Adoption of open government data: perspectives of user innovators
Hui-Ju Wang
Introduction. Open government data has drawn increasing attention from both governments and academic researchers around the world in recent years. Innovation on the basis of such data can be termed data-driven innovation, and it has been considered a critical mechanism that can create social and economic value. Understanding the driving factors for the adoption of these data among user innovators has become a significant issue for associated initiatives. Accordingly, this study examines the factors influencing the adoption of open government data from the perspective of individual user innovators to fill the existing knowledge gap.
Method. Based on the social cognitive theory, this study developed a research model that integrates the following factors that are central to individuals' adoption decisions: computer self-efficacy, tool experience, government support, and social influence. This study examined this model by using survey data from individual users with experience in the adoption of open government data for innovation in Taiwan.
Analysis. The study utilised structural equation modelling to examine the research framework and hypotheses.
Results. The results show a significant positive relationship among computer self-efficacy, social influence, and adoption of open government data.
Conclusions. This study serves as a first attempt to advance knowledge on the adoption of open government data from the perspectives of individual user innovators by empirically testing a research model that integrates factors within personal and environmental contexts. This study contributes by offering significant theoretical and managerial implications for researchers of open government data and government policy practitioners.
Introduction
Open government data makes public sector information freely available in open formats that allow the public to access and exploit it (Kalampokis et al., 2011). Governments and academic researchers around the world have been paying a great deal of attention to the issues surrounding open government data initiatives over the past several years. Nevertheless, few studies have explored the issues related to the adoption of open government data from the perspective of user innovators.
Individual users or individual user firms that develop new products and services for themselves without assistance from or the involvement of the producers have been referred to as user innovators (von Hippel, 1988). An early research stream showed that users play an important but peripheral role by providing producers with some of the critical inputs needed to develop and market products that better meet customer needs (e.g., Burns and Stalker, 1961; Myers and Marquis, 1969; Rothwell, 1977). Subsequent studies further argued that users can be innovators too, not just helpers to producers who innovate (Hyysalo, 2009; von Hippel, 1988, 2005). An increasing number of studies has focused on the determinants of user innovation (Bin, 2013; Nambisan et al., 1999), the measurement of user innovation (Flowers et al., 2010), and its impact on industrial development (Hyysalo, 2009). Nonetheless, most of these studies have been conducted in the context of the sports industry (Franke et al., 2006; Hienerth, 2006; Hyysalo, 2009; Lüthje, 2004; Lüthje et al., 2005). The understanding of user innovators in the context of open government data has drawn less attention from researchers (e.g., Susha et al., 2015)
Innovation on the basis of open government data can be termed data-driven innovation (Susha et al., 2015). Data-driven innovation has been seen as a critical mechanism that can create economic value through the generation of new knowledge, processes, services, products and businesses (e.g., Carrara et al., 2015; Jetzek et al., 2014). The governments of various countries have encouraged the public to use open government data to develop new commercial products and services in the last few years, such as the US (Virginia Employment Commission, 2016), Canada, Singapore, and Taiwan. It is crucial for governments to understand the perspectives of individual user innovators on open government data adoption because it can illuminate the dynamics of using open government data for innovation.
Past studies have concentrated on the driving factors of open government data adoption; however, their efforts have been limited to the perspectives of government agencies (Wang and Lo, 2016) and entrepreneurs (Susha et al., 2015) rather than user innovators. Accordingly, this study attempts to conduct a systematic analysis of the factors that influence the adoption of open government data among individual user innovators based on the social cognitive theory (Bandura, 1986). The social cognitive theory is one of the most powerful theories for explaining human behaviour and has been employed in various contexts of information technology adoption, such as an e-government system (Loo et al., 2009; Rana and Dwivedi, 2015; Sahu and Gupta, 2007), Internet uses (LaRose and Eastin, 2004), and computer systems (e.g., Compeau and Higgins, 1995; Loo et al., 2009; Venkatesh et al., 2003). Therefore, this study proposes a research model that integrates the framework of social cognitive theory and factors that have been extensively used in technology adoption studies to explain the determinants of open government data adoption decisions among user innovators at the individual level, including computer self-efficacy, tool experience, government support, and social influence.
This study contributes to the literature in several ways. First, this study presents a new perspective (i.e., social cognitive theory) to identify significant determinants of open government data adoption. Second, this study serves as an initial attempt to advance knowledge on open government data adoption from the perspectives of individual user innovators by empirically testing a research model that integrates factors within personal and environmental contexts. Finally, the managerial implications of this study will benefit policy practitioners and researchers of open government data.
This study is organized as follows: The study first reviews the relevant literature on the adoption of open government data and user innovators, theoretical perspectives on its adoption, and the social cognitive theory. This is followed by hypotheses development and the research methodology. Thereafter, this study reveals the research results and discusses the findings and the implications of the research. Finally, the study concludes with contributions, limitations, and directions for future research.
Literature review
Open government data vs. user innovators
Several studies concerning open government data have concentrated on topics such as strategic frameworks for open government data initiatives (Jetzek et al., 2012; Meijer and Thaens, 2009) and data quality measurement (Vetrò et al., 2016), benefits and barriers (Janssen et al., 2012; Zuiderwijk et al., 2012), and determinants of open government data adoption (Susha et al., 2015; Wang and Lo, 2016). Among these studies, open government data adoption of user innovators has not drawn attention from researchers.
There is a growing body of research showing that users play a much more active role in processes of innovation than has been generally believed. This has sparked various research issues around user innovation, including how to measure it (Flowers et al., 2010) and its determinants and impacts (Bin, 2013; Hyysalo, 2009; Nambisan et al., 1999). Recently, the research on users as innovators has been extended from the contexts of the chemical industry (Freeman, 1968), sports industry (Franke et al., 2006; Hienerth, 2006; Hyysalo, 2009; Lüthje, 2004; Lüthje et al., 2005), library industry (Morrison et al., 2000), and high-tech industry (de Jong and von Hippel, 2009) to the context of open government data (Susha et al., 2015).
According to Jetzek et al. (2013), innovation refers to the implementation of a new or significantly improved product (goods or services) or process, a new marketing method or a new organizational method in business practices' workplace organization or external relations. Innovation that is largely based on the use of open government data has been termed data-driven innovation (Jetzek et al., 2014; Susha et al., 2015). Based on the perspective of the data value chain (Carrara et al., 2015), user innovators play an important role in creating value for open government data.
The data value chain can serve as a basis for understanding different types of reuse for open government data. The value chain underlying data-driven innovation has been generally described as follows: suppliers (government organizations) publish data, aggregators combine the data to produce insights, developers build apps, enrichers enhance the existing products with the data, and enablers facilitate the supply and use of data (Carrara et al., 2015; Hammell et al., 2012; Susha et al., 2015). Through the development of new or improved services using open data, innovation can occur and take the form of single purpose or interactive apps, information aggregators, comparison models, open data repositories, or service platforms (Janssen and Zuiderwijk, 2014). The data value chain shows the potential value that can be reached by making open data available for user innovators to reuse. Innovation using open government data has been expected to generate positive effects (Carrara et al., 2015; Susha et al., 2015), including more competition, further innovation and use of open data, increased public engagement, more trust in government, better efficiency of public services, and economy-wide effects (e.g., the transformation of markets and industries).
From the perspective of the data value chain, open government data adoption by user innovators is significant for policy initiatives of open government data because it serves as a driver of innovation, which can bring further social and economic value. Past studies have explored impediments to open government data use (Huijboom and Van den Broek, 2011; Janssen et al., 2012; Zuiderwijk et al., 2012), predictors of open government data adoption (Zuiderwijk et al., 2015), driving factors of open government data adoption (Susha et al., 2015; Wang and Lo, 2016), and positive impacts of open government data adoption (Janssen et al., 2012; Jetzek et al., 2012), while neglecting the perspectives of user innovators on open government data adoption. Accordingly, in exploring the determinants of open government data adoption, diverting the context from government agencies (Wang and Lo, 2016) and businesses (Susha et al., 2015) to user innovators is critical for advancing knowledge of open government data.
Theoretical perspectives on adoption of open government data
All ideas, products, methods, or process that are viewed as new or improved by an individual or other unit of adoption are called innovations (Jetzek et al. 2013; Rogers, 1995). Open government data involves not only data-driven innovation but also a technological innovation as they are government services delivered by new information technology applications. Several popular theoretical models have been widely used to study individuals' use behaviour in the context of innovation, such as the theory of reasoned action (Fishbein and Ajzen, 1975), theory of planned behaviour (Ajzen, 1991), technology acceptance model (Davis, 1989), and the unified theory of acceptance and use of technology (Venkatesh et al., 2003). Nevertheless, for the following reasons, this study does not use any of these models to develop research frameworks for the adoption of open government data among individual user innovators.
First, these models have been employed in a wide range of technology innovation studies, providing explanations of individual behaviour. However, simply applying one of the models may be inappropriate and may fall short of more accurately explaining innovation behaviour with multiple characteristics (Song, 2014). In this study, open government data adoption of user innovators involves multiple characteristics of new products or services; thus, it is expected to be associated with a set of adoption drivers that are different from those identified by these models for traditional innovation adoption.
Second, several researchers have suggested that any borrowed theory must be refined to fit a given setting, thus researchers can specify models and variables that match their specific research purposes. Social cognitive theory (Bandura, 1986) is a generic theory that allows researchers to combine various sources of influence instead of specifying variables. It can be applied in the 'specific contexts' where individual user innovators' adoption behaviour occurs, offering a useful base for examining the open government data adoption of user innovators at the individual level. Based on the above arguments, this study utilises the social cognitive theory to guide a systematic analysis of factors that influence open government data adoption among individual user innovators.
Social cognitive theory
Social cognitive theory is one of the most powerful theories of human behaviour (Bandura, 1986). According to social cognitive theory, an individual's behaviour is regulated by the person through the cognitive processes and by the environment through external social situations (Bandura, 1986; Cooper and Lu, 2016). The three factors interact with each other to influence an individual's behaviour.
In terms of personal factors, an individual's perception, beliefs, expectations, feelings, knowledge, abilities, and skills affect their behaviour (Bandura, 1986, 1989; Benight and Bandura, 2004; Prussia and Kinicki, 1996). In addition, Bandura (1989) also noted that an individual's environment, that is, the factors external to the individual, influences the person's behaviour. This environment contains the physical and social environment; the former involves the natural and manmade objects within an individual's surroundings, and the latter includes the immediate physical surroundings, virtual world, social relationships, social norms, and cultural milieus within which defined groups of people function and interact (Bandura, 1991; Narayan, 2013). The final component of the social cognitive theory is behaviour, which is the way in which individuals act or respond to a particular situation or object and involves how individuals respond to technology or technological innovations Bandura, 1991; LaRose and Eastin, 2004; Ratten and Ratten, 2007).
Social cognitive theory has been employed in various research contexts, such as the adoption of an e-government system (Loo et al., 2009; Rana and Dwivedi, 2015; Sahu and Gupta, 2007), task complexity (Bolt et al., 2001), organizational management (Wood and Bandura, 1989), technological innovation adoption (Compeau, et al., 1999; Ratten and Ratten, 2007), tourism sustainability (Font et al., 2016), Internet uses and gratifications (LaRose and Eastin, 2004), and use intention toward computer systems (e.g., Compeau and Higgins, 1995; Loo et al., 2009; Venkatesh et al., 2003). The social cognitive theory has been successfully utilised to explain individual behaviour in various contexts of organizational management and technological adoption. Accordingly, it is appropriate to employ the social cognitive theory to explore adoption behaviour of open government data among individual user innovators.
Research model
Based on social cognitive theory, a model tailored for open government data adoption among individual user innovators is developed and depicted in Figure 1. The model reveals two major contexts of open government data adoption (i.e., personal and environmental contexts) integrating four factors: computer self-efficacy, tool experience, government support, and social influence. The framework is discussed briefly below.
Personal context
Computer self-efficacy
Originating from social cognitive theory (Bandura, 1986), self-efficacy is defined as individuals' assessments of their effectiveness or competency to perform a specific behaviour successfully. It involves individuals' beliefs about their ability to successfully achieve goals and manage environments that affect their lives (Bandura, 1989). Self-efficacy perceptions have been a crucial determinant of behaviour and have received increasing attention in the literature concerning issues in managerial and information technology settings, such as career choice and development (Betz and Hackett, 1981; Jones, 1986), sales performance (Barling and Beattie, 1983), specific technologies and innovations (White et al., 2008; Youn, 2009), and technological usage behaviour (Agarwal et al., 2000; Compeau and Higgins, 1995; Durndell and Haag, 2002; Teoh et al., 013; Thatcher and Perrewe, 2002; Venkatesh, 2000; Venkatesh and Davis, 1996). Among these studies, computer self-efficacy has been widely used in the field of information systems and is significant in influencing individual perceptions and behaviour toward diverse technologies.
Computer self-efficacy refers to individuals' beliefs about their ability to successfully use computers to solve tasks and manage situations (Compeau and Higgins, 1995; Marakas et al., 1998). In previous studies, many researchers have found the positive effects of computer self-efficacy on users' technology adoption perceptions, such as technological ease of use (Venkatesh, 2000; Venkatesh and Davis, 1996), attitude (Dillon and Lending, 2010; Durndell and Haag, 2002; Sam et al., 2005), and behaviour (Hill et al., 1987). However, they have found negative relationships between computer self-efficacy and computer anxiety (Durndell and Haag, 2002). In past studies, computer self-efficacy has been the focus of various technological issues, such as integrated clinical information systems (Dillon et al., 2003), hospital information systems (Dillon and Lending, 2010), healthcare technology (Rahman et al., 2016), Internet technology (Durndell and Haag, 2002), computer technology (Compeau and Higgins, 1995; Hill et al., 1987), and information technology (Venkatesh et al., 2003). Based on the above discussion, this study suggests that computer self-efficacy is influential in specific technology adoption. Thus, in the context of open government data, the study proposes the following hypothesis:
H1: Computer self-efficacy will have a positive effect on open government data adoption among individual user innovators.
Tool experience
An individual's experience with a given item in the past has a decisive impact on their effort expectations (Venkatesh et al., 2003) and behaviour toward that item (Fishbein and Ajzen, 1975). These issues have been explored in various technological contexts, such as a mobile payment system (Liébana-Cabanillas et al., 2014), a computer system (O'Cass and Fenech, 2003; Smith and Brynjolfson, 2001), and e-commerce (Dholakia and Uusitalo, 2002; Hsu et al., 2007; Kwak et al., 2002; Liao and Cheung, 2001; Miyazaki and Fernandez, 2001). User's perceptions of the use of these specific technologies have been referred to as tool experiences (Sun, 2016).
Tool experience, representing individual abilities, has been included in the task-technology fit model as a direct effect on information technology utilisation (Dishaw and Strong, 1998). Some researchers have also explored the impacts of tool experience on perceived ease of use, perceived usefulness, attitudes towards the tool, intention to use the tool, and tool readiness. For instance, Dishaw and Strong (1999) propose a model that integrates the technology acceptance and task-technology fit models to examine individuals' choices about using information technology. They found a direct influence of tool experience on perceived ease of use and perceived usefulness and an indirect effect of tool experience on the attitude towards use. Additionally, Sun (2016) explored the relationship between tool experience and tool readiness from the perspective of a human activity readiness theory. Based on previous studies, tool experience is expected to influence individuals' use of open government data. Therefore, the hypothesis is proposed below:
H2: Tool experience will have a positive effect on open government data adoption among individual user innovators.
Environmental context
Government support
Government support has been influential in technology adoption in previous studies (Gibbs and Kramer, 2004; Teo and Ranganathan, 2004). Several researchers suggest that the government can create a favourable environment and provide impetus for technology adoption (Gibbs and Kramer, 2004; Kurnia et al., 2015; Tan and Ouyang, 2006). Specifically, government regulations can encourage or discourage the adoption of innovations (Lin and Ho, 2009). Governments in various countries have initiated user innovation of open government data over the past several years. For instance, the Industrial Development Bureau in Ministry of Economic Affairs in Taiwan initiated the Open Data Application Promotion Plan in 2013, which has promoted the Open Data Contest for Innovative Applications for several years to encourage enterprises and individuals to develop innovative services or products by reusing open government data. More recently, the governor of Virginia in the United States launched the Open Data, Open Jobs initiative to provide an open data set of available jobs in Virginia in 2016, allowing entrepreneurs to use the data to create apps and programs (Governor McAuliffe's Office, 2016). In this study, government support is expected to be the driver of open government data adoption among individual user innovators; therefore, the hypothesis is proposed below:
H3: Government support will have a positive effect on open government data adoption among individual user innovators.
Social influence
Social influence refers to the degree to which the actions, reactions, and thoughts of an individual are influenced by other people or groups (Ajzen and Fishbein, 1980; Fishbein and Ajzen, 1975). The significance of social influence in affecting individuals' perceptions and behaviour has been identified in past studies (e.g., Venkatesh, 2000; Warshaw, 1980).
There have been various concepts that are similar to social influence. Social influence as a direct determinant of behavioural intention is represented as a subjective norm in the theory of reasoned action and the theory of planned behaviour, social factors in a model of personal computer utilisation, and an image in innovation diffusion theory (Venkatesh et al., 2003). Comparing these models, Venkatesh et al. (2003) found that the social influence constructs listed above behave similarly and suggest that each of these constructs encompasses the explicit or implicit notion that the individual's behaviour is influenced by the way in which they believe others will view them as a result of having used the technology. The role of social influence in information technology acceptance decisions has been identified in diverse technological settings, such as mobile government Liu et al., 2014), social networking sites (Ifinedo, 2016), building information modelling (Howard et al., 2017), information technology systems (Venkatesh et al., 2003), and e-commerce systems (Hwang, 2010). Based on the above findings, the impacts of social influence on open government data adoption are expected in this study. Thus, the following hypothesis is proposed:
H4: Social influence will have a positive effect on open government data adoption among individual user innovators.
Based on those hypothesized relationships, the research model is presented in Figure 1.
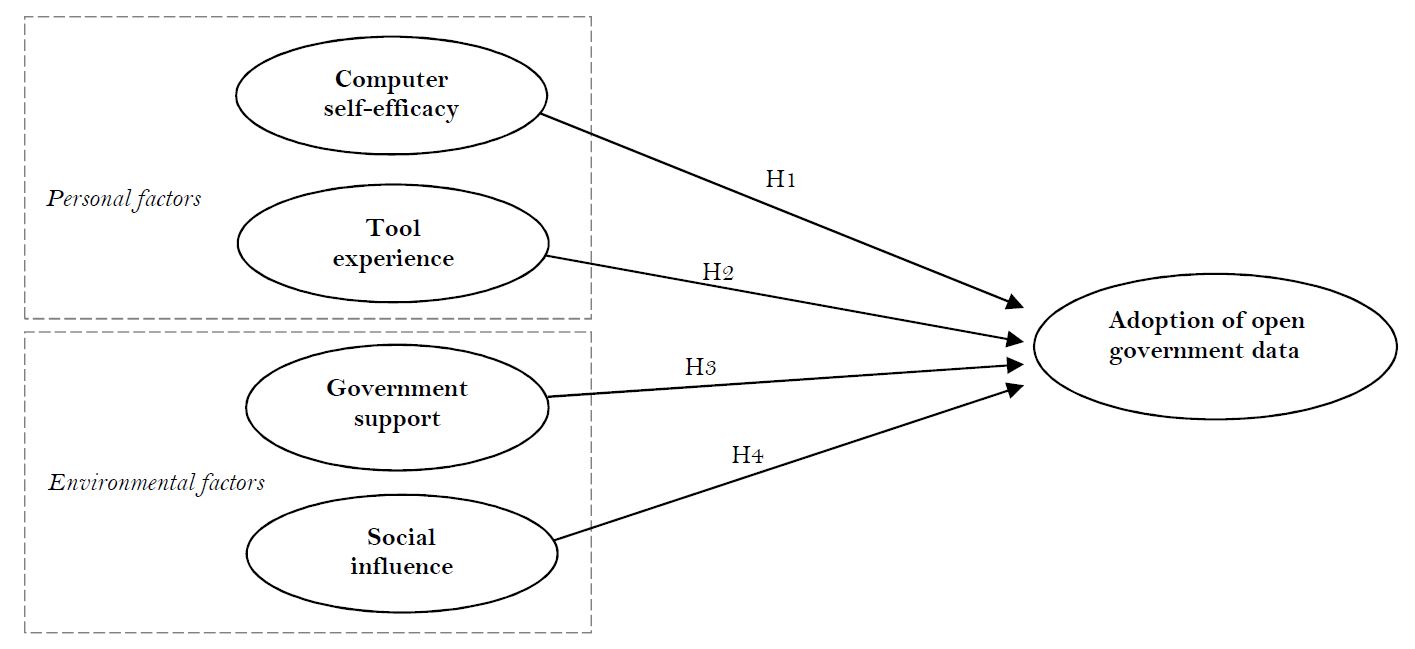
Figure 1: Proposed model for evaluating the adoption of open government data by individual user innovators
Research methods
Sample
The sample studied in this study is composed of individuals who have experience with open government data adoption for innovation in Taiwan. The study collected sample data from multiple sources in two stages. At the first stage, the study chose face-to-face venues to issue questionnaires. The Open Data Contest for Innovative Applications is held by the Taiwanese government to encourage innovative applications that adopt. Therefore, the study invited the contestants to complete the questionnaires in the competition venue. The study allowed the participants to return the questionnaires online or by offline methods. The study received a total of fifty-nine responses offline and twenty-one responses online.
Because the Contest participants are limited in number, the study enlarged the sample size through other sources. At the second stage, the study collected a list of the 193 apps that have used open government data through the App Store on a mobile phone. The developers were invited by e-mail to join the survey. Forty-five online questionnaires were returned. The survey was conducted from May 2017 to December 2017. Deleting two repeat questionnaires, the study received 123 valid responses in total.
Of the respondents, thirty-three (26.8%) were female and ninety (73.2%) were male. The respondents ranged from seventeen to sixty-two years, with an average of 24.6 years, and majority of the respondents were between the ages of twenty-five and thirty-four (44.7%). The occupations of the respondents are variable, including information technology-related positions (47.2%), students (22.8%), project specialists (8.9%), managers (8.1%), administration members (8.1%), leaders (i.e., Chief Technical Officers and Chief Executive Officers) (1.6%), researchers (1.6%), and others (1.6%). A majority of the respondents have more than sixteen years of Internet usage experience (52.8%) and more than ten hours of Internet access per day (34.1%). In addition, each respondent used open government data platforms 7.7 times per month on average.
Measurement items
The questionnaire items in the proposed model were mostly adapted from previous studies and were pre-tested by having several professors review them to ensure the validity and reliability of the scales. Before the items were distributed, they were carefully assessed and revised for content validity and clarity. The study initially used English to develop the questionnaire items, which were subsequently translated into Chinese for the respondents. The study employed the back-translation technique to ensure linguistic equivalence between the English and Chinese versions (cf. Bhalla and Lin, 1987).
The measurement of the questionnaire items used a Likert-type five-point response scale that ranged from not at all (1) to very strongly (5). This study proposes five constructs; computer self-efficacy, tool experience, government support, social influence, and adoption of open government data, and referred to previous related studies to develop the definitions and measurements of these constructs.
Computer self-efficacy
In this study, computer self-efficacy is defined as 'a perception of his or her ability to use open government data in the accomplishment of an innovation task' (Compeau and Higgins, 1995). The measurement of computer self-efficacy includes five items (X1-X5): I would feel confident using platforms of open government data if no one is around to tell me what to do as I proceed (X1); I would feel confident using platforms of open government data if I had seen someone else using them before trying them myself (X2); I would feel confident using platforms of open government data if I had sufficient time to practice (X3); I would feel confident using platforms of open government data if I had read the manuals (X4); and I would feel confident using platforms of open government data if I had participated in training courses (X5). These items were adapted from Compeau and Higgins.
Tool experience
This study refers to Dishaw and Strong (1999), defining tool experience as 'an individual's experience level, prior level of use, and prior hours of use toward IT tools used'. The study employs three items for measuring tool experience (X6-X8), which were adapted from Dishaw and Strong: I often use the Internet (X6); I often use open government data (X7); and I have been using IT facilities for a long time (e.g., computer, Internet, and smart phone) (X8).
Government support
The study defines government support as, whether the government understands the nature and functions of open government data and therefore fully supports its development. The study adapted the measurements of Lian et al. (2014) to measure government support, including three items (X9-X11): open government data development is becoming one of the government’s major policies (X9); the government has planned to develop open government data (X10); and the government encourages firms to adopt open government data (X11).
Social influence
Social influence in this study is defined as 'the degree to which the open government data platform use of an individual is influenced by other people who are important to him/her' (Ajzen and Fishbein 1980). The study adapted the measurements from Venkatesh et al. (2003) to measure social influence, including five items (X12-X16): most of my colleagues think that open government data should be used for our job (X12); my supervisor thinks that I should use open government data for my job (X13); my supervisor encourages me to use open government data for my job (X14); my supervisor asks me to use open government data for my job (X15); people around me expect me to use open government data for tasks (X16).
Adoption of open government data
Three items were developed to measure open government data adoption (Y1-Y3): I often review the variety of open government data that can be increased (Y1); I often use the functions provided by open government data platforms (Y2); and I often download the data set from open government data platforms (Y3).
Analysis and results
The study used structural equation modelling to examine the research framework and hypotheses (Jöreskog and Sörbom, 1993). Although there is no consensus regarding the appropriate sample size for structural equation modelling, recommendations range between 100 and 200 (Hair et al., 2009). Additionally, ‘the sample size should also be large enough when compared with the number of estimated parameters (as a rule of thumb at least 5 times the number of parameters)…’ (Reisinger and Turner, 1999, pp. 78–79). Accordingly, the sample size in this study is adequate for model testing. The study presents the results of the measurement model, structural model, and hypothesis testing in this section.
Measurement model
Referring to Anderson and Gerbing (1988), the study examined the hypotheses through a two-step procedure. First, the study conducted a confirmatory factor analysis of the measurement model to estimate if the measurement items were appropriate to represent each construct. Secondly, the study examined the structural model after a satisfactory fit in the measurement model was found.
The study used the method of maximum likelihood estimation for the confirmatory factor anal as the normality assumption was met. The results suggested a good fit for the measurement model [χ2 /df = 1.29 (x2(94) = 121.49, P = 0.03), a goodness of fit index = 0.89, a normed fit index = 0.95, a comparative fit index = 0.98, and a root mean square error of approximation = 0.049 and a high degree of scale reliability and convergent validity (after the removal of some measures, including X7, X15, and Y3). This study examined the reliability and validity of all the measures via the items’ loadings, the composite reliabilities, and average variance extracted.
As indicated in Table 1, the loadings of all constructs are significant. The results of the composite reliabilities and average variance extracted exceed the cutoff values suggested by Hair et al. (2010). According to Hair et al., composite reliabilities values between 0.6 and 0.7 are acceptable, and 0.7 or higher suggests a good result. An average variance extracted value of 0.5 or higher suggests adequate convergent validity. These findings indicate the adequate internal consistency among multiple items for each construct as well as a large portion of the variance explained by the constructs. Moreover, the study compared the average variance extracted with the squared correlation among the constructs to test discriminant validity (Fornell and Larcker, 1981). The results reveal that the average variance extracted was greater than the squared correlations between any pair of constructs, suggesting discriminant validity among the constructs.
The study employed procedural remedies and statistical remedies to reduce the errors associated with common method variance (Podsakoff et al., 2003). With regard to procedural remedies, the study collected the data from various sources. Moreover, this study adopted Harman’s single factor for the statistical remedies. An exploratory factor analysis was conducted on all items, identifying the number of factors that are essential to explain the variance in the items via the factor analysis of unrotated principal components. No common method variance problem is found in this study based on the following results. First, multiple factors with eigenvalues greater than 1.0 rather than a single factor account for 70.76% of the total variance. Secondly, the first factor accounts for 43.14% of the variance, lower than the 50% threshold (Podsakoff et al., 2003).
Constructs | Items | Loadings | Composite reliabilities | Average variance extracted |
---|---|---|---|---|
Adoption of open government data | Y1 | 0.85*** | 0.89 | 0.80 |
Y2 | 0.93*** | |||
Computer self-efficacy | X1 | 0.75*** | 0.90 | 0.65 |
X2 | 0.86*** | |||
X3 | 0.83*** | |||
X4 | 0.84*** | |||
X5 | 0.75*** | |||
Tool experience | X6 | 0.86*** | 0.86 | 0.76 |
X8 | 0.88*** | |||
Government support | X9 | 0.85*** | 0.85 | 0.66 |
X10 | 0.87*** | |||
X11 | 0.71*** | |||
Social influence | X12 | 0.70*** | 0.88 | 0.65 |
X13 | 0.92*** | |||
X14 | 0.87*** | |||
X16 | 0.71*** | |||
***p<0.001 |
Structural model and hypothesis testing
The study used the maximum likelihood estimation to analyse the structural model. The results suggest a good fit for the model with chi-square statistics χ2 /df = 1.29 (x2(94) = 121.49, P = 0.03, a goodness of fit index=0.89, a normed fit index=0.95, a comparative fit index=0.98, and a root mean square error of approximation=0.049. The value for the adoption of open government data is acceptable (R2AD = 0.46).
Two path coefficients in the integrated model are significant. As indicated in Table 2, computer self-efficacy and social influence positively affect the adoption of open government data based on standardised path coefficients (0.26 and 0.38, respectively), while tool experience and government support are not significantly influential in the adoption of open government data. Accordingly, the results only support the relationships in H1 and H4.
Paths | Hypotheses | Path coefficients | t-value |
---|---|---|---|
Computer self-efficacy→Adoption of open government data | H1: supported | 0.26* | 2.01 |
Tool experience→Adoption of open government data | H2: not supported | 0.13 | 1.24 |
Government support→Adoption of open government data | H3: not supported | 0.10 | 1.11 |
Social influence→Adoption of open government data | H4: supported | 0.38*** | 3.35 |
*p<0.05, ***p<0.001 |
Discussion
Discussion of findings
This study has confirmed two determinants of open government data adoption among individual user innovators: computer self-efficacy and social influence. This result suggests that the personal and environmental factors of the social cognitive theory are suitable for explaining the open government data adoption of individual user innovators.
The results of this study clearly support the idea that social influence has the highest degree of influence on the adoption of open government data. The findings on the significance of social influence are consistent with those of previous studies with regard to information technology adoption decisions in the settings of social networking sites (Ifinedo, 2016), mobile government (Liu et al., 2014), information technology systems (Venkatesh et al., 2003), e-commerce systems (Hwang, 2010), and building information modelling (Howard et al., 2017). Accordingly, this study provides further support for the importance of social influence in understanding technology adoption by extending the knowledge from these settings to open government data settings.
In addition, the finding that computer self-efficacy has positive effects on open government data adoption is consistent with our hypothesis. This finding supports the results of the innovation literature that computer self-efficacy is a driver of technology adoption behaviour (e.g., Hill et al., 1987). However, the results on the significance of computer self-efficacy are not in line with the findings of Venkatesh et al. (2003), which revealed a nonsignificant relationship between computer self-efficacy and behaviour intention due to the effect being captured by effort expectancy. The study also complements the significance of computer self-efficacy in technology adoption by extending the technological focus from health-care systems (Dillon and Lending, 2010; Dillon et al., 2003; Rahman et al., 2016) and computer-related technologies (Compeau and Higgins, 1995; Hill et al., 1987; Venkatesh et al., 2003) to open government data.
Surprisingly, tool experience and government support were not found to be significant determinants. First, the significant effects of tool experience on the adoption of open government data were not observed. This result is inconsistent with those of previous studies with regard to information technology use (Dishaw and Strong, 1998), attitudes towards information technology use (Dishaw and Strong, 1999), and tool readiness (Sun, 2016). The insignificant relationship between tool experience and open government data adoption found in this study may be explained by the following. Based on the profile of the respondents in this study, the roles of the respondents are diverse, including information technology-related positions, managers, project specialists, administration, researchers, students, etc. It is obvious that a background in information technology is not the sole feature of the user innovators surveyed, which may demonstrate that tool experience is not the main factor that influences individual user innovator open government data adoption decisions.
Secondly, while the significance of government support is supported by previous studies on innovative adoption (e.g., Teo and Ranganathan, 2004), a significant relationship between government support and open government data adoption was not found in this study. The possible explanations are as follows: the Taiwanese government has been implementing the Open Data Application Promotion Plan since 2013, encompassing diverse plans such as open government data contests and subsidies for open government data use of enterprises. These initiatives are still in the early stages; specifically, Open Data Contest for Innovative Applications was not held until 2014, which results in most of the user innovators not having been informed of the government’s plans related to initiatives of open government data. This reason may account for the insignificant effects of government support on open government data adoption among individual user innovators in this study.
Theoretical implications
This study first contributes to the research literature regarding determinants of open government data adoption from the perspectives of individual user innovators. While previous studies have identified the benefits, barriers, and drivers of open government data adoption according to public servants, none have presented an integrated model for systematically examining the determinants of open government data adoption among user innovators at the individual level, specifically in terms of the social cognitive theory perspective. This study serves as a first attempt to advance knowledge on open government data adoption by empirically testing a research model that integrates the social cognitive theory, computer self-efficacy, tool experience, government support, and social influence. This study identified the effects of both personal and environmental factors, i.e., computer self-efficacy and social influence, on open government data adoption. These factors were explored to understand the determinants of technological innovation adoption, and they effectively explain the factors that influence adoption decisions of open government data by individual user innovators.
Secondly, the results of this study complement and extend innovation adoption studies that have refined and validated the social cognitive theory for various technical issues, such as the adoption of an e-government system (Loo et al., 2009; Rana and Dwivedi, 2015; Sahu and Gupta, 2007), technological innovation adoption (Compeau et al., 1999; Ratten and Ratten, 2007), Internet uses and gratifications (LaRose and Eastin, 2004), and use intention toward computer systems (e.g., Compeau and Higgins, 1995; Loo et al., 2009; Venkatesh et al., 2003), by applying the social cognitive theory perspective to the adoption of open government data.
Managerial implications
This study makes a practical contribution by identifying the determinants of open government data adoption among individual user innovators. The findings may help policy practitioners of open government data develop more effective strategies that increase user innovators’ adoption motivations at the individual level.
This study first concludes that policy practitioners should focus on social influence, which is a major factor in individual user innovators’ adoption of open government data. The study advises policy practitioners to place more emphasis on encouraging open government data adoption of individual user innovators by posting information related to contests or workshops of open government data adoption for innovation on social networking sites such as Facebook, Twitter, and Instagram. In these venues, social influence on members’ perceptions and choice behaviour toward new products has been recognized by marketing researchers (e.g., Dholakia and Bagozzi, 2001). Accordingly, they serve as a good candidate for policy practitioners to promote open government data adoption of user innovators at the individual level. Additionally, policy practitioners may need to pay attention to computer self-efficacy among individual user innovators when promoting open government data policy. Policy practitioners are advised to encourage user innovator acceptance of open government data by offering them manuals and training courses about how to use open government data platforms and adopting it to develop new services or products. For example, the government can put more effort into encouraging individual user innovators adopt open government data for innovation via subsidies of computer training courses and choosing the best practices of open government data adoption among individual user innovators. By doing so, their perceptions toward their ability and confidence in using open government data in completing an innovation task can be enhanced and can further influence their adoption behaviour.
Conclusion
This study has revealed two factors that influence the adoption of open government data by individual user innovators, namely social influence and computer self-efficacy. Specifically, social influence may be more important than other factors related to the adoption of open government data. This study may have significant contributions to academic researchers and practitioners involved in developing policy related to open government data. The study suggests that policy makers consider the two significant determinants of open government data adoption among individual user innovators based on their relative importance while devising strategic plans for open government data initiatives.
This study has several limitations. First, because the initiative related to open government data use in Taiwan is still in its infancy, few contests or workshops related to innovative application of open government data have been held for user innovators, which created a major challenge for the study with regard to collecting sample data and reaching possible respondents. Accordingly, the limited sample size of this study may affect the generalisability of our findings to all user innovators who have experience in open government data adoption. Future researchers could attempt to collect a larger sample size via other sources to better examine the factors affecting individual user innovators’ adoption of open government data.
Moreover, the adoption of open government data can also be examined from the perspective of different interested parties. It is the Taiwanese government’s policy to provide open government data for use of government, businesses, and individuals. The study extends the research that has been conducted in the context of open government data adoption in government agencies to the context of user innovators. Future studies can explore whether the factors influencing the adoption of open government data among individual user innovators in this study can be generalised to the contexts of end-users (i.e., individuals without innovation abilities). These issues may offer comprehensive insights into the driving factors of open government data adoption.
Acknowledgements
The study was supported by a grant from the Ministry of Science and Technology (MOST 105-2410-H-431-009-), Taiwan.
About the author
Hui-Ju Wang is an Assistant Professor in the Bachelor Degree Program in Ocean Business Management at National Taiwan Ocean University, Taiwan. Her research interests include marketing management, brand management, consumer behaviour, open government data, and network analysis. She can be contacted at: hjwang@email.ntou.edu.tw.
References
- Agarwal, R., Sambamurthy, V., & Stair, R. M. (2000). Research report: the evolving relationship between general and specific computer self-efficacy - an empirical assessment. Information Systems Research, 11(4), 418–430. https://doi.org/10.1287/isre.11.4.418.11876
- Ajzen, I. (1991). The theory of planned behavior. Organizational Behaviour and Human Decision Processes, 50(2), 179–211. https://doi.org/10.1016/0749-5978(91)90020-T
- Ajzen, I., & Fishbein, M. (1980). Understanding attitudes and predicting social behavior. Prentice-Hall.
- Anderson, J. C., & Gerbing, D. W. (1988). Structural equation modeling in practice: a review and recommended two-step approach. Psychological Bulletin, 103(3), 411–423.
- Bandura, A. (1986). Social foundations of thought and action: a social cognitive theory. Prentice-Hall.
- Bandura, A. (1989). Human agency in social cognitive theory. The American Psychologist, 44(9), 1175–1184. https://doi.org/10.1037/0003-066X.44.9.1175
- Bandura, A. (1991). Social cognitive theory of moral thought and action. In W. M. Kurtines & J. L. Gewirtz (Eds.), Handbook of moral behavior and development (pp.45–103). Lawrence Erlbaum Associates, Inc.
- Barling, J., & Beattie, R. (1983). Self-efficacy beliefs and sales performance. Journal of Organizational Behavior Management, 5(1), 41–51. https://doi.org/10.1300/J075v05n01_05
- Benight, C. C., & Bandura, A. (2004). Social cognitive theory of posttraumatic recovery: the role of perceived self-efficacy. Behaviour Research and Therapy, 42(10), 1129–1148. https://doi.org/10.1016/j.brat.2003.08.008
- Betz, N. E., & Hackett, G. (1981). The relationships of career-related self-efficacy expectations to perceived career options in college women and men. Journal of Counseling Psychology, 28(5), 399–410. https://psycnet.apa.org/doi/10.1037/0022-0167.28.5.399
- Bhalla, G., & Lin, L. (1987). Cross-cultural marketing research: a discussion of equivalence issues and measurement strategies. Psychology & Marketing, 4(4), 275–285.
- Bin, G. (2013). A reasoned action perspective of user innovation: model and empirical test. Industrial Marketing Management, 42(4), 608–619. https://doi.org/10.1016/j.indmarman.2012.10.001
- Bolt, M. A., Killough, L. N., & Koh, H. C. (2001). Testing the interaction effects of task complexity in computer training using the social cognitive model. Decision Sciences, 32(1), 1–20. https://doi.org/10.1111/j.1540-5915.2001.tb00951.x
- Burns, T., & Stalker, G. M. (1961). The management of innovation. Tavistock.
- Carrara, W., Chan, W. S., Fischer, S., & Steenbergen, E. v. (2015). Creating value through open data. European Commission. https://doi.org/10.2759/328101 (https://www.europeandataportal.eu/sites/default/files/edp_creating_value_through_open_data_0.pdf - archived by WebCite® at http://www.webcitation.org/75r7wnZvv)
- Compeau, D. R., & Higgins, C. A. (1995). Computer self-efficacy: development of a measure and initial test. MIS Quarterly, 19(2), 189–211. https://doi.org/10.2307/249688
- Compeau, D. R., Higgins, C. A., & Huff, S. (1999). Social cognitive theory and individual reactions to computing technology: a longitudinal study. MIS Quarterly, 23(2), 145–158. https://doi.org/10.2307/249749
- Cooper, C., & Lu, L. (2016). Presenteeism as a global phenomenon: unraveling the psychosocial mechanisms from the perspective of social cognitive theory. Cross Cultural & Strategic Management, 23(2), 216–231. https://doi.org/10.1108/CCSM-09-2015-0106
- Davis, F. D. (1989). Perceived usefulness, perceived ease of use, and user acceptance of information technology. MIS Quarterly, 13(3), 319–340. https://doi.org/10.2307/249008
- de Jong, J. P. J., & von Hippel, E. (2009). Transfers of user process innovations to process equipment producers: a study of Dutch high-tech firms. Research Policy, 38(7), 1181–1191. https://doi.org/10.1016/j.respol.2009.04.005
- Dholakia, R. R., & Uusitalo, O. (2002). Switching to electronic stores: consumer characteristics and the perception of shopping benefits. International Journal of Retail and Distribution Management, 30(10), 459–469. https://doi.org/10.1108/09590550210445335
- Dholakia, U. M., & Bagozzi, R. P. (2001). Consumer behavior in digital environments. In V. M. J. Wind (Ed.) Digital marketing: global strategies from the world's leading experts (pp. 163–200). Wiley.
- Dillon, T. W., & Lending, D. (2010). Will they adopt? Effects of privacy and accuracy. Journal of Computer Information Systems, 50(4), 20–29. https://doi.org/10.1080/08874417.2010.11645427
- Dillon, T. W., Lending, D., Crews, T., & Blankenship, R. (2003). Nursing self-efficacy of an integrated clinical and administrative information system. Computers Informatics Nursing, 21(4), 198–205.
- Dishaw, M. T., & Strong, D. M. (1998). Experience as a moderating variable in a task-technology fit model. Proceedings of the Americas Conference on Information Systems (AMCIS). (paper 242). Association for Information Systems. http://aisel.aisnet.org/amcis1998/242?utm_source=aisel.aisnet.org%2Famcis1998%2F242&utm_medium=PDF&utm_campaign=PDFCoverPages
- Dishaw, M. T., & Strong, D. M. (1999). Extending the technology acceptance model with task-technology fit constructs. Information & Management, 36(1), 9–21. https://doi.org/10.1016/S0378-7206(98)00101-3
- Durndell, A., & Haag, Z. (2002). Computer self efficacy, computer anxiety, attitudes towards the internet and reported experience with the internet, by gender, in an East European sample. Computers in Human Behavior, 18(5), 521–535. https://doi.org/10.1016/S0747-5632(02)00006-7
- Fishbein, M., & Ajzen, I. (1975). Belief, attitude, intention and behavior: an introduction to theory and research. Addison-Wesley.
- Flowers, S., von Hippel, E., Jong, J. d., & Sinozic, T. (2010). Measuring user innovation in the UK: the importance of product creation by users. Nesta. https://media.nesta.org.uk/documents/measuring_user_innovation _in_the_uk.pdf (Archived by WebCite® at http://www.webcitation.org/75r9MABwb)
- Font, X., Garay, L., & Jones, S. (2016). A social cognitive theory of sustainability empathy. Annals of Tourism Research, 58(1), 65–80. https://doi.org/10.1016/j.annals.2016.02.004
- Fornell, C., & Larcker, D. F. (1981). Evaluating structural equation models with unobservable variables and measurement error. Journal of Marketing Research, 18(1), 39–50. https://doi.org/10.1177%2F002224378101800104
- Franke, N., von Hippel, E., & Schreier, M. (2006). Finding commercially attractive user innovations: a test of lead-user theory. Journal of Product Innovation Management, 23(4), 301–315. https://doi.org/10.1111/j.1540-5885.2006.00203.x
- Freeman, C. (1968). Chemical process plant: innovation and the world market. National Institute Economic Review, (No. 45), 29–57.
- Gibbs, J. L., & Kramer, K. L. (2004). A cross-country investigation of the determinants of scope of e-commerce use: an institutional approach. Electronic Markets, 14(2), 124–137. https://doi.org/10.1080/10196780410001675077
- Hair, J. F., Black, B., Babin, B., Anderson, R. E., & Tatham, R. L. (2010). Multivariate data analysis. (7th ed.). Prentice-Hall.
- Hair, J. F., Black, W. C., Babin, B. J., & Anderson, R. E. (2009). Multivariate data analysis. (5th ed.). Prentice Hall.
- Hammell, R., Lewis, H., Perricos, C., & Branch, D. (2012). Open growth: stimulating demand for open data in the UK. Deloitte. https://www2.deloitte.com/content/dam/Deloitte/uk/Documents/deloitte-analytics/open-growth.pdf (Archived by WebCite® at http://www.webcitation.org/75r8yJmqb)
- Hienerth, C. (2006). The commercialization of user innovations: the development of the rodeo kayak industry. R&D Management, 36(3), 273–294. https://doi.org/10.1111/j.1467-9310.2006.00430.x
- Hill, T., Smith, N. D., & Mann, M. F. (1987). Role of efficacy expectations in predicting the decision to use advanced technologies: the case of computers. Journal of Applied Psychology, 72(2), 307–313. https://psycnet.apa.org/doi/10.1037/0021-9010.72.2.307
- Howard, R., Restrepo, L., & Chang, C.-Y. (2017). Addressing individual perceptions: an application of the unified theory of acceptance and use of technology to building information modelling. International Journal of Project Management, 35(2), 107–120. https://doi.org/10.1016/j.ijproman.2016.10.012
- Hsu, M.-H., Ju, T. L., Yen, C.-H., & Chang, C.-M. (2007). Knowledge sharing behavior in virtual communities: the relationship between trust, self-efficacy, and outcome expectations. International Journal of Human-Computer Studies, 65(2), 153–169. https://doi.org/10.1016/j.ijhcs.2006.09.003
- Huijboom, N., & Van den Broek, T. (2011). Open data: an international comparison of strategies. European Journal of ePractice, (No. 12), 1–13. https://pdfs.semanticscholar.org/ba0c/1a38276957f3b093dbec87e3543bcb8d3faf.pdf#page=4
- Hwang, Y. (2010). The moderating effects of gender on e-commerce systems adoption factors: an empirical investigation. Computers in Human Behavior, 26(6), 1753–1760. https://doi.org/10.1016/j.chb.2010.07.002
- Hyysalo, S. (2009). User innovation and everyday practices: micro-innovation in sports industry development. R&D Management, 39(3), 247–258. https://doi.org/10.1111/j.1467-9310.2009.00558.x
- Ifinedo, P. (2016). Applying uses and gratifications theory and social influence processes to understand students' pervasive adoption of social networking sites: perspectives from the Americas. International Journal of Information Management, 36(2), 192–206. https://doi.org/10.1016/j.ijinfomgt.2015.11.007
- Jöreskog, K. G., & Sörbom, D. (1993). Lisrel 8: Structural equation modeling with the simplis command language. Scientific Software International.
- Janssen, M., Charalabidis, Y., & Zuiderwijk, A. (2012). Benefits, adoption barriers and myths of open data and open government. Information Systems Management, 29(4), 258–268. https://doi.org/10.1080/10580530.2012.716740
- Janssen, M., & Zuiderwijk, A. (2014). Infomediary business models for connecting open data providers and users. Social Science Computer Review, 32(5), 694–711. https://doi.org/10.1177%2F0894439314525902
- Jetzek, T., Avital, M., & Bjørn-Andersen, N. (2013). Generating value from open government data. In Proceedings of the Thirty Fourth International Conference on Information Systems, Milan, Italy. Association for Information Systems.
- Jetzek, T., Avital, M., & Bjorn-Andersen, N. (2012). The value of open government data: a strategic analysis framework. Paper presented at the SIG eGovernment pre-ICIS Workshop, Orlando, Florida. https://bit.ly/32eZP9P
- Jetzek, T., Avital, M., & Bjorn-Andersen, N. (2014). Data-driven innovation through open government data. Journal of Theoretical and Applied Electronic Commerce Research, 9(2), 100–120. http://dx.doi.org/10.4067/S0718-18762014000200008
- Jones, G. R. (1986). Socialization tactics, self-efficacy, and newcomers' adjustments to organizations. Academy of Management Journal, 29(2), 262–279. https://doi.org/10.5465/256188
- Kalampokis, E., Tambouris, E., & Tarabanis, K. (2011). A classification scheme for open government data: towards linking decentralised data. International Journal of Web Engineering and Technology, 6(3), 266–285. http://citeseerx.ist.psu.edu/viewdoc/download?doi=10.1.1.703.4909&rep=rep1&type=pdf
- Kurnia, S., Karnali, R. J., & Rahim, M. M. (2015). A qualitative study of business-to-business electronic commerce adoption within the Indonesian grocery industry: a multi-theory perspective. Information & Management, 52(4), 518–536. https://doi.org/10.1016/j.im.2015.03.003
- Kwak, H., Fox, R. J., & Zinkhan, G. M. (2002). What products can be successfully promoted and sold via the internet? Journal of Advertising Research, 42(1), 23–38.
- LaRose, R., & Eastin, M. S. (2004). A social cognitive theory of internet uses and gratifications: toward a new model of media attendance. Journal of Broadcasting & Electronic Media, 48(3), 358–377.
- Liébana-Cabanillas, F., Sánchez-Fernández, J., & Muñoz-Leiva, F. (2014). The moderating effect of experience in the adoption of mobile payment tools in virtual social networks: the m-payment acceptance model in virtual social networks (MPAM-VSN). International Journal of Information Management, 34(2), 151–166. https://doi.org/10.1016/j.ijinfomgt.2013.12.006
- Lian, J.-W., Yen, D. C., & Wang, Y.-T. (2014). An exploratory study to understand the critical factors affecting the decision to adopt cloud computing in Taiwan hospital. International Journal of Information Management, 34(1), 2836. https://doi.org/10.1016/j.ijinfomgt.2013.09.004
- Liao, Z., & Cheung, M. T. (2001). Internet based e-shopping and consumer attitudes: an empirical study. Information & Management, 38(5), 299–306. https://doi.org/10.1016/S0378-7206(00)00072-0
- Lin, C. Y., & Ho, Y. H. (2009). An empirical study on the adoption of RFID technology for logistics service providers in China. International Business Research, 2(1), 23–36. http://citeseerx.ist.psu.edu/viewdoc/download?doi=10.1.1.668.2091&rep=rep1&type=pdf#page=24
- Liu, Y., Li, H., Kostakos, V., Goncalves, J., Hosio, S., & Hu, F. (2014). An empirical investigation of mobile government adoption in rural China: a case study in Zhejiang province. Government Information Quarterly, 31(3), 432–442. https://doi.org/10.1016/j.giq.2014.02.008
- Loo, W. H., Paul, H. P., Yeow, P. H. P., & Chong, S. C. (2009). User acceptance of Malaysian government multipurpose smartcard applications. Government Information Quarterly, 26(2), 358–367. https://doi.org/10.1016/j.giq.2008.07.004
- Lüthje, C. (2004). Characteristics of innovating users in a consumer goods field: an empirical study of sport-related product consumers. Technovation, 24(9), 683–695. https://doi.org/10.1016/S0166-4972(02)00150-5
- Lüthje, C., Herstatt, C., & von Hippel, E. (2005). User-innovators and "local" information: the case of mountain biking. Research Policy, 34(6), 951–965. https://doi.org/10.1016/j.respol.2005.05.005
- Marakas, G. M., Yi, M. Y., & Johnson, R. D. (1998). The multilevel and multifaceted character of computer self-efficacy: toward clarification of the construct and an integrative framework for research. Information Systems Research, 9(2), 126–163. https://doi.org/10.1287/isre.9.2.126
- Meijer, A., & Thaens, M. (2009). Public information strategies: making government information available to citizens. Information Polity, 14(1-2), 31–45. https://doi.org/10.3233/IP-2009-0167
- Miyazaki, A. D., & Fernandez, A. (2001). Consumer perceptions of privacy and security risks for online shopping. The Journal of Consumer Affairs, 35(1), 27–44. https://doi.org/10.1111/j.1745-6606.2001.tb00101.x
- Morrison, P. D., Roberts, J. H., & von Hippel, E. (2000). Determinants of user innovation and innovation sharing in a local market. Management Science, 46(12), 1513–1527. https://doi.org/10.1287/mnsc.46.12.1513.12076
- Myers, S., & Marquis, D. (1969). Successful industrial innovations. National Science Foundation.
- Nambisan, S., Agarwal, R., & Tanniru, M. (1999). Organizational mechanisms for enhancing user innovation in information technology. MIS Quarterly, 23(3), 365–395. https://doi.org/10.2307/249468
- Narayan, B. (2013). Social media use and civil society: from everyday information behaviours to clickable solidarity. Cosmopolitan Civil Societies, 5(3), 32–53. https://doi.org/10.5130/ccs.v5i3.3488
- O'Cass, A., & Fenech, T. (2003). Web retailing adoption: exploring the nature of internet users web retailing behaviour. Journal of Retailing and Consumer services, 10(2), 81–94. https://doi.org/10.1016/S0969-6989(02)00004-8
- Podsakoff, P. M., MacKenzie, S. B., Lee, J.-Y., & Podsakoff, N. P. (2003). Common method biases in behavioral research: a critical review of the literature and recommended remedies. Journal of Applied Psychology, 88(5), 879–903. https://psycnet.apa.org/doi/10.1037/0021-9010.88.5.879
- Prussia, G. E., & Kinicki, A. J. (1996). A motivational investigation of group effectiveness using social-cognitive theory. Journal of Applied Psychology, 81(2), 187–198. https://psycnet.apa.org/doi/10.1037/0021-9010.81.2.187
- Rahman, M. S., Ko, M., Warren, J., & Carpenter, D. (2016). Healthcare technology self-efficacy (HTSE) and its influence on individual attitude: an empirical study. Computers in Human Behavior, 58, 12–24. https://doi.org/10.1016/j.chb.2015.12.016
- Rana, N. P., & Dwivedi, Y. K. (2015). Citizen's adoption of an e-government system: validating extended social cognitive theory (SCT). Government Information Quarterly, 32(2), 172–181. https://doi.org/10.1016/j.giq.2015.02.002
- Ratten, V., & Ratten, H. (2007). Social cognitive theory in technological innovations. European Journal of Innovation Management, 10(1), 90–108. https://doi.org/10.1108/14601060710720564
- Reisinger, Y., & Turner, L. (1999). Structural equation modeling with Lisrel: application in tourism. Tourism Management, 20(1), 71–88. https://doi.org/10.1016/S0261-5177(98)00104-6
- Rogers, E. M. (1995). Diffusion of innovations. (4th ed.). Free Press.
- Rothwell, R. (1977). The characteristics of successful innovators and technically progressive firms. R&D Management, 3, 191–206. https://doi.org/10.1111/j.1467-9310.1977.tb01334.x
- Sahu, G. P., & Gupta, M. P. (2007). Users' acceptance of e-government: a study of Indian central excise. International Journal of Electronic Government Research, 3(3), 1–21. https://doi.org/10.4018/jegr.2007070101
- Sam, H. K., Othman, A. E. A., & Nordin, Z. S. (2005). Computer self-efficacy, computer anxiety, and attitudes toward the internet: a study among undergraduates in Unimas. Educational Technology & Society, 8(4), 205–219. https://www.jstor.org/stable/jeductechsoci.8.4.205
- Smith, M., & Brynjolfson, E. (2001). Consumer decision making at an internet shopbot: brand still matters. The Journal of Industrial Economics, 49(4), 541–558. https://doi.org/10.1111/1467-6451.00162
- Song, J. (2014). Understanding the adoption of mobile innovation in China. Computers in Human Behavior, 38, 339–348. https://doi.org/10.1016/j.chb.2014.06.016
- Sun, J. (2016). Tool choice in innovation diffusion: a human activity readiness theory. Computers in Human Behavior, 59, 283–294. https://doi.org/10.1016/j.chb.2016.02.014
- Susha, I., Grönlund, Å., & Janssen, M. (2015). Driving factors of service innovation using open government data: an exploratory study of entrepreneurs in two countries. Information Polity, 20(1), 19–34. https://doi.org/10.3233/IP-150353
- Tan, Z. X., & Ouyang, W. (2006). China: overcoming institutional barriers to e-commerce. Cambridge University Press.
- Teo, T. S. H., & Ranganathan, C. (2004). Adopters and non-adopters of business-to-business electronic commerce in Singapore. Information & Management, 42(1), 89–102. https://doi.org/10.1016/j.im.2003.12.005
- Teoh, W. M.-Y., Chong, S. C., Lin, B., & Chua, J. W. (2013). Factors affecting consumers' perception of electronic payment: an empirical analysis. Internet Research, 23(4), 465–485. https://doi.org/10.1016/j.im.2003.12.005
- Thatcher, J. B., & Perrewe, P. L. (2002). An empirical examination of individual traits as antecedents to computer anxiety and computer self-efficacy. MIS Quarterly, 26(4), 381–396. https://doi.org/10.2307/4132314
- Venkatesh, V. (2000). Determinants of perceived ease of use: integrating control, intrinsic motivation, and emotion into the technology acceptance model. Information Systems Research, 11(4), 342–365. https://doi.org/10.1287/isre.11.4.342.11872
- Venkatesh, V., & Davis, F. D. (1996). A model of the antecedents of perceived ease of use: development and test. Decision Sciences, 27(3), 451–481. https://doi.org/10.1111/j.1540-5915.1996.tb00860.x
- Venkatesh, V., Morris, M. G., Davis, G. B., & Davis, F. D. (2003). User acceptance of information technology: toward a unified view. MIS Quarterly, 27(3), 425–478. https://doi.org/10.2307/30036540
- Vetrò, A., Canova, L., Torchiano, M., Minotas, C. O., Iemma, R., & Morando, F. (2016). Open data quality measurement framework: definition and application to open government data. Government Information Quarterly, 33(2), 325–337. https://doi.org/10.1016/j.giq.2016.02.001
- Virginia Employment Commission. (2016, July 7). Governor McAuliffe announces data analytics initiative to connect job seekers to jobs. https://www.vec.virginia.gov/node/3610 (Archived by WebCite® at http://www.webcitation.org/765KX9eyg)
- von Hippel, E. (1988). The sources of innovation. Oxford University Press.
- von Hippel, E. (2005). Democritizing innovation. MIT Press.
- Wang, H.-J., & Lo, J. (2016). Adoption of open government data among government agencies. Government Information Quarterly, 33(1), 80–88. https://doi.org/10.1016/j.giq.2015.11.004
- Warshaw, P. R. (1980). A new model for predicting behavioral intentions: an alternative to Fishbein. Journal of Marketing Research, 17(2), 153–172. https://doi.org/10.1177%2F002224378001700201
- White, G. L., Shah, J. R., Cook, J. R., & Mendez, F. (2008). Relationship between information privacy concerns and computer self-efficacy. International Journal of Technology and Human Interaction, 4(2), 52–82. https://doi.org/10.4018/jthi.2008040104
- Wood, R., & Bandura, A. (1989). Social cognitive theory of organizational management. Academy of Management Review, 14(3), 361–384. https://doi.org/10.5465/amr.1989.4279067
- Youn, S. (2009). Determinants of online privacy concern and its influence on privacy protection behaviors among young adolescents. Journal of Consumer Affairs, 43(3), 389–418. https://doi.org/10.1111/j.1745-6606.2009.01146.x
- Zuiderwijk, A., Janssen, M., Choenni, S., Meijer, R., & Alibaks, R. S. (2012). Socio-technical impediments of open data. Electronic Journal of e-Government, 10(2), 156–172. http://www.ejeg.com/issue/download.html?idArticle=255 (Archived by the Internet Archive at https://web.archive.org/web/20190803195319/http://www.ejeg.com/issue/download.html?idArticle=255)
- Zuiderwijk, A., Janssen, M. & Dwivedi, Y. K. (2015). Acceptance and use predictors of open data technologies: drawing upon the unified theory of acceptance and use of technology. Government Information Quarterly, 32(4),429–440. https://doi.org/10.1016/j.giq.2015.09.005