now in our context there is not likely to be such a high degree of trust, but it does illustrate that trust has the potential power to stop someone from engaging in critical thought relevant to the subject of trust.
The nature, and utility, of trust is that it is supposed to reduce complexity and allow us to believe that something will get done without out intervention or oversight—you no longer need to worry, or even think about it. We do not want users to do that with information systems, especially if they do not come to trust a system because of critical assessment in the first place; ‘Well-placed trust grows out of active inquiry rather than blind acceptance’ (O’Neill, 2002). If a user develops affective-motivation attributing trust towards a system they will limit their critical assessment of the information system itself, and possibly the information provided as they will trust the system to provide them information that meets their needs.
Additionally, a more insidious issue is when developers identify trust as a useful tool to increase adoption of new technology. We should be all for the development of trustworthy technology, however, it is ethically questionable to develop technology that is designed to play on psychological cues that may subconsciously encourage a user to see a system as trustworthy. As we saw with the computers as social actors paradigm, it is entirely possible to design a system that will play on certain social cues to elicit social reactions such as trust from a user that would not otherwise consider the system to be trustworthy.
Towards an information interaction model of affect-motivation attributing trust
If we want to take on the role of intervening in the development of inappropriate trust towards algorithmic systems, we should have some idea of where trust fits into an information interaction. To this end we can explore the adaption of an information interaction model, in our case Ingwersen and Jarvelin’s (2005) Integrative Model for Interactive Information Search and Retrieval. The model’s use of the cognitive viewpoint is useful for adaption a perception based social interaction such as trust as it centres cognitions and the interaction between mental models. Understanding the influence of the cognitive viewpoint allows us to see how the model allows us to visualize and better understand how trust functions in an information interaction.
The cognitive viewpoint and algorithms
The cognitive viewpoint does not have a precise, commonly understood definition, but a commonality to most definitions in information science it is that cognitive processes occur at both end of any communicative process (Belkin, 1980). The cognitive viewpoint is epistemologically ‘based on the conception of social interaction between individual cognitive structures in context’ (Ingwersen and Javelin, 2005, p. 30). The appeal is in the holistic nature of the framework. In information science the cognitive viewpoint has been used to explore mental representations of knowledge, intentions, beliefs, texts, etc. and to use representation as a way to understand the interactions between users, systems and information (Belkin, 1989).
A key understanding in the cognitive viewpoint is the understanding that any actor’s (machine or human) cognitive state is influenced by its experiences as well as its social and cultural context (Ingwersen and Jarvelin, 2005). The cognitive state is composed of cognitive structures such as knowledge structures, perceived social experiences, education, etc. but also includes emotional states. Each actor can also include any other actor in their cognitive models—including machine actors such as algorithms but also actors not directly present in the process such as designers and developers; in these cases, the actors are represented by their work and influence on the system and process (Ingwersen and Jarvelin, 2005). A subject’s cognitive models not only interact with the other cognitive models within their cognitive space, but also the cognitive models of other actors.
The cognitive viewpoint provides a foundation to view information interaction as a highly networked process that includes emotional and affective perceptions and structures within their conception of cognitive models. The model reflects the interactive and holistic perspective of the cognitive viewpoint, particularly in its understanding of the relationship between users and the searching system. Models of context are the result of a ‘shared process of interpretation and adaption on both sides of the interface’ (Ingwersen and Jarvelin, 2005, p. 281). The model recognizes that the technical characteristics of the system and its interfaces influence the user’s perception of the system and its capabilities, and this influences perceptions and interpretations of both the process and the informational objects. Information models that make use of the cognitive viewpoint are primed to be able to accommodate trust as a phenomenon with a potential to influence the information interaction. Particularly our conception of an affective motivation attributing trust that needs a model that includes how additional factors such as experiences and emotional to influence perceptions of an information system and the information interaction itself.
Information interaction
An interaction is a reciprocal event where two objects influence each other with their actions and thus communication is central (Savolainen, 2018). Early conceptions of information interaction evolved from librarians engaging in open ended interactive question negotiation processes (Taylor, 1968) and thus emphasized the human-to-human communication process. Traditionally the term human information interaction has been used, however, our interest is in adapting these concepts to AI systems that some people may afford some degree of moral agency and modelling the affective dimensions of trust in human – AI interactions. From now on it will be referred to as simply information interaction.
Search engines, uncertainty and discretion
It is important to re-emphasize that we are interested in search systems such as Google that are powered by machine learning algorithms. These systems are able to make discretionary decisions about the search process and what results to show a user. In search engines that do not utilize machine learning it is easy to understand why you are seeing those specific search results in that specific order. The results you see are the documents that meet the search parameters that users prescribe.
Let us use Google Search as an example to further illustrate some of the discretionary decisions that can occur in a search process on behalf of a user. The search algorithm component can be broken down into 3 further components: the analysis of a user’s query, matching search results, and ranking search results. Google search uses natural language processing to interpret a user’s search and to understand the meaning of a search. The search is not limited to a query exactly as it is entered as is the case with traditional academic search engines. Google search also utilizes other information such as location and past searches to try to contextualize a search query and meet unspecified information needs. By interpreting searches beyond the exact query, the search engine is attempting to articulate a user’s information need more precisely than the user has. The search engine then runs its interpreted search against the search index and knowledge graph. The search engine is not simply looking for a simple keyword match, the exact nature of how the search engine is selecting search results and the criteria it is using is hidden from scrutiny.
According to Google, the algorithms analyse hundreds of different factors in order to select and rank results. Google provides a few examples such as ‘freshness’ of content and ‘the number of times your search terms appear’ (Google, 2018) which are similar to traditional database search criteria and are relatively objective and concrete. However, they also cite other factors such as ‘whether the page has a good user experience,’ and looking at how many ‘prominent websites’ on the same subject link to the page and whether or not many users seem to ‘value’ the page for similar queries (whatever that means) as way to assess trustworthiness and authority (Google, 2018). These other factors introduce a subjective element of judgement into the search result selection process. The search algorithm is constantly analysing and learning what search results are received well and constantly adapting itself based on that analysis, that is what makes the search engine so successful at giving people satisfying results. However, that is also why the search process is so opaque to the user. The black box nature of the search algorithms is what invites a user to take a trust stance towards the system—remember that the utility of trust is in reducing uncertainty when you cannot completely oversee another agent and their actions.
Ingwersen and Jarvelin’s integrative model
Ingwersen and Jarvelin’s (2005) integrative model includes four key interactions: (1) between a user’s cognitive space and the context of information seeking and retrieval, (2) between the information seeker and interface, (3) between the information objects, information technology and interface, and (4) the retrieval of information objects by means of information technology (Savolainen, 2018). The model is structured and technical, reflecting their interest in the development of information seeking and retrieval systems. For our purposes we will interpret the framework broadly and translate and describe concepts in a way that is more in line with information practice theory and research.
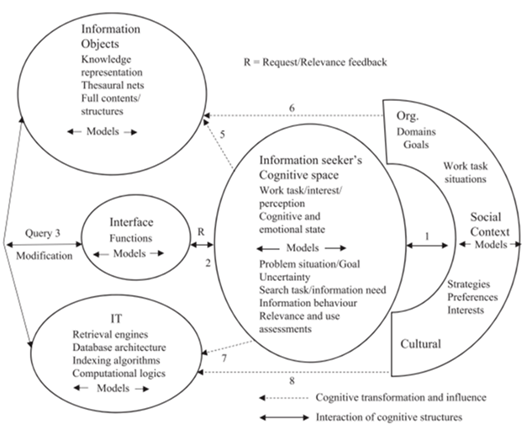
Ingwersen and Jarvelin’s (2005) model consists of five central components: the information technology setting; the information space holding objects of potential information value; information seekers; interface mechanisms; and socio-organizational contexts. Each of the components includes the data and cognitive structures of all actors involved in their development and transformations. The notion of context is central to the model and seen as the culmination of an actor’s experiences and knowledge—a very broad an all-encompassing conception which includes many factors such as social and cultural influences, work and daily-life tasks, emotional interests and personal preferences. Each component of the model represents the perception of the context of that actor or entity.
The model’s primary goal is to represent information seeking and retrieval, but it also encompasses other relevant information behaviours such as use, creation, communication, selection of information objects and indexing of such objects. These behaviours are originally characterized as human, however, we will use the characterization as agential instead to recognize that machine learning algorithms can be capable of these behaviours as well. Further, we will shift the focus from the cognitive models of the developers to that of the algorithm, while still recognizing that this model is influenced by its developers.
Ingwersen and Jarvelin’s (2005) model includes emotional and affective perceptions and structures within their conception of cognitive models. The model reflects the interactive and holistic perspective of the cognitive viewpoint, particularly in its understanding of the relationship between users and the searching system. Models of context are the result of a ‘shared process of interpretation and adaption on both sides of the interface’ (p. 281). The model recognizes that the technical characteristics of the system and its interfaces influence the user’s perception of the system and its capabilities, and this influences perceptions and interpretations of both the process and the informational objects.
Modelling affect based trust in artificial agents
The key difference in our high-level adaption of Ingwersen and Jarvelin’s (2005) model is that we are recognizing Google search as a distinct informational agent capable of some levels of interpretation and creating its own cognitive models and frameworks for engaging in informational practices. Ridley (2019) proposes a description of autonomous information behaviour, one that is distinct from human information behaviour, and one that acknowledges the (disputed) agency of artificial intelligence. Ridley proposes that while both share the general behaviours: needs, seeking, managing, giving and use, they are undertaken by different processes in different contexts. This idea is very pertinent when considering an autonomous algorithmic system such as Google, and the idea that it is distinct from a mere computational system, but not the same as a human, and therefore introduces distinct considerations for human information practice models. By recognizing Google Search as an, at least, partially autonomous agent, that can be perceived as such, we can explore how this impacts informational interactions between the system and its users—particularly the cognitive models the users develop of the system and the information search process.
Recognizing that Google search displays a reflexivity and adaptability that could be conceived as having a cognitive model, this allows us to add the features and elements into the model to better be able to identify and discuss the relevant interactions and relationships (see Figure 2). Under the information seeker’s cognitive space, we can add the trust models, which integrates the user’s model of Google search and the search process, either conscious or unconscious perception. Under information objects we include the web pages and the search results that the interface displays to the user, as well as the search index and knowledge graph that is accessed by the search engine (although users can access the search index and knowledge graph, this is not done in a typical Google search process). Lastly, under information technology, is where we place Google search itself and the algorithmic systems that comprise it.
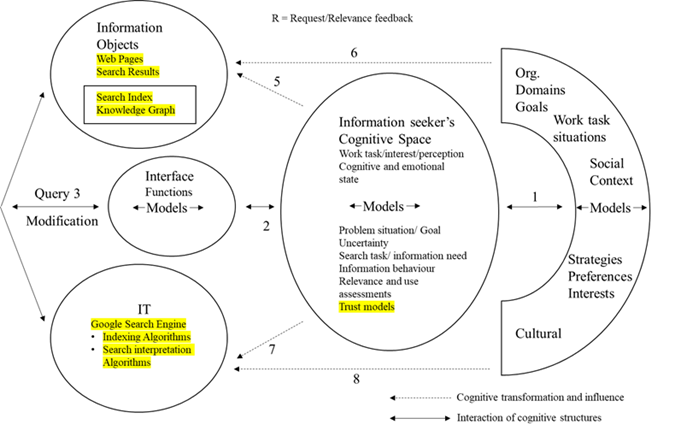
This may look simplistic and that we really could wedge any social phenomena such as trust into the information seeker’s cognitive space, but it is important to recognize that this is a high-level model that requires further empirical research into affective-based trust on embedded algorithmic systems to detail further. It is also critical that the interactions between the models within a user’s cognitive space are captured. By focusing on the information seeker’s cognitive space, we can see that the model includes emotional and affective perceptions and structures within their conception of cognitive models which shows the model is amenable to recognizing subconscious influences, like those we saw in the Computers as Social Actors paradigm, to the users cognitive space and resulting behaviour. The model of the interface will influence the trust model and vice versa. This is not intended to be comprehensive but to begin to give an idea of how we can model trust for further investigation.
Conclusion
We need to recognize that it is possible for users to react to AI, and even non-AI, information systems in a social manner that may lead to the formation of trust attitudes. As information professionals we want to develop interventions that will encourage users to stay critically engaged with their interactions with information systems, even when they perceive them to be autonomous. This work could be done under the umbrella of information or algorithmic literacy. More research is needed to understand what particular contexts and factors are likely to lead to the development of misplaced trust in order to determine appropriate interventions. Google was used as an example throughout this paper, but the potential for perceptions of autonomy and intentionality is likely to increase with the development and deployment of interactive information systems, particularly those that employ avatars as some kind of agential personality where the system is explicitly anthropomorphised—if people react socially to televisions with signs what is the potential impact of interactive agents?
Acknowledgements
The author would like to acknowledge the support of Dr. Jacqueline Burkell and Dr. Paulette Rothbauer. This research was also supported by the Vector Institute.
About the author
Danica Pawlick-Potts is a PhD student, Faculty of Information and Media Studies (FIMS), Western University, London, Ontario, Canada. Her research interests are artificial intelligence ethics, information behaviour and Indigenous data. She can be contacted at dpawlic@uwo.ca
References
- Andersson, C. (2017). “Google is not fun”: An investigation of how Swedish teenagers frame online searching. Journal of Documentation, 73(6), 1244-1260. https://doi.org/10.1108/JD-03-2017-0048
- Artz, D., & Gil, Y. (2007). A survey of trust in computer science and the semantic web. Journal of Web Semantics, 5(2), pp. 58-71. https://doi.org/10.1016/j.websem.2007.03.002
- Baier, A. (1986). Trust and antitrust. Ethics, 96(2), 231-260. https://doi.org/10.1086/292745
- Belkin, N. J. (1980). Anomalous states of knowledge as a basis for information retrieval. Canadian Journal of Information Science, 5(1), 133-143. https://tefkos.comminfo.rutgers.edu/Courses/612/Articles/BelkinAnomolous.pdf (Internet Archive)
- Belkin, N. J. (1989). The cognitive viewpoint in information science. Journal of Information Science, 16(1), 11–15. https://doi.org/10.1177/016555159001600104
- Coeckelbergh, M. (2009). Virtual moral agency, virtual moral responsibility: on the moral significance of the appearance, perception, and performance of artificial agents. AI & Society, 24(2), 181-189. https://doi.org/10.1007/s00146-009-0208-3
- Dzindolet, M. T., Peterson, S. A., Pomranky, R. A., Pierce, L. G., & Beck, H. P. (2003). The role of trust in automation reliance. International journal of human-computer studies, 58(6), 697-718. https://doi.org/10.1016/S1071-5819(03)00038-7
- Floridi, L., & Sanders, J. W. (2004). On the morality of artificial agents. Minds and Machines, 14(3), 349-379. https://doi.org/10.1023/B:MIND.0000035461.63578.9d
- Glikson, E., & Woolley, A. W. (2020). Human trust in artificial intelligence: Review of empirical research. Academy of Management Annals, 14(2), 627-660. https://doi.org/10.5465/annals.2018.0057
- Google. (2018). How search algorithms work. https://www.google.com/search/howsearchworks/algorithms/ (Internet Archive)
- Ingwersen, P., & Jarvelin, K. (2005). The turn: Integration of information seeking and retrieval in context. Springer.
- Jones, K. (1996). Trust as an affective attitude. Ethics, 107(1), 4-25. https://doi.org/10.1086/233694
- Kulthau, C. C. (1991). Inside the search process: Information seeking from the user’s perspective. Journal of the American Society for Information Science, 42(5), 361-372. https://doi.org/10.1002/(SICI)1097-4571(199106)42:5<361::AID-ASI6>3.0.CO;2-%23
- McLeod, C. (2020). Trust. In The Stanford Encyclopedia of Philosophy. https://plato.stanford.edu/archives/fall2020/entries/trust/ (Internet Archive)
- Nass, C., & Moon, Y. (2000). Machines and mindlessness: Social responses to computers. Journal of Social Issues, 56(1), 81-103. https://doi.org/10.1111/0022-4537.00153
- Nickel, P. J., Franssen, M., & Kroes, P. (2010). Can we make sense of the notion of trustworthy technology? Knowledge, Technology & Policy, 23(3-4), 429-444. https://doi.org/10.1007/s12130-010-9124-6
- O’Neill, O. (2002). Lecture 4: Trust and transparency. A question of trust: The BBC reith lectures 2002. Cambridge University Press.
- Pan, P., Hembrooke, H., Joachims, T., Lorigo, L., Gay, G., & Granka, L. (2007). In Google we trust: User’s decisions on rank, position, and relevance. Journal of Computer-Mediated Communication, 12, 801-823. https://doi.org/10.1111/j.1083-6101.2007.00351.x
- Ridley, M. (2019). The autonomous turn in information behaviour. In Proceedings of ISIC, The Information Behaviour Conference, Krakow, Poland, 9-11 October: Part 2. Information Research, 24(1), paper isic1832. http://InformationR.net/ir/24-1/isic2018/isic1832.html (Internet Archive)
- Ridley, M., & Pawlick-Potts, D. (2021). Algorithmic literacy and the role for libraries. Information technology and libraries, 40(2). https://doi.org/10.6017/ital.v40i2.12963
- Ryan, M. (2020). In AI we trust: ethics, artificial intelligence, and reliability. Science and Engineering Ethics, 26(5), 2749-2767. https://doi.org/10.1007/s11948-020-00228-y
- Savolainen, R. (2018). Pioneering models for information interaction in the context of information seeking and retrieval. Journal of Documentation, 74(5), 966-986. https://doi.org/10.1108/JD-11-2017-0154
- Smith, B. C. (2019). The promise of artificial intelligence: reckoning and judgment. MIT Press.
- Sundin, O., & Carlsson, H. (2016). Outsourcing trust to the information infrastructure in schools: how search engines order knowledge in education practices. Journal of Documentation, 72(6), 990-1007. https://doi.org/10.1108/JD-12-2015-0148
- Sundin, O., Haider, J., Andersson, C., Carlsson, H., & Kjellberg, S. (2017). The search-ification of everyday life and the mundane-ification of search. Journal of Documentation. 73(2), 224-243. https://doi.org/10.1108/JD-06-2016-0081
- Taylor, R. S. (1968). Question-negotiation and information seeking in libraries. College and Research Libraries, 29, 178-194. https://doi.org/10.5860/crl.76.3.251
- Wilson, T. D. (1999). Models in information behaviour research. Journal of documentation, 55(3), 249-270. https://doi.org/10.1108/EUM0000000007145